Unleashing the Potential of Knowledge Graphs with Elasticsearch
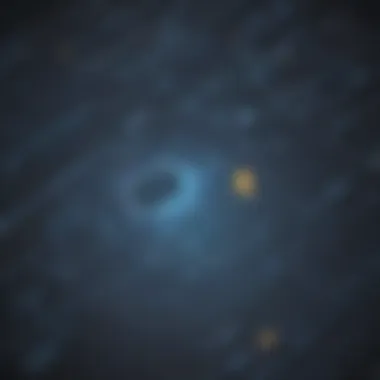
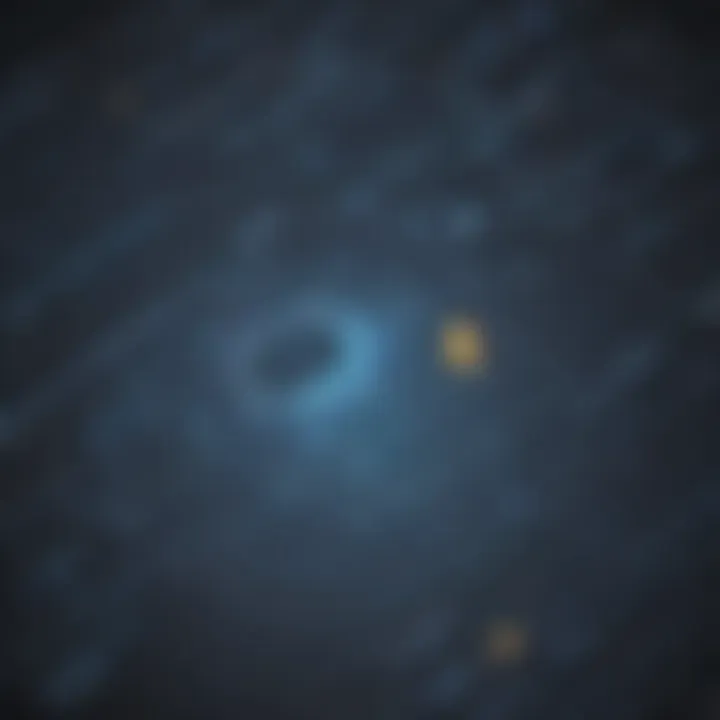
Software Overview
When it comes to exploring the powerful synergy between knowledge graphs and Elasticsearch, delving into the software overview is crucial. Elasticsearch is renowned for its robust features and functionalities that cater to data discovery, search, and analysis. Its high-performance capabilities make it a preferred choice for both small and large businesses looking to extract meaningful insights from their data. Additionally, Elasticsearch offers flexible pricing and licensing options, ensuring that organizations can scale their usage based on their specific requirements. This compatibility extends its usability across a wide range of platforms, enhancing its accessibility and integration possibilities.
User Experience
In terms of user experience, Elasticsearch shines with its intuitive interface design and ease of use. Professionals in the IT and software domains appreciate the simplicity and effectiveness of Elasticsearch's user settings, allowing for seamless customization based on individual preferences. Moreover, Elasticsearch's performance and speed capabilities set it apart, providing swift and accurate results for data retrieval and analysis tasks.
Pros and Cons
Undoubtedly, Elasticsearch boasts numerous strengths and advantages, such as its scalability, real-time data processing, and extensive search functionality. However, like any software, it also has its limitations, including potential complexity in setup for novice users and the need for continuous optimization to maintain peak performance. A comparison with similar products further illuminates Elasticsearch's unique selling points and areas for potential enhancement.
Real-world Applications
In real-world scenarios, Elasticsearch finds diverse industry-specific uses, from e-commerce and healthcare to finance and cybersecurity. Through compelling case studies and success stories, organizations can gain insights into how Elasticsearch effectively addresses specific challenges, offering tailored solutions that drive efficiency and innovation. By highlighting its problem-solving capabilities, Elasticsearch emerges as a versatile tool with widespread applicability.
Updates and Support
Keeping abreast of software updates is essential in the rapidly evolving tech landscape, and Elasticsearch ensures regular updates to enhance functionality and address user feedback. Customers benefit from a range of support options, including dedicated customer service channels and active community forums that foster peer-to-peer learning and knowledge sharing. This robust support ecosystem empowers users to maximize the potential of Elasticsearch and stay informed about the latest developments in the field.
