Understanding Big Eye Data Quality in Depth
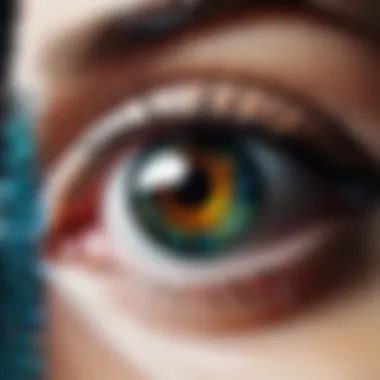
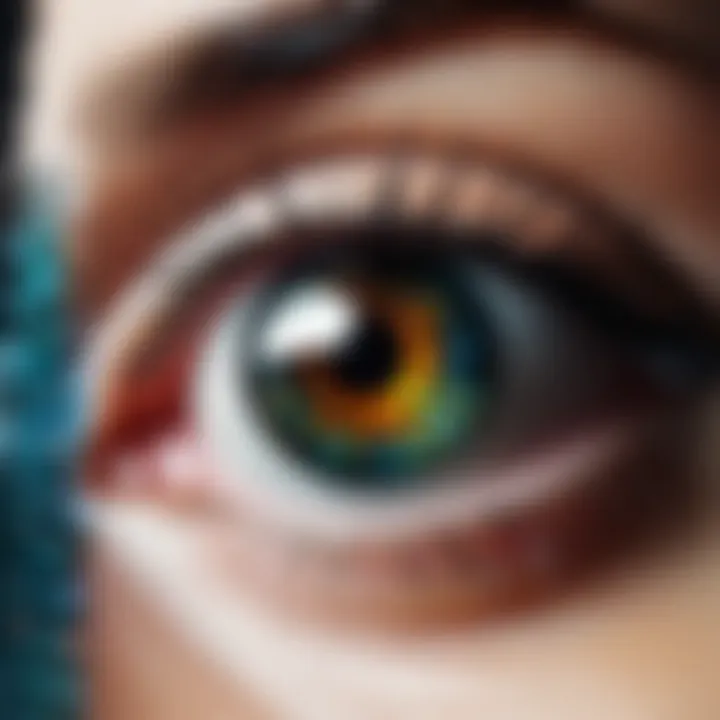
Intro
In an age where data flows like a river, understanding the quality of that data becomes critical, especially when it comes to big eye datasets. These datasetsâcharacterized by their enormous volume, velocity, and varietyâpose unique challenges and opportunities in the realm of data quality. As organizations lean heavily on data for decision-making, ensuring its integrity, accuracy, and consistency cannot be overstated. The stakes are high, from improving operational efficiency to gaining a competitive edge in the marketplace.
Navigating through the intricacies of big eye data quality opens up a treasure trove of insights. The following sections will peel back the layers of this complex topic, discussing foundational principles, common pitfalls, and practical strategies for enhancing data integrity. By the end, technology professionals and enthusiasts alike will walk away better equipped to elevate their data practices.
Prolusion to Big Eye Data
In today's rapidly changing world, data is the lifeblood of decision-making. Big Eye Data, known for its extensive scope and intricate details, plays an increasingly critical role. Understanding it goes beyond just being a buzzword; it requires digging deep into what makes this data valuable and relevant. Companies, be they small startups or towering enterprises, rely heavily on high-quality data to navigate through their everyday challenges, seize opportunities, and ultimately drive their success.
Data today comes in volumes that would make anyone's head spin, and that is why dissecting Big Eye Data is essential. This section aims to highlight how significant Big Eye Data is in the digital arena by emphasizing specific elements like its properties, benefits, and the overarching considerations regarding data quality. Whether itâs for strategic planning, operational efficiency, or risk management, harnessing the power of Big Eye Data could be the difference between thriving and merely surviving in a competitive environment.
Defining Big Eye Data
Big Eye Data refers to vast volumes of complex datasets that organizations gather from myriad sources, often in real-time. This encompasses social media postings, sensor data from IoT devices, transactional data, and an array of other information streams. The unique aspect of Big Eye Data is that it encompasses not just the raw numbers but also layers of context that can drive insightful decisions.
