Understanding SPS Data Analytics for Enhanced Decision-Making
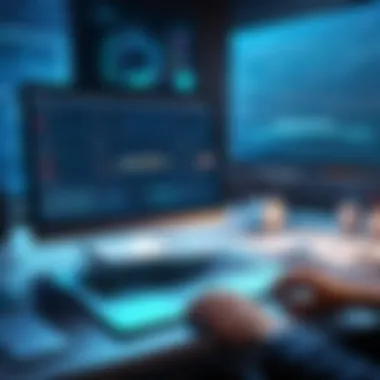
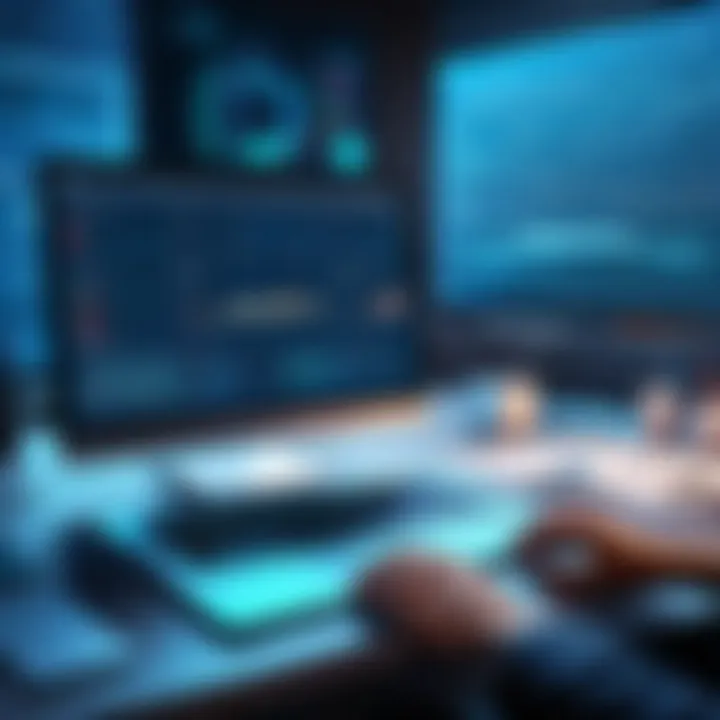
Intro
In the ever-evolving landscape of data analytics, the Statistical Package for the Social Sciences (SPSS) emerges as a pivotal tool utilized across various sectors. With its robust functionalities and user-friendly interface, SPSS empowers professionals to conduct thorough analysis, facilitating informed decision-making processes. This exploration seeks to unpack the layers of SPS data analytics, illuminating its methodologies, applications, and future directions.
Understanding data analytics in the context of SPSS is crucial, especially for businesses striving to elevate their strategic planning. As markets flourish with vast quantities of data, the need for effective analysis becomes more paramount than ever. Organizations that grasp the nuances of SPSS can leverage its capabilities to glean actionable insights, ultimately steering them toward enhanced operational efficacy.
In this article, we will take a closer look at several key areas within the SPS data analytics realm:
- Software Overview: A glimpse into the features, pricing, and compatibility of SPSS.
- User Experience: Insights on interface design and overall performance.
- Pros and Cons: A balanced examination of the strengths and limitations.
- Real-world Applications: Exploring industry-specific applications and tangible outcomes.
- Updates and Support: Information on software updates and user resources.
Through this detailed narrative, we aim to provide a comprehensive understanding of how SPSS data analytics can transform data into meaningful insights for both small and large enterprises.
Intro to SPS Data Analytics
SPS data analytics holds a pivotal role in todayâs rapidly evolving landscape of information technology and data management. Understanding this context is essential for those involved in IT and data-driven environments, whether it be professionals in established companies or startups that are still finding their footing. The principles and methodologies of SPS analytics can illuminate a path to improved decision-making, ultimately leading to enhanced operational efficiency and strategic insight.
In this article, we will tackle the core elements of SPS data analytics, reviewing its foundational principles, historical background, and significance in the modern world. \n
Definition and Overview
At its heart, SPS (Statistical Package for the Social Sciences) data analytics is a software application designed to manage and analyze data, particularly in social science research. It provides a suite of functionalitiesâfrom basic statistics to complex data manipulationsâthat serve a broad array of users. Notably, this software empowers researchers and businesses alike to make sense of vital data, turning raw numbers into actionable insights.
More than just a tool, SPS analytics creates a scaffold for accumulating knowledge. It hinges on the interplay between data and statistical rigor, aiding in uncovering patterns or revealing correlations that might otherwise slip beneath the radar. Its user-friendly interface allows novices and experts alike to engage with data without a steep learning curve, making it accessible while maintaining depth.