Introduction to Knowledge Graphs
In the domain of data management, the discourse surrounding knowledge graphs is crucial. Understanding knowledge graphs involves grasping the interconnected nature of data and the potential they hold for enhancing information retrieval. Knowledge graphs serve as powerful tools that organize data into a structured format, enabling efficient analysis and exploration. The essence of knowledge graphs lies in their ability to connect disparate data points, bridging relationships and offering a holistic view of information. This article sheds light on the implications of integrating knowledge graphs within Elasticsearch, paving the way for improved data discovery and search functionalities.
Understanding Knowledge Graphs
Why Knowledge Graphs are Essential in Data Management
The significance of knowledge graphs in data management cannot be understated. These structured representations of knowledge play a fundamental role in deciphering complex relationships within data sets. By contextualizing information and establishing connections between entities, knowledge graphs facilitate seamless data exploration and interpretation. Their innate ability to capture not just data but the relationships between data points empowers organizations to extract valuable insights from vast troves of information. Incorporating knowledge graphs in data management strategies not only enhances data organization but also streamlines the process of deriving meaningful conclusions from data.
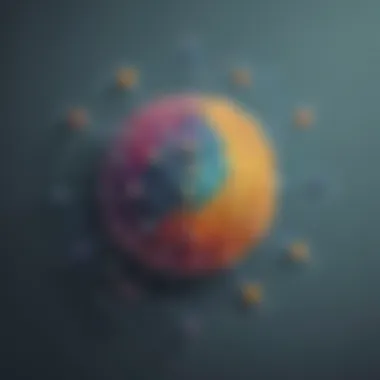
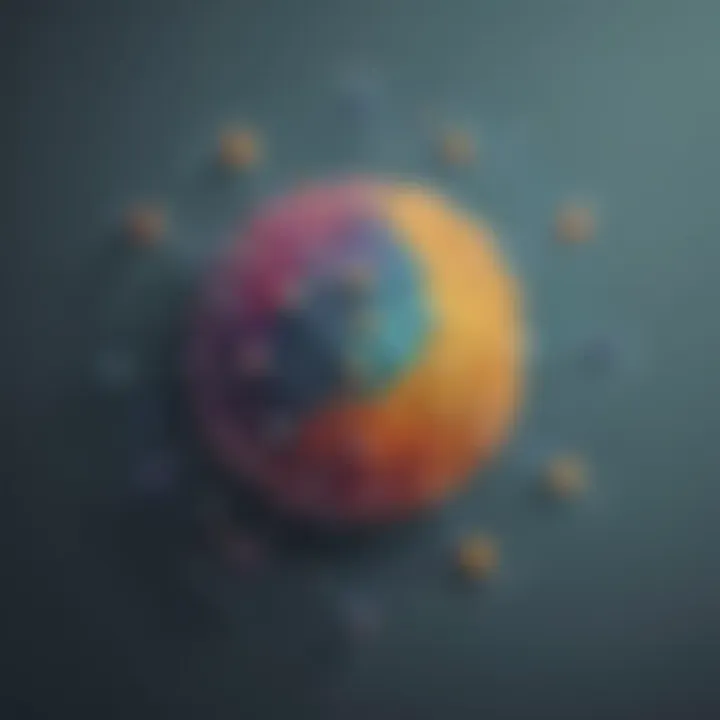
Key Components of a Knowledge Graph
Within the realm of knowledge graphs, several key components form the foundational pillars of their functionality. Nodes, which represent entities, and edges, which depict relationships between entities, constitute the basic building blocks of a knowledge graph. Properties attached to nodes and edges provide additional context, enriching the depth of information encoded within the graph structure. The hierarchical structure of knowledge graphs enables the modeling of complex relationships, offering a comprehensive perspective on interconnected data points. Leveraging these components optimizes data querying, information retrieval, and fosters a deeper understanding of the underlying information landscape.