One key feature of Big Eye Data is its ability to capture a diverse array of characteristics â from qualitative insights provided by social media sentiments to quantitative metrics derived from sales transactions. This diverse nature makes it multifaceted, and it can be utilized to paint a holistic picture of market trends, customer preferences, or operational workflows efficiently.
When organizations effectively define and curate their Big Eye Data, they open a floodgate to potential insightsâsomething that could radicalize their approaches to business strategy.
The Growing Importance of Data Quality
As organizations increasingly turn to Big Eye Data for insights, the importance of maintaining data quality cannot be overstated. Good data quality is not just an added bonus; it's a necessity. Poor quality data can mislead organizations, lead to faulty conclusions, and ultimately tarnish their reputations.
Data quality is often measured against certain benchmarks:
- Accuracy: Does the data reflect the true state of affairs?
- Completeness: Is all necessary data collected and available for analysis?
- Consistency: Do all data sources speak the same language?
- Timeliness: Is the data up-to-date and relevant to current conditions?
- Uniqueness: Is the data free from duplication?
When data is accurate, complete, consistent, timely, and unique, organizations can make informed decisions that align with their strategic goals. Furthermore, embracing quality data enhances operational efficiency, ensures compliance with regulations, and supports risk management initiatives, fundamentally transforming how business operates.
"High data quality is no longer optional; it is crucial for driving effective decision-making and maintaining a competitive edge."
By prioritizing data quality, organizations can unlock the full potential of their Big Eye Data, ensuring they harness insights that foster growth, innovation, and sustainability.
Dimensions of Data Quality
In the realm of big eye data, dimensions of data quality form the very backbone of effective data utilization. Understanding these dimensions equips professionals with the tools needed to assess data accurately and ultimately make informed decisions. Each dimension plays a unique role in ensuring that the data harnessed aligns with organizational goals, propelling businesses towards success.
Accuracy
Accuracy is a critical dimension that refers to how closely data values conform to the actual or true values. Itâs crucial because, without accuracy, decision-making can falter like a ship without a compass. For instance, consider a company that relies on sales data to forecast future demand. If that data contains errors or misreporting, the forecasts may be completely off base, leading to oversupply or stock shortages.
Itâs not just about having the right numbers; itâs about ensuring those numbers are rooted in reality. Regular validation processes, such as cross-checks and audits, can aid in maintaining accuracy.
Completeness
Completeness addresses whether all required data is present. Incomplete datasets can lead to a skewed understanding of the circumstances surrounding decisions. For example, imagine a marketing team that only has partial customer demographics. This lack of information may result in misguided targeting strategies, wasting resources and missing potential revenue.
Organizations should strive to collect and maintain comprehensive datasets. Implementing data governance frameworks can ensure all necessary information is gathered right from the outset, preventing issues stemming from missing pieces down the line.
Consistency
Consistency measures whether data aligns and remains uniform across various datasets. For instance, if a customerâs name is spelled differently in two databases, this inconsistency can cause chaos in communications and lead to distrust from clients. Achieving consistency often requires robust integration processes to ensure all data sources reflect the same information. Utilizing standardized formats, naming conventions, and regular reconciliation methods is key, allowing stakeholders to trust that they are working with the same information.
Timeliness
Timeliness refers to the degree to which data is up-to-date. Outdated information can be as dangerous as no information at all. In many industries, timing is key to maintaining a competitive edge. A retailer that uses last yearâs sales data to make inventory decisions might find themselves stuck with products no one wants anymore. To enhance timeliness, organizations should assess their data refresh cycles and establish real-time data feeds when necessary. Regular reviews of data and updates can keep everything fresh and relevant.
Uniqueness
Uniqueness assesses whether each record is represented once within a dataset. Redundant data not only clutters storage but can confuse business intelligence efforts. A common scenario is a customer who makes multiple purchases being counted multiple times in sales analysis. This can result in inflated figures that misguide strategic decisions. Techniques like deduplication and data cleaning can be employed to maintain uniqueness, allowing for a more accurate representation of data.
"A fine business reputation is built upon a solid foundation of good quality data."