In essence, SPS data analytics helps answer critical questions, such as:
- How did a marketing campaign perform?
- What are the preferences of a specific demographic?
- Which factors contribute most significantly to patient recovery in healthcare?
These inquiries underscore the software's practicality and highlight its importance across disciplines.
Historical Development
The timeline of SPS analytics traces back to the 1960s when it was initially developed to analyze survey data. Over the decades, this simple tool has transformed significantly, evolving into a robust software suite utilized across multiple industries. From its humble beginnings focused on academic research, its scope broadened to encompass various sectorsâincluding healthcare, marketing, and business intelligence.
Key milestones in its evolution include the introduction of user-friendly graphical interfaces, the integration of advanced statistical techniques, and the ability to handle large datasets. As computing power increased, so did the capabilities of SPS, making it adaptable to the demands of modern data analysis.
The 1980s marked the introduction of SPSS for Windows, enhancing accessibility and usability. Subsequently, updates have fostered its growth into a sophisticated system capable of predictive analytics and machine learning applications.
Today, SPS is viewed not merely as a tool for statistics but as a vital component in the ongoing quest for data-driven strategies. As organizations seek to optimize performance and innovate continuously, an understanding of SPS data analytics becomes not just beneficial but essential.
Understanding SPSS
Understanding SPSS is pivotal for anyone serious about leveraging data analytics for insights. The Statistical Package for the Social Sciences (SPSS) stands as a robust tool that can transform raw data into actionable information. This section will explore what SPSS is, its key features, and how it stacks up against its competitors in the data analytics landscape.
What is SPSS?
SPSS is a software package used for statistical analysis, widely recognized among researchers and data analysts. Initially developed by Norman H. Nie, C. Hadlai Hull, and William G. Harder in 1968, it has evolved significantly. SPSS is particularly popular in social science research and business analytics, thanks to its user-friendly interface and extensive capabilities. Its ability to perform complex data manipulations and statistical analyses with ease makes it an invaluable asset in any analyst's toolbox.
The tool supports a variety of statistical procedures, including:
- Descriptive statistics
- T-tests
- ANOVA (Analysis of Variance)
- Regression analysis
- Factor analysis
By enabling users to visualize data patterns effectively, SPSS helps in making informed decisions based on empirical evidence rather than gut feelings.
Key Features of SPSS
SPSS comes packed with features that cater to various analytical needs. Some standout functionalities include:
- User-Friendly Interface: Even those without a technical background can navigate through SPSS efficiently, thanks to its intuitive design.
- Data Management Capabilities: Whether merging datasets or reshaping data, SPSS provides tools that facilitate seamless data management.
- Visualization Tools: SPSS equips users with graphs and charts to visualize results, enhancing understandability. This assists in communicating findings to stakeholders who may not have a technical background.
- Extensive Reports and Documentation: Generating comprehensive reports with relevant statistical outputs is straightforward. This is particularly useful for presentations and academic submissions.
The versatility of SPSS positions it as a leader in data analytics software, suitable for academic, commercial, and research settings.
Comparison with Other Data Analytics Tools
When contrasting SPSS with other data analytics tools, several factors come into play: usability, functionality, and cost-effectiveness.
- Usability: SPSS excels in its ease of use compared to more complex applications, such as R or Python, which require programming expertise.
- Functionality: While SPSS is particularly strong in statistical analysis, tools like Tableau offer superior data visualization capabilities. However, SPSS integrates multiple functionalities, minimizing the need to switch between applications.
- Cost: SPSS can be on the pricier side, especially for comprehensive licenses, making tools like OpenOffice Calc or R often preferred for those on a budget.
Overall, the choice of tool can depend on specific project needs, team expertise, and budget constraints. However, whether one is working on a complex research project or simple data analysis, understanding SPSS is essential for effective data-driven decision-making.