Elasticsearch: An Overview
Introduction to Elasticsearch
Scalability and Performance of Elasticsearch
The scalability and performance of Elasticsearch play a pivotal role in the efficacy of this technology within the context of knowledge graphs. Elasticsearch's ability to scale seamlessly with growing amounts of data ensures that businesses can efficiently manage and analyze large datasets. Additionally, its exceptional performance in terms of speed and reliability empowers users to execute complex queries and obtain real-time insights promptly. The elastic nature of Elasticsearch accommodates the dynamic nature of knowledge graphs, allowing for fluid and efficient operations in data retrieval and analysis. While its scalability facilitates the handling of immense data volumes, its performance ensures quick and accurate results, making it a preferred choice for businesses seeking a robust data management solution.
Features and Capabilities
Exploring the features and capabilities of Elasticsearch reveals a diverse set of tools and functionalities that amplify its utility in conjunction with knowledge graphs. The unique features such as full-text search, real-time analytics, and horizontal scalability contribute significantly to its effectiveness in data processing and analysis. The capabilities of Elasticsearch encompass versatile search options, advanced analytics, and seamless integration with various data sources, making it a comprehensive tool for information retrieval and exploration. However, despite its numerous advantages, Elasticsearch does present certain disadvantages, including complex setup processes and potential performance bottlenecks under specific conditions. Understanding these features and capabilities is essential for leveraging Elasticsearch optimally within the realm of knowledge graphs.
The Integration of Knowledge Graphs and Elasticsearch
In this section, we delve into the critical juncture where Knowledge Graphs and Elasticsearch converge, unraveling the intricate tapestry of their integration. The fusion of these technologies holds immense significance in augmenting data discovery, search efficiency, and analytical capabilities. By combining the structured and connected data representation of Knowledge Graphs with Elasticsearch's robust search and indexing functionalities, organizations can unlock a new realm of possibilities in information retrieval and exploration. This section will expound on the specific elements, benefits, and considerations inherent in the seamless integration of Knowledge Graphs and Elasticsearch.
Creating a Cohesive Ecosystem
Utilizing Elasticsearch for Knowledge Graph Querying
Delving into the realm of utilizing Elasticsearch for Knowledge Graph querying unveils a pivotal aspect of this integration. Elasticsearch's prowess in handling complex search queries at scale complements the intricate relationship mappings within Knowledge Graphs, facilitating efficient and accurate data retrieval. The key characteristic that sets Elasticsearch apart in this scenario is its ability to swiftly traverse vast datasets while maintaining high performance levels, making it a preferred choice for enabling Knowledge Graph-based queries in this article. The unique feature of Elasticsearch lies in its near real-time search capabilities, ensuring that as new data is added to the Knowledge Graph, it is readily accessible through Elasticsearch queries. Though overwhelmingly beneficial, one must consider certain nuances, such as data consistency and indexing overheads, when leveraging Elasticsearch for Knowledge Graph querying within this integrated ecosystem.
Enhancing Search Relevance with Knowledge Graph Insights
Another focal point within this integrated landscape is the enhancement of search relevance through Knowledge Graph insights. By leveraging the semantic relationships encoded in the Knowledge Graph, Elasticsearch can deliver more contextually relevant search results to users. This synergy empowers organizations to extract deeper insights from their data repositories, improving decision-making processes and user experiences. The key characteristic of this enhancement is the ability to surface interconnected information based on semantic meanings rather than keyword matching alone, elevating the overall search relevance and user satisfaction. The unique feature here lies in the dynamic adaptability of search results based on evolving relationships in the Knowledge Graph, providing a more personalized and insightful search experience. Despite its evident advantages, considerations need to be given to optimizing relevance weighting and query performance to ensure a seamless integration of Knowledge Graph insights into Elasticsearch search results in this article.