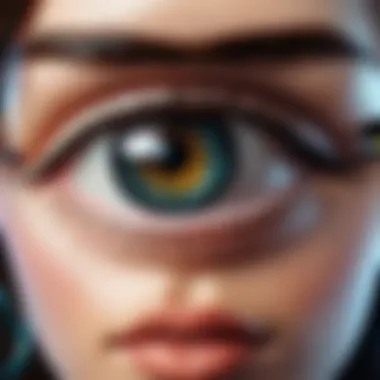
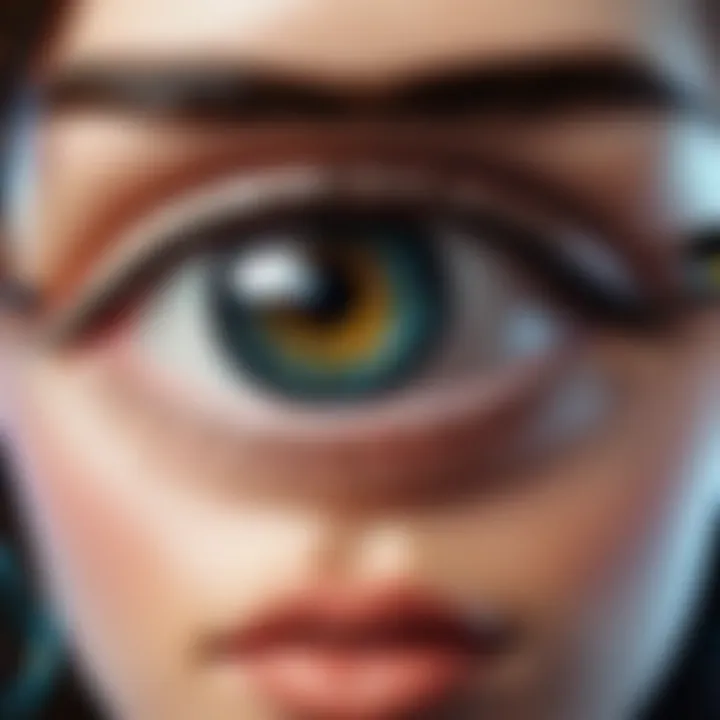
In sum, understanding these dimensions of data quality and putting them into practice allows organizations to navigate the complex waters of data management. Their interplay directly influences data integrity, decision-making processes, and ultimately, the ability to drive business success.
Challenges in Maintaining Data Quality
In the realm of big eye data, maintaining data quality isn't merely a checkbox on a project plan; it's an integral component that can make or break the success of any data-driven initiative. Organizations today collect vast amounts of data at a staggering pace, but this relentless inflow does not guarantee the correctness or reliability of that data. As data becomes more dynamic and intricate, understanding the challenges that impede data quality is crucial for IT and software professionals aiming to elevate their practices and decision-making processes.
Volume and Velocity of Big Eye Data
The sheer volume and velocity at which big eye data moves can be quite daunting. Organizations often find themselves inundated with information, with streams of data flowing in from an array of sources, such as social media, IoT devices, and online transactions. This rapid influx can overwhelm existing systems, leading to various issues related to data quality.
High volume likens to trying to fill a bathtub with a fire hoseâif not managed properly, clean data can easily become muddied. Data retrieval processes might lag, causing outdated information to linger in systems. Furthermore, rapid data processing might result in filtering out valuable insights or misinterpreting incoming data patterns. Therefore, it becomes essential that every department involved establishes robust data handling protocols and infrastructures that can accommodate both high volume and high velocity efficiently and effectively.
Data Integration Issues
Integrating data from multiple heterogeneous sources can be another minefield in ensuring data quality. Different systems often have different formats, standards, and meanings attached to the same variables. For instance, one data source may categorize customer age groups differently than another, leading to conflicting insights if improperly integrated.
- Data Silos: Often, departments work in isolation which creates silos of data. Each team develops its own sets of standards and validation techniques. This lack of cohesion results in inconsistent information across the organization and creates headaches when trying to obtain a unified view of data.
- ETL Challenges: Extract, Transform, Load (ETL) processes can become complicated when aligning data from disparate sources. If not correctly executed, data integrity suffers, making gleaning meaningful insights a challenging task.
To mitigate these integration issues, organizations need to prioritize harmonizing their data standards and employing robust ETL tools to ensure consistent and accurate data flow. With such efforts, the likelihood of discrepancies can be lowered significantly.