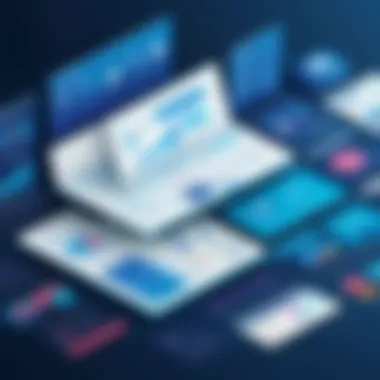
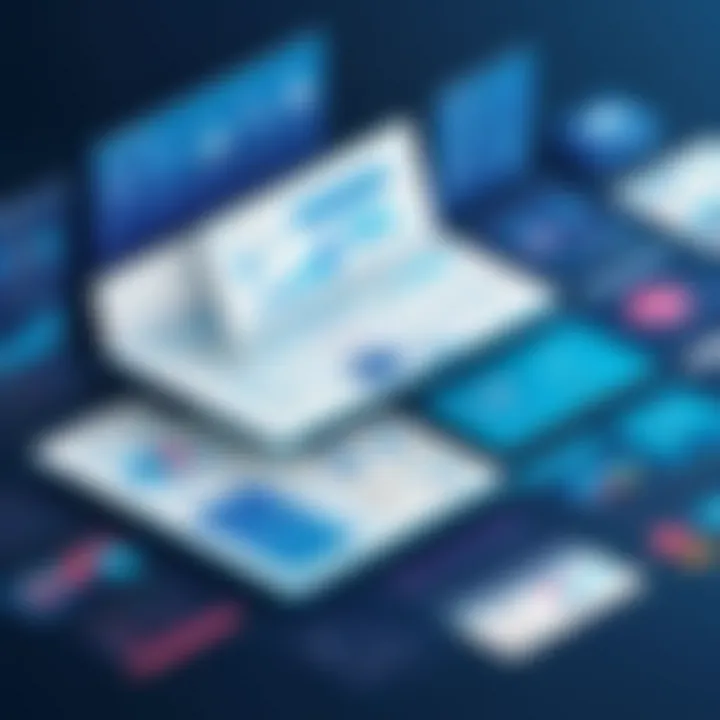
"Understanding SPSS and its capabilities is not just about using a software; itâs about transforming data into insights that drive actionable change."
By mastering SPSS, one positions themselves at the forefront of analytical prowess in their field.
Methodologies in SPS Data Analytics
Understanding the methodologies in SPS data analytics is vital for professionals and organizations looking to harness the power of data. These methodologies serve as the backbone for conducting thorough and reliable analyses that lead to actionable insights. Itâs not just about gathering data but how you interpret it and what steps you take to ensure accuracy and validity. By adopting robust methodologies, businesses can significantly improve decision-making processes and strategic planning.
Data Collection Techniques
Data collection is the first step in any analytics project, and it's where the foundation of valid results is laid. Without effective data collection techniques, even the most advanced analytical methods can fall flat.
- Surveys and Questionnaires: Surveys are common for gathering quantitative data. Crafting questions that are clear and unbiased is crucial. This ensures that responses reflect true sentiments and experiences. Online tools like Google Forms or SurveyMonkey are often used for this purpose.
- Interviews and Focus Groups: These are useful for qualitative insights. They help in understanding context and motivations behind the numbers. A semi-structured interview format can open dialogue and lead to unexpected insights.
- Observational Studies: Watching behavior in real time provides immediate context to data. This is especially important in marketing analytics where understanding customer behavior can lead to better-targeted campaigns.
- Using Existing Data: Often, organizations have a treasure trove of data lying around in old databases or reports. Leveraging existing data can save time and resources, but it's essential to ensure its relevance to the current analysis objectives.
Gathering the right data entails asking the right questions and choosing appropriate methods. This can boost the reliability of the findings.
Data Cleaning and Preparation
After collecting data, itâs imperative to clean and prepare it for analysis. Data, once collected, can be messy, fraught with inconsistencies or outliers. This stage is often regarded as the most labor-intensive but is critical to the integrity of the analysis.
Key steps include:
- Removing Duplicates: Duplicate entries can lead to skewed results. Identifying and eliminating these duplicates helps ensure that the analysis reflects true trends.
- Handling Missing Values: Missing data can be handled by techniques such as imputation or exclusion. Each has its pros and cons; the choice often depends on the data set and the analysis planned.
- Standardization: Converting data into a uniform format is key. This includes ensuring that all date formats are consistent or that categorical variables have the same naming conventions.
Proper data cleaning ensures that analytics can continue smoothly. Without it, analysts run the risk of basing decisions on flawed data, which can be a costly mistake.
Statistical Analysis Methods
The heart of SPS data analytics lies in its statistical analysis methods. Choosing the right analysis method can significantly affect the outcomes and subsequent recommendations. Some prevalent methods include:
- Descriptive Statistics: These methods provide summaries of the data and are often the first step. They allow analysts to understand what, where, and when certain trends or patterns happen.
- Inferential Statistics: This goes a step further by allowing analysts to make predictions or generalizations about a population based on a sample. Methods such as regression analysis can identify relationships between variables.
- Multivariate Analysis: This method examines more than two variables to understand relationships and impacts more deeply. Techniques like factor analysis and cluster analysis can reveal hidden patterns within datasets.