Benefits of Combining Knowledge Graphs with Elasticsearch
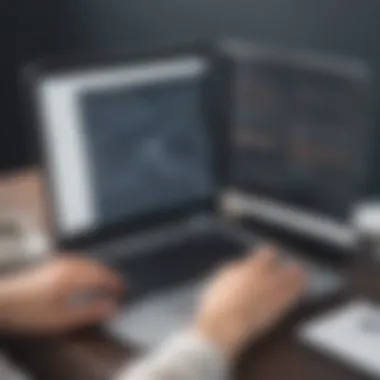
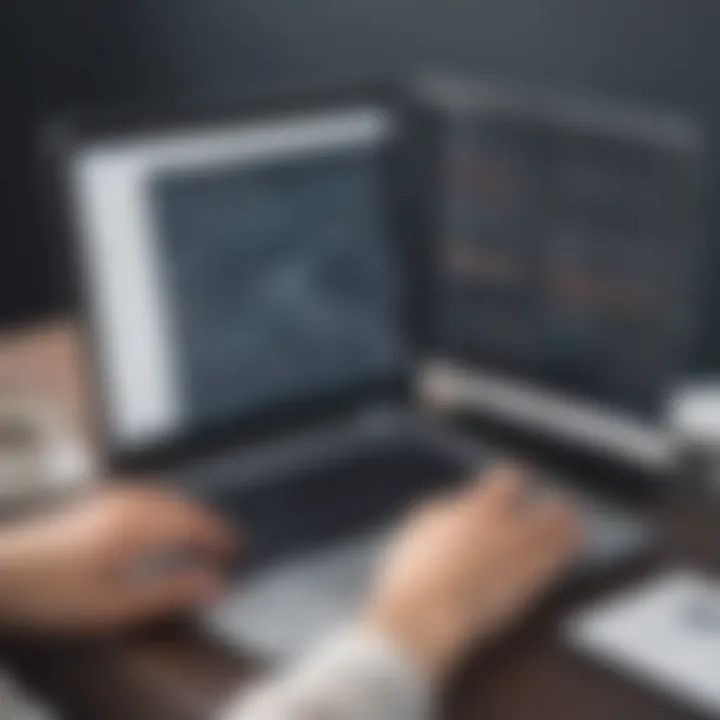
In this article, the crucial focus is on elucidating the advantages of merging knowledge graphs with Elasticsearch to enhance data discovery, search, and analysis capabilities. By integrating knowledge graphs into Elasticsearch, a more refined and insightful approach to information retrieval and exploration is achieved. This amalgamation opens up a realm of possibilities for improving the efficiency and effectiveness of handling vast amounts of data. Leveraging the strengths of both technologies leads to a synergistic relationship that amplifies the overall performance and usability of Elasticsearch in various industries and applications.
Improved Data Discovery
Enhanced Semantic Search Capabilities
The prowess of enhanced semantic search capabilities lies in its ability to decipher the contextual meaning behind search queries. By incorporating semantic elements into search processes, the system can understand the intent behind user queries beyond keyword matching. This advanced search functionality empowers users to refine their search criteria, leading to more accurate and personalized results. The distinctive feature of enhanced semantic search is its capacity to interpret complex relationships between data points, offering a profound understanding of the connections within the information landscape. While enhancing search precision, this capability also reduces irrelevant search results, streamlining the data discovery process.
Increased Contextual Understanding
Increased contextual understanding plays a pivotal role in enriching the overall data exploration experience. Through contextual insights, users can grasp the significance and relevance of data within a given context. This feature not only provides a deeper understanding of the data but also facilitates more informed decision-making processes. The key characteristic of increased contextual understanding is its ability to present information in a meaningful context, enabling users to extract valuable insights effortlessly. However, it is essential to note that while increased contextual understanding enhances data comprehension, it may also introduce complexities in managing and interpreting contextual nuances within large datasets.
Use Cases and Applications
Knowledge Graph Integration in Industries
Healthcare
In the realm of healthcare, the integration of knowledge graphs with Elasticsearch plays a pivotal role in revolutionizing patient care, medical research, and health management systems. The key characteristic that sets healthcare apart in this context is the complex network of medical data, patient records, and treatment protocols that can be efficiently indexed and queried through Elasticsearch-powered knowledge graphs. This synergy enables healthcare providers to unlock insights into patient histories, treatment outcomes, and disease patterns with unprecedented speed and accuracy, ultimately leading to enhanced clinical decision-making and personalized healthcare solutions.
Finance
Within the finance sector, leveraging knowledge graphs with Elasticsearch offers a strategic advantage in risk management, fraud detection, and financial analysis. The distinctive feature of finance lies in its vast interconnected data landscape comprising market trends, customer transactions, and regulatory frameworks that can be effectively navigated and analyzed using Elasticsearch functionalities. By harnessing the power of knowledge graphs, financial institutions can gain a comprehensive view of market dynamics, assess transactional risks, and optimize investment strategies with a heightened level of precision and timeliness.