Evolving Data Standards
The data landscape is constantly in flux, with new technologies and methodologies emerging regularly, and existing standards evolving to adapt to these changes. When organizations fall behind in keeping pace with evolving data standards, they risk jeopardizing the quality of their data. New regulations or practices may surface, requiring firms to adjust their data governance frameworksâanything short of this might render their data obsolete or non-compliant.
- Flexibility: Organizations must cultivate a culture of flexibility and adaptability. Whether itâs integrating newer predictive analytics techniques or aligning with recent GDPR regulations, teams must be ready to adjust their practices without considerable disruption.
"Adapting to change is not optional; it is a necessity for maintaining data quality in a digital world that never sleeps."
- Training: Continuous education for stakeholders is vital. Keeping personnel informed about changing standards and practices enables a proactive approach to data integrity. Regular training sessions and workshops can facilitate this.
Assessing Data Quality
Assessing data quality stands as a pivotal aspect in the realm of big eye data. It not only implies measuring how well data fits the needs of an organization but also outlines processes that help maintain the integrity of this data over time. As organizations increasingly rely on data for making critical business decisions, understanding the quality of this data becomes essential.
The importance of assessing data quality can be underscored through several key elements. Firstly, knowing the quality of data helps in pinpointing any issues that might impede decision-making processes. For instance, if sales figures come from an unreliable dataset, the strategies based on that data can lead a company astray, potentially costing them not just financially but also in terms of reputation.
Moreover, this has a ripple effect on operational efficiency. When good data practices are in place, employees can spend less time clarifying conflicting information and more time focusing on actual productivity. In turn, this reduces operational costs and fosters a clearer path towards achieving strategic goals. However, organizations must pay attention to specific considerations when assessing data quality. It's not just about tools or metrics; it's about an ingrained culture of valuing data integrity.
Quality Metrics and KPIs
Quality metrics and key performance indicators (KPIs) serve as the backbone of assessing data quality. Organizations ought to cultivate defined metrics to evaluate their data rigorously. Commonly, the metrics such as accuracy, completeness, and consistency are mainstream in discussions. But what's often overlooked are the bespoke metrics tailored for specific industries or organizations. For example:
- Accuracy: Measures if the data correctly reflects the real-world situation.
- Completeness: Examines whether all required data is available, such as ensuring customer information contains all pertinent fields.
- Consistency: Checks if the same data point shows up in different datasets without discrepancies.
Setting KPIs based on these metrics allows organizations to maintain a snapshot of data health over time. Monitoring these indicators regularly can aid in recognizing patterns that might indicate deeper issues, like a particular segment of data that consistently falls short on completion.
Data Profiling Techniques
Data profiling involves scrutinizing the actual data within a dataset to understand its structure, content, and relationships. This process can reveal a treasure trove of information about data quality and help in diagnosing issues that affect quality. Techniques like
- Frequency Analysis: Helps in understanding the distribution of values within a dataset.
- Pattern Matching: Identifies deviations from expected formats, such as finding telephone numbers that don't conform to the expected structure.
- Null Value Analysis: Indicates data gaps, directing attention where improvements are needed.
By employing these techniques, organizations can uncover hidden data anomalies and take corrective measures proactively, ensuring data remains a valuable asset rather than a hindrance.
Audit and Monitoring Procedures
Implementing robust audit and monitoring procedures is non-negotiable for maintaining high data quality. These processes help to ensure that data remains compliant with industry regulations and internal policies throughout its lifecycle. Audits can take many forms, from manual checks to automated scripts that flag irregularities.
Regularly scheduled data audits can reveal areas where data quality processes are failing. For instance, if an audit shows high levels of inconsistency in sales reports, teams can investigate root causes, perhaps discovering that data entry systems are failing to capture information accurately.
Furthermore, ongoing monitoring allows organizations to set alerts for certain thresholds of data quality metrics. For example, if the percentage of missing data points exceeds a specific limit, an alert can trigger an immediate investigation. This level of vigilance creates an environment where data quality is not a one-time goal but an ongoing mission, thereby further embedding a culture of accountability around data integrity.
"Assessing data quality is not just about finding faults but about building a data ecosystem where trust can flourish."