The chosen method must align with the analytics objectives. Each approach has its nuances and is appropriate for different circumstances, depending on the goals of the analysis.
"In the realm of SPS data analytics, the methods used are not just tools; they are the compass that guide decision-makers through the jungle of data."
Having a solid grasp of methodologies ensures not only accurate results but also drives informed decision-making that can be seamlessly integrated into broader organizational strategies.
Applications of SPS Data Analytics
The integration of SPS data analytics into diverse fields shines a light on its significance in making well-informed decisions across multiple domains. This section discusses how different sectors benefit from the capabilities provided by SPSS, focusing on practical applications that enhance organizational effectiveness.
SPS in Marketing
SPS data analytics plays a pivotal role in marketing strategies. This isn't just about putting numbers together; itâs about interpreting what those numbers say about customer behaviors and preferences. Companies use SPSS to analyze market trends, customer demographics, and buying patterns to tailor marketing efforts, ensuring they hit the target audience right where it matters. For instance, a retail brand may analyze past purchase data to predict future buying habits, allowing them to adjust inventory or marketing pushes accordingly.
- Improved ROI: Through targeted campaigns based on data insights, marketers can see a clearer return on their investments.
- Segmentation: SPSS allows businesses to segment their audience more effectively, ensuring messages resonate with each group.
- A/B Testing: Marketers can simulate potential outcomes of different marketing strategies and compare them using SPSS data to determine the most effective approach.
"Data is the new oil; itâs valuable, but if unrefined canât really be used."
Utilization in Social Research
Social research relies heavily on data analytics to understand societal trends and human psychology. SPSS offers the statistical tools researchers need to sift through complex data sets efficiently. Researchers can analyze survey data to capture public opinion or demographic studies to understand shifts in societal norms.
- Survey Analysis: SPSS simplifies the process of extracting insights from vast amounts of survey data, enabling researchers to gauge public perception.
- Qualitative Data Interpretation: The software aids analysts in transforming qualitative responses into quantifiable data, presenting findings that resonate in the field.
- Trend Analysis: By applying various statistical tests, researchers can identify trends over time, providing a clearer picture of how sentiments and behaviors evolve.
Role in Healthcare Analytics
In the healthcare sector, SPSS serves as a cornerstone for data-driven decision-making. With the rise of electronic health records, the amount of data available has skyrocketed, and using SPSS helps healthcare providers to optimize patient care and operational efficiencies.
- Patient Outcomes: Healthcare administrators can analyze data related to treatment efficacy and patient outcomes, improving care strategies.
- Resource Allocation: By understanding patient trends, hospitals can allocate resources more effectively, reducing wait times.
- Health Trends: Analyzing data helps in tracking and predicting outbreaks or chronic health issues, enabling proactive strategies.
Impact on Business Strategy
SPS data analytics impacts business strategy through informed decision-making grounded in tangible insights. Companies that harness the power of SPSS can create competitive advantages by understanding market dynamics and internal performance metrics.
- Strategic Planning: Businesses can use data from SPSS to support long-term strategic planning, identifying opportunities and threats in the market.
- Performance Metrics: Utilizing KPIs derived from SPSS analysis helps organizations streamline processes and boost productivity.
- Predictive Analysis: Businesses can forecast future trends based on historical data, positioning themselves ahead of the curve.
In this fast-paced environment where data serves as the backbone for most strategic decisions, the applications of SPS data analytics are paramount to fostering a culture where data-driven decisions take precedence. Each sector discussed leverages these insights not only to enhance operational efficiencies but also to navigate complexities inherent to their respective fields.
As the realm of SPS data analytics continues to evolve, understanding and applying its principles remains essential for any organization aiming for sustained success.