Retail
In the retail industry, the fusion of knowledge graphs with Elasticsearch drives advancements in customer analytics, inventory management, and demand forecasting. Retail's key characteristic in this integration is the abundance of consumer data points, product catalogs, and sales trends that can be seamlessly integrated and queried through Elasticsearch-driven knowledge graphs. This dynamic partnership empowers retailers to decode shopper preferences, streamline supply chain operations, and tailor marketing campaigns based on granular insights extracted from interconnected data sets, thus enhancing customer experiences and optimizing business performance.
Challenges and Considerations
In the intricate landscape of knowledge graphs integrated with Elasticsearch, understanding the challenges and considerations is paramount. This section delves into the essential factors crucial for optimizing the combined potential of these technologies. By addressing the hurdles and strategic elements involved, businesses can effectively harness the power of knowledge graphs in Elasticsearch to drive data discovery and analysis.
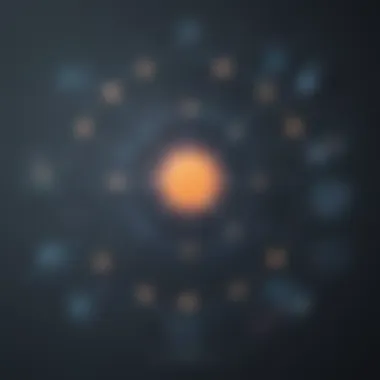
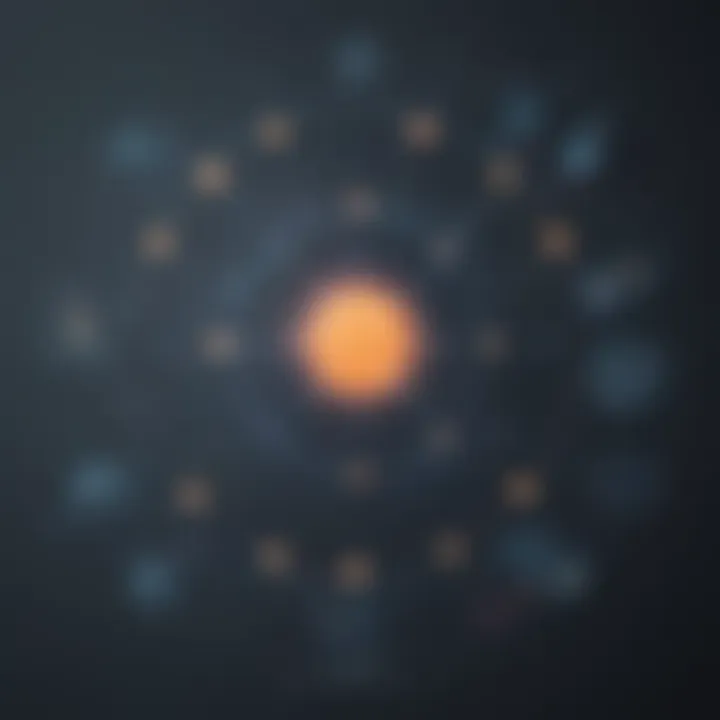
Addressing Scalability Issues
Handling Complex Relationships
Handling complex relationships within knowledge graphs and Elasticsearch is a critical aspect that significantly impacts data management and retrieval. This intricate process involves navigating interconnected data points, relationships, and hierarchies within a structured framework. One key characteristic of handling complex relationships is the ability to decipher intricate data connections to extract meaningful insights and establish correlations between disparate data sets. Adopting a sophisticated approach to managing complex relationships allows for a more nuanced understanding of data interdependencies, fostering enhanced analytical capabilities. Despite the complexities involved, the meticulous handling of intricate data relationships is a valuable strategy in optimizing knowledge graph utilization within Elasticsearch, offering a deeper level of data exploration and analysis.