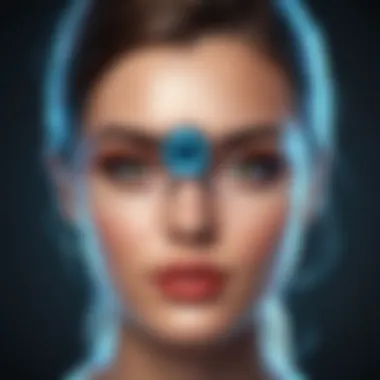
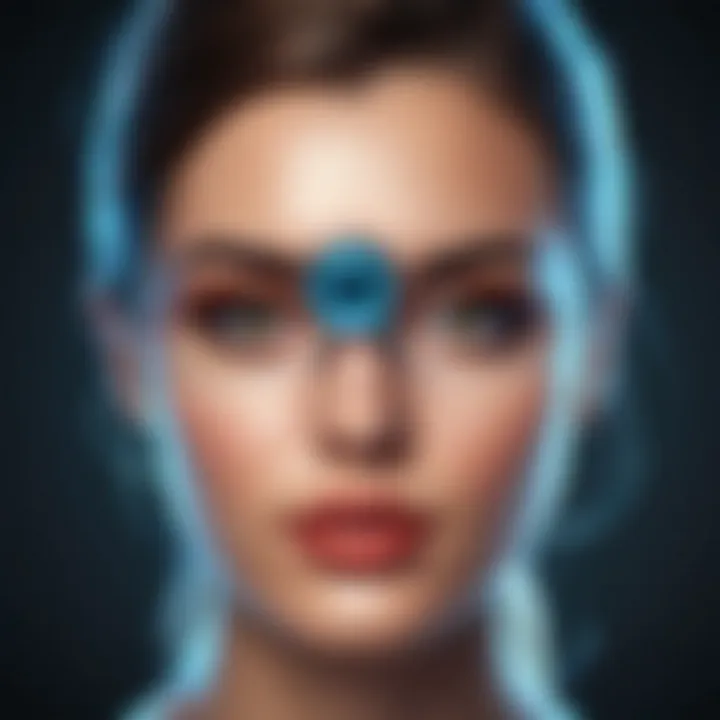
Improving Data Quality
Improving data quality is not just about ensuring that the information you have is accurate; it's about establishing a solid foundation for every facet of your operations. In a world driven by data, the integrity of that data can spell the difference between success and failure. Organizations that take this seriously often find themselves ahead of the game, making informed decisions that increase efficiency and reduce risks. Investing in data quality enhancement is akin to tuning a fine instrument, where even the smallest adjustment can yield harmonious results in analysis and strategy implementation.
Data Governance Framework
A strong data governance framework serves as the backbone of effective data quality efforts. This framework defines who is responsible for data-related decisions and how those decisions are made. When set up correctly, it ensures that everyone in the organization understands their role in maintaining data integrity.
Here are some key elements of a solid governance framework:
- Roles and Responsibilities: Clearly outline who does what, from data owners to data stewards. Each person should understand their contribution to quality.
- Policies and Procedures: Codify how data is handled, stored, and shared within the organization. This ensures that everyone follows the same protocols, minimizing the risk of errors.
- Data Quality Standards: Define what good data looks like. This can be in terms of accuracy, completeness, and format. Everyone must align with these standards.
Implementing a governance framework can initially seem daunting, but it streamlines processes and provides clarity and structure, fostering a culture of accountability around data management. When everybody knows their lane, itâs easier to drive toward high-quality data.
Implementation of Data Validation Protocols
Once a track is set, organizations must implement data validation protocols to catch errors before they snowball. Data validation involves systematic checks on information being entered into systems, aimed at ensuring that only accurate, complete, and relevant data is processed. Rather than a reactive approach, this proactive measure can save time and resources in the long run.
Some practical steps to establish data validation include:
- Automated Checks: Utilize software tools that can perform automatic validations, reducing the burden on staff and increasing accuracy. An example would be using a platform like Talend or Informatica.
- Manual Review Processes: Not all data can be checked by machines. Some may require human judgment to identify nuances and context.
- Feedback Loops: Encourage users to report data quality issues. This insight can be invaluable in honing the validation protocols continuously.
"An ounce of prevention is worth a pound of cure." Implementing data validation is exactly thatâa little effort goes a long way toward preventing larger issues.
Training and Development for Stakeholders
The importance of training cannot be overstated. Data quality efforts will falter if the people handling the data aren't equipped with the right skills and knowledge. Training and development initiatives must be tailored to different audiences within the organization, considering their specific roles related to data handling.