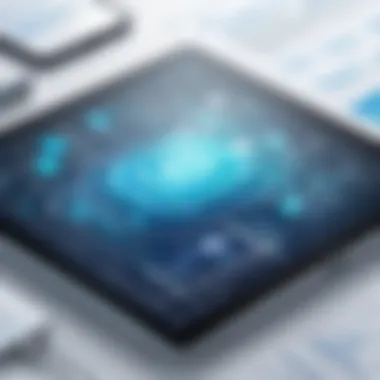
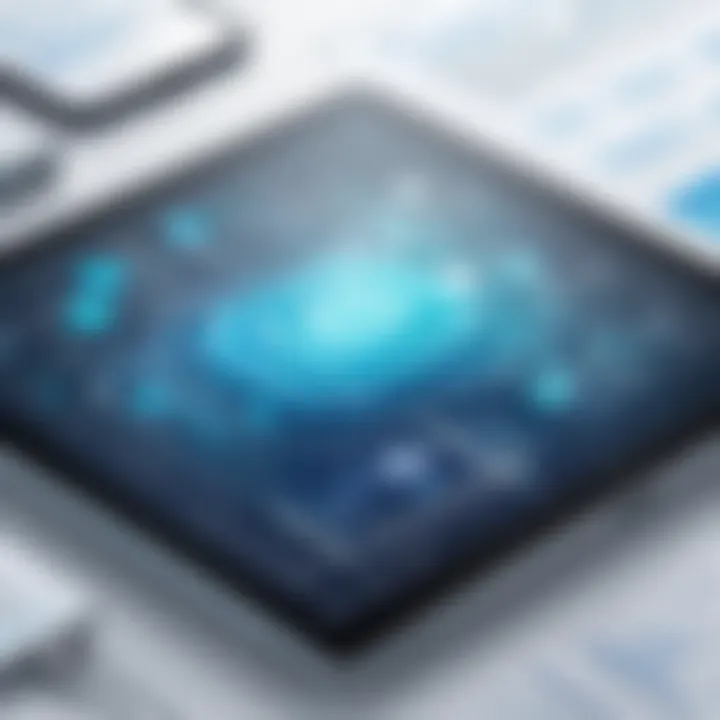
Best Practices in SPS Data Analytics
In an era where data drives decisions and shapes strategies, understanding and implementing best practices in SPS data analytics is paramount. These practices not only streamline the analytical processes but also ensure that the insights derived are both actionable and relevant. Businesses that leverage effective SPS analytics practices witness enhanced decision-making capabilities, as they can rely on data that is robust and well-structured. Here, we will delve into three imperative aspects: developing a data analytics framework, ensuring data quality and relevance, and fostering collaboration across disciplines.
Developing a Data Analytics Framework
To craft a solid data analytics framework, organizations must approach the task with a clear vision and methodical precision. This framework serves as a roadmap, guiding analysts and stakeholders through the labyrinth of data interpretation. A well thought out framework generally consists of the following components:
- Identification of Business Goals: Tailoring data initiatives to specific organizational objectives helps in aligning efforts with strategic outcomes.
- Data Collection Protocols: Establishing clear methods for data acquisition ensures that the information gathered is comprehensive, consistent, and timely.
- Analytical Techniques: Itâs crucial to choose the right techniques based on the complexity and nature of the data. For example, employing regression analysis for prediction or clustering techniques for segmentation can yield different insights.
Creating a framework is not a one-time affair. It requires frequent reviews and adjustments as new data challenges arise. As the saying goes, "a stitch in time saves nine"âthe earlier issues are addressed, the lesser the risk of compounded difficulties.
Ensuring Data Quality and Relevance
Quality data is like gold in gold miningâwithout high-quality input, the analytics will falter, leading to misleading conclusions. To maintain data quality, organizations can consider the following practices:
- Regular Audits: Performing periodic checks on the data can help identify inconsistencies that need correction. This process is much like checking your tires before a long trip; neglecting it might lead to disastrous outcomes.
- Standardization: Implementing uniform formats across datasets reduces the chances of errors and enhances comparability.
- Validation Techniques: Utilizing methods such as cross-verification and data triangulation ensures the authenticity of the data collected. Incorporating these practices can significantly increase the confidence in analysis results, thereby driving trust in the decision-making process.
Relevance, in this context, extends beyond merely ensuring high quality; it also emphasizes the importance of data being applicable to current business scenarios. Regularly revisiting the datasets ensures that insights remain pertinent to evolving market conditions and organizational needs.
Collaborating Across Disciplines
Given the complexity of modern data analysis, collaboration across various fields has become more crucial than ever. Leveraging diverse perspectives often results in richer insights. Here are a few ways in which inter-departmental collaboration can be fostered:
- Interdisciplinary Teams: Forming teams that include members from analytics, marketing, finance, and operations enables a comprehensive approach to data interpretation. Each discipline can offer unique viewpoints that enhance the analysis.
- Knowledge Sharing Platforms: Establishing forums or platforms for sharing analytical findings keeps everyone in the loop and opens the door for constructive feedback.
- Joint Training Sessions: Conducting workshops that involve multiple departments encourages a shared understanding of analytical methods and their implications. This can cultivate a data-centric culture across the organization, helping everyone play their part in data-driven strategies.
"A team that collaborates effectively unleashes the potential of data analytics in ways that no single discipline could achieve alone."
Adopting these best practices in SPSS data analytics does not merely optimize the analytical process; it fundamentally transforms how organizations make decisions. By establishing a structured framework, ensuring data quality, and fostering interdisciplinary collaboration, an organization can harness the full power of its data analytics initiatives, ultimately leading to smarter decisions and better outcomes.