Data Quality and Integration
The aspect of data quality and integration plays a pivotal role in ensuring the efficacy and reliability of knowledge graphs integrated with Elasticsearch. Maintaining high data quality standards is essential for accurate information retrieval and analysis within the combined system. Robust data integration processes are imperative for seamlessly harmonizing diverse data sources and formats, consolidating information for comprehensive analysis. A key characteristic of effective data quality and integration lies in the ability to cleanse, transform, and integrate data seamlessly, ensuring coherence and consistency across the knowledge graph and Elasticsearch platforms. While advancing data quality and integration enhances the accuracy and reliability of insights derived from knowledge graphs, inefficiencies or inaccuracies in this aspect can lead to skewed results and misinterpretations. By prioritizing data quality and integration within the knowledge graph and Elasticsearch ecosystem, organizations can unlock the full potential of these technologies for driving informed decision-making and actionable insights.
Future Trends and Innovations
Future Trends and Innovations play a crucial role in shaping the landscape of knowledge graphs and Elasticsearch integration. This section explores the evolving trends and advancements that are influencing the utilization of these technologies in data management and analytics. By staying abreast of Future Trends and Innovations in the field, businesses can adapt their strategies to remain competitive and maximize the benefits offered by Knowledge Graphs and Elasticsearch.
Machine Learning Integration
AI-Powered Knowledge Graphs:
The integration of Artificial Intelligence (AI) technologies into Knowledge Graphs enhances the capabilities of data analysis and exploration. AI-Powered Knowledge Graphs leverage machine learning algorithms to derive complex insights from interconnected data points, enabling more accurate search results and predictive analytics. The ability of AI-Powered Knowledge Graphs to self-learn and continuously improve their understanding of data sets makes them a valuable asset in transforming information retrieval processes and decision-making.
Predictive Analytics:
Predictive Analytics empower organizations to forecast future trends, behaviors, and outcomes based on historical data patterns. By integrating Predictive Analytics into the realm of Knowledge Graphs and Elasticsearch, businesses can gain foresight into potential developments and possibilities within their operations. This proactive approach allows companies to anticipate changes, identify opportunities, and mitigate risks effectively. However, the reliance on historical data for predictions in Predictive Analytics may introduce biases and limitations that need to be carefully managed for optimal decision-making.
Conclusion
In concluding this detailed exploration of the power unleashed when knowledge graphs integrate with Elasticsearch, it is vital to emphasize the transformative impact of this synergy. The significance of this union lies in the sharpening of data discovery, search efficiency, and analytical capabilities. By effectively marrying the sophisticated structuring of knowledge graphs with the agile querying prowess of Elasticsearch, organizations can propel their information retrieval and exploration endeavors to unprecedented heights. This amalgamation not only enhances the speed at which insights are uncovered but also enriches the relevance and contextuality of search results, thereby fostering a deeper understanding of complex data landscapes.
Harnessing the Potential of Knowledge Graphs in Elasticsearch
Unlocking Data Insights
Unlocking Data Insights occupies a pivotal role in this narrative as it serves as the linchpin for extracting valuable nuggets of knowledge from vast data reservoirs. Its core contribution to the overarching theme of this discourse is the facilitation of precise and targeted information retrieval. By harnessing the innate capacity of Unlocking Data Insights to navigate through intricate data webs and unearth correlations, organizations can distill actionable insights with unprecedented clarity. The key characteristic underpinning Unlocking Data Insights is its ability to decipher complex relational patterns and present them in a digestible format for enhanced decision-making. A common choice for datasets brimming with interconnections, Unlocking Data Insights holds an upper hand in deciphering convoluted data structures but may present challenges in scenarios of data scarcity.
Driving Innovation in Information Retrieval
Driving Innovation in Information Retrieval emerges as a key driver for reshaping the conventional paradigms of data access and interpretation. Its primary function within the broader context of this exposition is to revolutionize the efficiency and efficacy of information search processes. The standout feature that distinguishes Driving Innovation in Information Retrieval is its propensity to incorporate advanced algorithms and contextual understanding, thereby enhancing the precision and relevance of search outcomes. A popular choice for organizations seeking to stay at the vanguard of information management trends, this approach enriches the user experience by delivering tailored and insightful search results. However, one must tread carefully as the reliance on predictive models for information retrieval may introduce elements of bias and predisposition which warrant careful consideration.