Key aspects to consider in this area include:
- Awareness Programs: Start with initial workshops or seminars aimed at raising awareness about data qualityâs significance. Such programs can help instill a culture of quality within the organization.
- Role-Specific Training: Offer training sessions that are relevant to different stakeholders' daily tasks. For example, data entry personnel may need to focus on accuracy, while analysts might be more interested in assessing completeness and consistency.
- Ongoing Development: Data quality is not a one-time effort. Regular training sessions can adapt as data landscapes become more complex, particularly with the rise of new technologies.
Providing continuous training will ensure that employees remain engaged and informed, ultimately contributing significantly to elevating the overall data quality within their teams.
Improving data quality requires a multifaceted approach. Through a well-defined governance framework, robust validation protocols, and thorough training for stakeholders, organizations can position themselves to leverage data as a strategic asset. The journey may be gradual, but the destinationâa culture of qualityâcan be transformative.
Technologies Supporting Data Quality
In the contemporary landscape of data management, technology serves as the backbone for maintaining quality in big eye datasets. These advancements not only aid in rectifying inaccuracies but also streamline processes so businesses can harness the true power of their data. Organizations, both big and small, recognize that without reliable data quality, their decision-making processes can become muddled, affecting various operational aspects.
Data Quality Tools and Software
Data quality tools and software are increasingly seen as vital assets in the toolkit of any data management strategy. They serve a various functions including data cleansing, profiling, and monitoring, which collectively enhances the overall data quality. Examples such as Talend Data Quality, Informatica, and IBM InfoSphere offer real-time data analysis and insights.
Here are some specific benefits:
- Accuracy: They help spot and correct inaccuracies in datasets, minimizing the time spent on troubleshooting data issues.
- Automation: Tools allow repetitive tasks to be automated, boosting operational efficiency.
- Collaboration: They enable team members to collaborate more effectively by providing centralized access to data.
One key consideration when selecting a data quality tool is ensuring it aligns with existing systems and processes within your organization. Not all software integrates seamlessly, which can lead to further complications.
Artificial Intelligence in Data Quality Management
Artificial Intelligence is shaking up the industry, acting as a game-changer in data quality management. AI-powered solutions can analyze massive datasets far faster and with more accuracy than traditional methods. They identify patterns, anomalies, and trends that may fly under the radar of human analysts. Here are several ways AI enhances data quality:
- Predictive Analytics: AI systems can predict potential data quality issues before they even arise, allowing preemptive measures.
- Natural Language Processing: AI can process unstructured data, which is becoming increasingly relevant in a big data context.
- Machine Learning: This enables iterative improvements based on ongoing data assessments, leading to continuous quality enhancement.
Implementing AI in your data quality strategy isnât without its challenges. Organizations must tackle issues like data bias and ensure AI deployments comply with ethical guidelines. However, the rewards often outweigh the risks.
Automating Data Quality Processes
Automation in data quality processes can drastically streamline operations. By automating manual tasks, businesses can reduce human error and free up their employee's time for more strategic work. Here are some notable advantages of automation:
- Speed: Immediate identification and correction of data inconsistencies increases overall productivity.
- Consistency: Automated processes maintain uniformity across datasets, which is essential when managing large volumes of data.
- Scalability: As the amount of data continues to grow, automation enables organizations to scale their data quality initiatives without exponentially increasing workload.