Challenges in SPS Data Analytics
The field of SPS data analytics, while rich in potential, is not without its own set of challenges. Understanding these challenges is crucial for anyone looking to effectively implement and use the Statistical Package for the Social Sciences (SPSS) in their analytical practices. From navigating the complexities of data handling to addressing ethical considerations, these hurdles can significantly impact data-driven decision-making.
Specific elements worth considering include common pitfalls that can arise during the analytics process, the importance of addressing data privacy and ethics concerns, and the technological limitations that often hamper effective analytics. Recognizing and navigating these challenges not just prepares organizations for the potential issues, but also ensures better outcomes in their data analysis endeavors.
Common Pitfalls in Data Analytics
When embarking on a data analytics venture, organizations often stumble upon certain common pitfalls. These mistakes can severely skew results and lead to misguided strategies. Here are a few:
- Ignoring Data Quality: One fundamental error is taking poor quality data at face value. This can happen when data is collected without proper methods or without quality checks, leading to potential inaccuracies in findings.
- Overlooking Context: Data points exist within a context. Analyzing data in isolation, without understanding its background or relevance to current variables, can lead to misinterpretations.
- Neglecting Documentation: Failing to document processes and methodologies can create confusion down the line, making it difficult to replicate studies or verify results.
- Adopting One-size-fits-all Solutions: Using generic analytics solutions without customizing them for specific needs risks overlooking nuances, ending up with a less effective analysis.
By being aware of these pitfalls, professionals can take proactive measures to ensure their analytics projects are grounded in solid practices.
Data Privacy and Ethics Concerns
Data privacy is a hot topic in todayâs digital landscape. As organizations delve into SPS data analytics, they must grapple with significant ethical concerns regarding data usage. Mismanagement in this area not only risks violating regulations but also compromises public trust. Consider these important aspects:
- Compliance with Legal Standards: Organizations must stay abreast of legal frameworks like GDPR or HIPAA, enforcing strict measures to protect personal data.
- Informed Consent: Itâs not just about collecting data; data subjects should be informed of how their data will be used and whom it will impact.
- Minimization Principle: Collect only whatâs necessary for analysis. Hoarding unnecessary information can lead to complications, especially when trying to safeguard sensitive data.
Organizations need to prioritize ethical practices in their analytics to avoid repercussions that could arise from data mishandling.
Technological Limitations
While SPSS is a robust tool for data analysis, it is also subjected to technological limitations. Recognizing these limitations allows professionals to strategize effectively around them. Here are some key points to consider:
- Scalability Issues: As datasets grow larger, performance can suffer. SPSS may lag when processing vast amounts of data, necessitating efficient techniques to manage scalability.
- Integration Challenges: Combining SPSS with other analytics tools or data sources can prove tricky. Compatibility issues might arise when trying to harmonize data from diverse platforms.
- Learning Curve: Not all users pick up SPSS at the same rate. Even experienced analysts might encounter a steep learning curve when trying to leverage advanced features.
Understanding these limitations helps in setting realistic expectations and encourages users to seek out complementary tools and practices that enhance their analytics capabilities.
Future of SPS Data Analytics
In a world where data is generated at an unprecedented pace, the future of SPS data analytics holds immense significance. As organizations recognize the vast potential of data-driven insights, SPSS is becoming a fundamental tool in interpreting complex datasets. By probing into the forthcoming trends and challenges, businesses can prepare themselves for an evolving landscape.
Trends in Data Analytics Technologies
The realm of data analytics is constantly shifting. Some key trends are reshaping the landscape:
- Real-time Analytics: Gone are the days of waiting for reports. Organizations increasingly require insights at the momentâright when decisions need to be made. The integration of real-time analytics with SPSS means that data can be processed as it flows in, providing immediate insights and allowing organizations to act swiftly.
- Cloud-based Solutions: The rise of cloud computing is transforming how data is stored and processed. SPSS is now accessible through cloud platforms, which enhances collaboration and allows for greater scalability. This enables teams across geographies to work seamlessly, pulling and analyzing data as needed.
- Data Visualization: Visual storytelling continues to gain traction. As datasets grow, the ability to present findings in an intuitive manner is crucial. Advanced visualization tools integrated with SPSS can help transform raw numbers into compelling visuals, making data easily digestible for stakeholders.