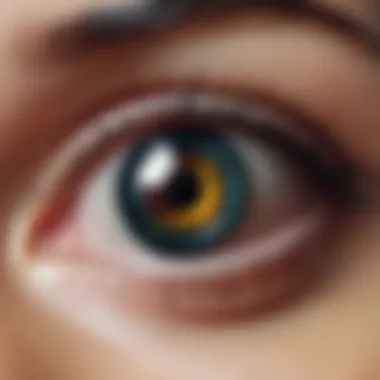
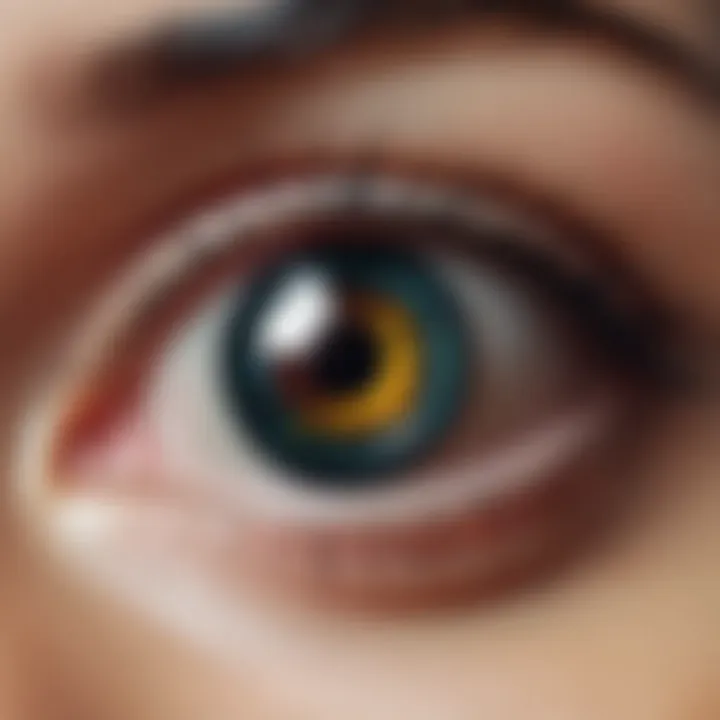
"Automation isn't just a trend; it's a necessity in our data-driven world. The ability to automate ensures that data quality is not an afterthought but an integral part of processes."
Tools like Informaticaâs Data Quality or Talend can simplify these processes. When using automation, itâs crucial to monitor the outputs continually to ensure that they align with expected quality standards.
In essence, incorporating advanced technologies not only mitigates risks associated with poor data quality but also empowers organizations to make informed decisions based on reliable, high-quality data.
The Business Impact of High Data Quality
In today's fast-paced, data-driven environment, the quality of information an organization utilizes can be the difference between hitting a home run or striking out. High data quality can drive better business outcomes, promote effective strategies, and help mitigate risks that could lead to costly mistakes. It's not just about having data; it's about having the right data, and hereâs why that matters.
Influence on Decision-Making
Decisions are only as good as the information that back them up. When decision-makers rely on high-quality data, they gain a clearer view of the landscape, leading to informed choices that resonate throughout the organization. For instance, businesses like Starbucks leverage data analytics to determine new store locations by analyzing customer behavior and preferences, which ensures they invest wisely.
"Data-driven decisions are the vital signs of business health"
Low-quality data can throw a wrench in the works, causing uncertainties and tantalizingly incorrect insights. Erroneous data often leads to missed opportunities or misguided strategies, as seen in the infamous case of Target, where inaccurate data on customer buying habits led to a major public relations fiasco. In contrast, firms that prioritize data quality can create a culture wherein every department, from marketing to finance, makes decisions that are both timely and relevant.
Operational Efficiency and Cost Reduction
Consider this: when data is consistent and accurate, it removes redundancies and streamlines operations. High data quality allows companies to reduce the time spent reconciling errors and maintaining data integrity. This translates into lower operational costs and a more agile workforce. For example, a company like Amazon relies on precise inventory data to manage stock levels efficiently, minimizing excess inventory while ensuring product availability.
Moreover, effective data quality management can lead to lower costs associated with compliance. Companies that invest in high-quality data have fewer fines or issues related to regulatory requirements, meaning that a focus on data quality can save significant financial resources. To put it simply, spending effort on data quality today prevents much larger expenses tomorrow.
Regulatory Compliance and Risk Management
Navigating regulatory frameworks can be a minefield without the right data. High-quality data ensures an organization can meet compliance requirements, which can vary widely by industry. For businesses in finance or healthcare, for instance, improper data management can lead to severe penalties.
Maintaining accurate and complete data allows companies to remain compliant with regulations such as the GDPR or HIPAA, mitigating risks associated with potential breaches or violations. Furthermore, understanding patterns in data can also aid in identifying potential risks before they escalate into issues. For instance, using quality data in predictive analytics can help anticipate cash flow problems, allowing for preemptive financial strategies.
In summary, the impact of high data quality reverberates through multiple facets of a business. From enhancing decision-making and promoting operational efficiency to ensuring compliance and mitigating risks, investing in data quality is not merely a technical choice, but a strategic business imperative.