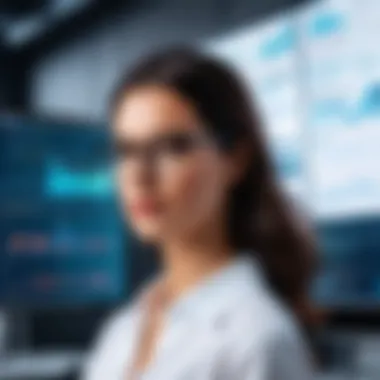
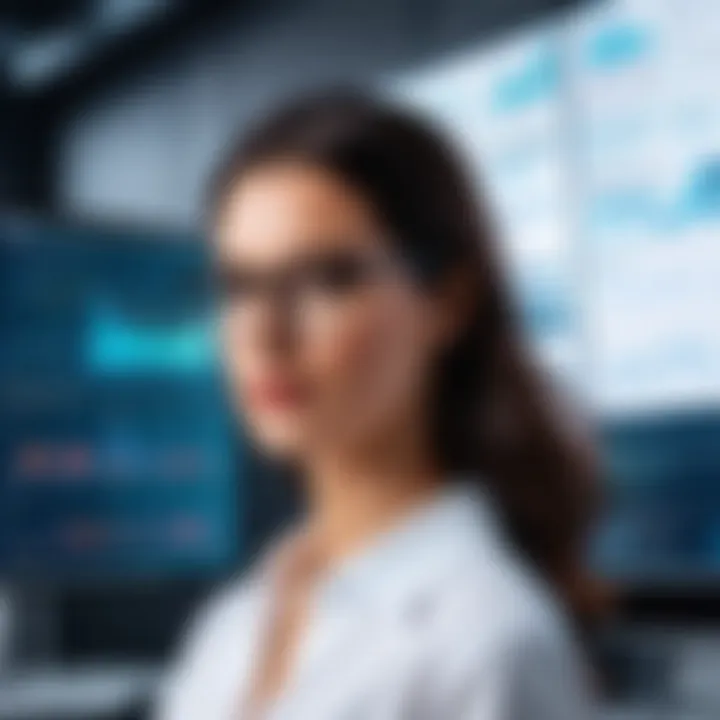
These trends underscore the need for organizations to stay ahead of the curve. As the digital landscape evolves, being adept in these areas will differentiate the leaders from the laggards.
The Growing Role of AI and Machine Learning
Artificial Intelligence and Machine Learning aren't just buzzwords; they're fundamental components shaping the future of data analytics. Their integration into SPSS offers fascinating possibilities:
- Predictive Analytics: With AI-enhanced algorithms, SPSS can analyze historical data to forecast future outcomes. This predictive capability is invaluable across industriesâbe it understanding consumer behavior in retail or predicting patient outcomes in healthcare.
- Automated Insights: Machine learning can streamline the analytical process. Instead of manually sifting through data, SPSS can automatically highlight trends and anomalies, allowing analysts to focus on strategy rather than data wrangling.
- Natural Language Processing: The ability for users to query data in plain language is becoming a reality. This makes SPSS more accessible to non-technical users, enabling a wider range of professionals to engage with data insights without needing extensive training.
By leveraging these advancements, businesses can harness the power of data more effectively, gaining competitive advantage.
Anticipating Future Challenges
While there is great promise in the future of SPS data analytics, it's not without challenges:
- Data Overload: With the abundance of data, distinguishing valuable insights from noise becomes increasingly difficult. Organizations must implement robust strategies for data management and filtering to avoid analysis paralysis.
- Skills Gap: The rapid evolution of technologies necessitates an ongoing commitment to training. As analytics tools become more sophisticated, ensuring that team members have the necessary skills will be paramount.
- Ethical Considerations: As organizations leverage data for decision-making, ethical concerns grow. Issues surrounding data privacy and unbiased algorithms must be addressed to maintain trust among consumers and stakeholders.
Becoming aware of these potential hurdles is crucial. By taking a proactive stance, organizations can devise plans to mitigate risks while fully capitalizing on the opportunities that arise.
"The future of data analytics is not just about technology; it's about people and processes standing strong together."
In summary, the future of SPS data analytics is poised for exciting growth, marked by transformative trends, innovative AI applications, and important challenges. Embracing these elements will mold the next generation of data-driven strategies.
Case Studies of Successful SPS Analytics Implementation
Case studies play a vital role in illustrating the practical application and significance of SPS data analytics across various sectors. By diving into real-world examples, professionals can glean valuable insights into effective strategies and methodologies, bolstering their understanding. The relevance of this section extends beyond mere academic discourse; it serves as a roadmap for practitioners seeking to implement similar strategies in their own environments. Key benefits of examining these case studies include:
- Practical Learning: Understanding how organizations navigate challenges and leverage data to inform decisions provides a concrete basis for action.