Future Trends in Data Quality
In the rapidly evolving landscape of information technology, keeping an eye on the future trends in data quality is vital. As organizations become ever more reliant on data for strategic decision-making, understanding the emerging trends can provide a competitive edge. The confluence of advanced technologies and shifting operational landscapes means that businesses must adapt to sustain their data integrity and relevance. Identifying these trends not only helps organizations protect their data assets but also enables them to leverage opportunities for growth and efficiency.
Emerging Technologies
The rise of emerging technologies is reshaping how businesses approach data quality. Innovations like blockchain, internet-of-things (IoT), and robust machine learning algorithms are making waves. For instance, blockchain offers an immutable record of transactions, thus enhancing the credibility of data sources. In sectors like finance, where accuracy is non-negotiable, this technology opens doors to transparent data flow. Additionally, IoT generates an unprecedented amount of data, necessitating new mechanisms to assess and ensure its quality at scale. Organizations must align their data practices with these advancements to remain agile and informed.
"Staying ahead of the curve means not just adopting technology but ensuring data quality remains a cornerstone of business strategy."
The Shift Towards Predictive Analytics
Predictive analytics has gained traction as it enables organizations to anticipate future trends and behaviors, ultimately leading to enlightened decision-making. As data systems mature, businesses will increasingly rely on predictive models crafted from large sets of clean, high-quality data. This transition is significant; if data quality falters, the predictive insights lose their validity, leading to misguided decisions. Moreover, predictive analytics is becoming more accessible to both small and large enterprises, thanks to advancements in software tools and cloud-based solutions, which often incorporate built-in data quality checks.
Increasing Demand for Data-Driven Decisions
The emphasis on data-driven decision-making is palpable across industries. As companies begin to realize the power of big eye datasets, there's a pressing need for high standards of data quality to support this trend. The push for evidence-based strategies can be seen in every corner of the business spectrumâfrom marketing initiatives driven by customer behavior data to operational intents optimized through analytical insights. With this demand, businesses face challenges related to data governance and user accessibility; a strategic focus on data quality will facilitate better analytics and operational decisions.
In summary, the future of data quality is intrinsically linked with technology and shifting business paradigms. Organizations are encouraged to embrace these changes proactively, ensuring they invest in data quality measures that can withstand the test of time.
End
In concluding this comprehensive exploration of big eye data quality, itâs essential to reflect on the multifaceted nature of the topic. As organizations navigate through the complexities of vast datasets, the sustained focus on data quality becomes non-negotiable. This isn't just an operational concern â it's a fundamental component for strategic decision-making that fundamentally shapes business outcomes.
Summary of Key Findings
- Importance of Data Quality
High-quality data is the bedrock of effective decision-making. Organizations that prioritize data quality often see improved insights that drive innovations and enhance customer satisfaction. - Challenges Encountered
The dynamic nature of big eye data introduces unique hurdles, including issues related to volume, integration, and evolving standards. Understanding these challenges is critical for devising robust data management strategies. - Technologies and Methodologies
Numerous tools and methodologies have emerged to help businesses improve data quality. From automated data cleaning processes to advanced AI applications that monitor data integrity, the landscape is rich with solutions tailored to todayâs data-driven needs.
Final Thoughts on Big Eye Data Quality
Reflecting on big eye data, it's clear that organizations must embrace a culture of data integrity. In today's fast-paced environment, where data often fuels competitive advantages, overlooking the nuances of data quality is akin to sailing in uncharted waters without a compass. To truly reap the rewards of data-driven initiatives, organizations must diligently invest in maintaining data quality, which not only mitigates risks but also elevates operational efficiency.
As we peer into the horizon of future trends in data quality, it seems the trajectory is only going upward. Increasing reliance on predictive analytics and emerging technologies promises to refine our approach even further, fostering environments where decisions can be data-backed and insight-driven, always anchored in the quality of information at hand.
"Data is the new oil! But itâs only valuable if refined."
In essence, the focus on big eye data quality isn't merely a trend; it's a clarion call for modern enterprises to align their data management with strategic imperatives. Taking these lessons to heart equips IT professionals, software developers, and businesses of all sizes with the mindset needed to navigate the intriguing and often challenging seas of big data.