- Identification of Best Practices: Successful examples shine a light on effective approaches that can be adopted or tailored to fit unique circumstances.
- Awareness of Potential Pitfalls: Case studies also reveal common obstacles faced during implementation, enabling professionals to anticipate and mitigate risks.
Case Study: Marketing Success through Data Insights
In the world of marketing, leveraging data analytics can make or break a campaign. One remarkable case involves a consumer goods company that utilized SPS to track customer behavior across multiple channels. Through a meticulous analysis of purchasing patterns and feedback collected via surveys, the company discovered that their target audience was becoming increasingly health-conscious.
By synthesizing this data, the marketing team redefined their product line to emphasize organic ingredients, tailored advertising messaging, and adjusted pricing strategies accordingly. This pivot not only boosted sales by a staggering 30% within six months but also positioned the brand as a leader in the health-oriented product market.
A critical takeaway from this case is the necessity of continuously monitoring consumer preferences and adapting accordingly. It underscores how data-driven decisions can produce tangible returns when aligned with market realities.
Case Study: Enhancing Healthcare Efficiency
Another telling example can be found in a large metropolitan hospital that implemented SPS for improving patient care processes. The hospital faced long patient wait times and inefficiencies in handling emergency admissions. By adopting a data analytics approach, they meticulously analyzed historical patient data alongside operational metrics.
Utilizing SPSS, the healthcare team discovered peak admission times and patient flow patterns. They restructured schedules for medical staff and optimized resource allocation based on these insights. As a result, patient wait times decreased by 40% and overall satisfaction ratings improved markedly.
This case highlights the transformative power of data analytics in healthcare contexts. It reflects the paradigm shift from reactive to proactive management, offering implications for what can be achieved through informed operational decisions.
Case Study: Social Research Breakthroughs
In the field of social research, data analytics has demonstrated its power to reveal significant societal trends. A recent study conducted by a university class utilized SPS to examine the effects of remote work on employee mental health. Researchers collected data from surveys and social media platforms, seeking patterns and correlations in sentiments expressed online.
The SPSS analysis revealed a striking link between isolation levels and employee productivity metrics, facilitating a deeper understanding of modern workplace dynamics. This insight prompted further investigation and eventually resulted in a university policy shift aimed at enhancing mental health support for remote workers.
The lessons learned from this case indicate that data analytics can stimulate change not only in business practices but also in broader social policies. Through such case studies, stakeholders gain awareness of the ripple effect analytics can have across disciplines, paving the way for informed discussions and policies.
"The application of SPS analytics is not merely about crunching numbers; it's about transforming those numbers into actionable insights that drive change."
Exploring these case studies fosters a richer understanding of SPS data analytics, standing as examples of how thoughtful analysis can lead to substantial improvements in a range of domains.
Culmination
The conclusion of this article weaves together the various threads discussed throughout the exploration of SPS data analytics. It serves as a critical platform to underscore the significance of data-driven decision-making across a multitude of sectors. With SPSS at the helm, professionals can engage profoundly with data, transforming raw numbers into actionable insights. This empowers businesses of all sizes to elevate their strategies and operational efficiency.
Summation of Key Insights
To encapsulate the key takeaways, consider the following points:
- Versatility of SPSS lies in its ability to accommodate diverse data types and analysis requirements, making it a go-to tool in various fields, including marketing and social sciences.
- Methodologies discussed, such as advanced statistical analysis and thoughtful data preparation, highlight the foundational practices that enhance validity and usability of the results.
- Challenges like data privacy and technological limitations serve as reminders to remain vigilant and proactive in the analytics journey.
- Future trends, including the integration of AI, signal a shift towards more predictive and prescriptive analytics, reshaping the landscape of how data insights are generated and consumed.
The integration of these insights provides a roadmap for professionals looking to harness SPS data analytics effectively.
Final Thoughts on SPS Data Analytics
As the world grows increasingly data-centric, the relevance of SPSS and its methodologies cannot be overstated. Businesses that adapt to leverage data analytics will find themselves ahead of the curve, benefiting from informed decision-making and strategic foresight.
In a society where data is often seen as the new oil, understanding how to extract value from it becomes paramount. Emphasizing best practices not only ensures high-quality results but also builds trust in the data used to drive business outcomes.
"Data doesn't just speak for itself; it requires a proficient listener to translate its message into actionable strategies."
Ultimately, navigating the complexities of SPSS data analytics poses challenges, yet also presents immense opportunities for growth, innovation, and enhanced organizational efficacy. Embracing this journey leads to a future where data is not just collected but intelligently utilized for optimal impact.