Unlocking the Potential: The Impact of Qlik Predictive Analytics
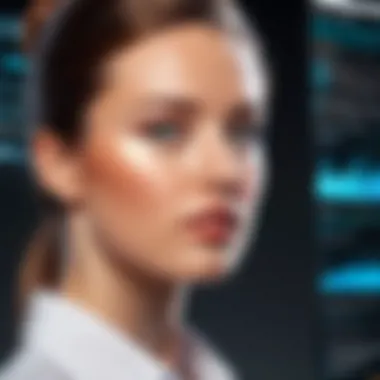
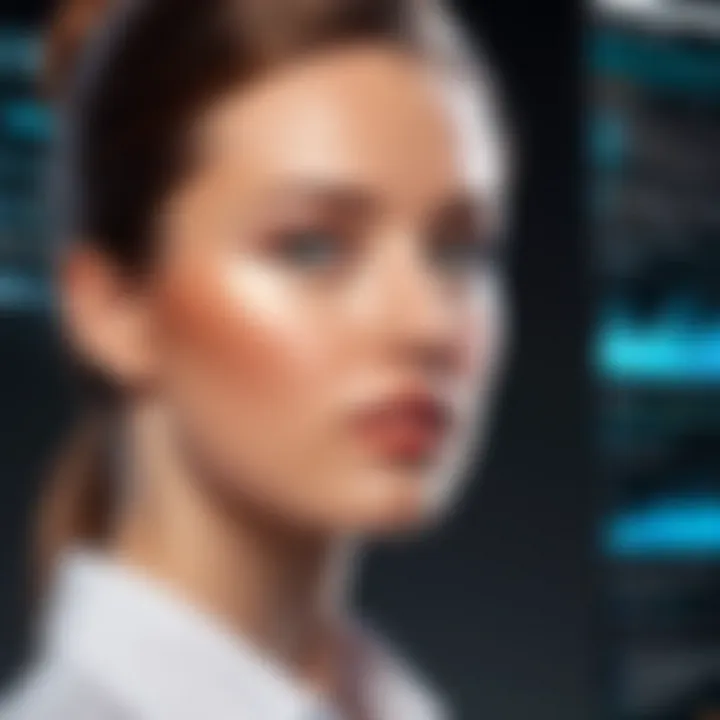
Software Overview
Qlik Predictive Analytics is a sophisticated tool renowned for its advanced features and functionalities in the realm of data analytics. The software offers a wide array of tools for predictive modeling, data visualization, and real-time data analysis, making it a valuable asset for businesses across various industries. In terms of pricing and licensing options, Qlik provides flexible packages tailored to the needs of individual users, ranging from small businesses to large enterprises. Additionally, the software is compatible with multiple platforms, ensuring seamless integration with existing systems.
User Experience
When it comes to user experience, Qlik Predictive Analytics excels in its intuitive interface design and ease of use. The platform offers customizable user settings, allowing users to tailor their experience to their specific requirements. Moreover, the software is known for its exceptional performance and speed, enabling users to analyze large datasets efficiently and derive actionable insights in real-time.
Pros and Cons
Among the strengths and advantages of Qlik Predictive Analytics are its powerful predictive modeling capabilities, interactive data visualization tools, and robust data security features. However, some users have noted limitations in terms of scalability and complex implementation processes when compared to similar products on the market. A thorough comparison with competing products can shed light on the unique offerings of Qlik in the predictive analytics landscape.
Real-world Applications
In real-world applications, Qlik Predictive Analytics finds widespread use in diverse industries such as retail, healthcare, finance, and manufacturing. Through various case studies and success stories, it becomes evident how the software has helped organizations gain valuable insights, optimize processes, and make data-driven decisions. By addressing specific challenges faced by different industries, Qlik proves to be a versatile solution for a wide range of business applications.
Updates and Support
To ensure optimal performance and user satisfaction, Qlik provides regular software updates to enhance functionality and address any issues that may arise. In addition, customers can access a range of support options, including customer service channels and community forums, for assistance with technical queries or product guidance. The availability of user resources further empowers users to maximize their utilization of the software and collaborate with a thriving community of Qlik enthusiasts.
Introduction to Qlik Predictive Analytics
Qlik Predictive Analytics unfolds a realm of data-driven insights and strategic decision-making. In this informative article, we plunge into the depths of Qlik Predictive Analytics, unraveling its significance in revolutionizing analytical processes. Highlighting the intricate balance between technological advancements and predictive modeling, this section serves as a foundational pillar for comprehending the power and potential of predictive analytics within diverse industries.
Understanding Predictive Analytics
Definition and Concept
Delving into the core essence of Predictive Analytics, this segment accentuates its role in extrapolating future trends based on historical data patterns. By employing sophisticated algorithms and statistical analyses, Predictive Analytics offers invaluable foresight essential for preemptive decision-making in dynamic business landscapes. The intrinsic feature of predictive modeling lies in its ability to predict outcomes with a high degree of accuracy, empowering organizations to anticipate trends and capitalize on opportunities effectively.
Importance in Data Analysis
The integration of Predictive Analytics in data analysis revolutionizes conventional approaches, elevating insights from mere observations to proactive strategies. By integrating predictive models, organizations can unearth hidden patterns and correlations, enabling them to make data-driven decisions with greater confidence and precision. The salient feature of Predictive Analytics in data analysis is its capacity to identify potential risks and opportunities, providing a strategic advantage in competitive markets.
Evolution of Qlik Predictive Analytics
Key Milestones
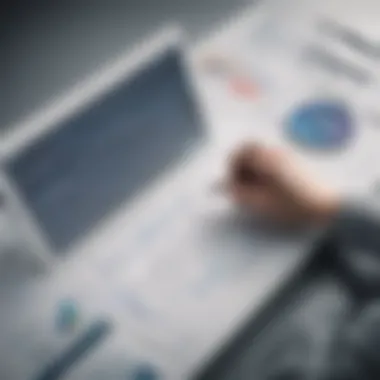
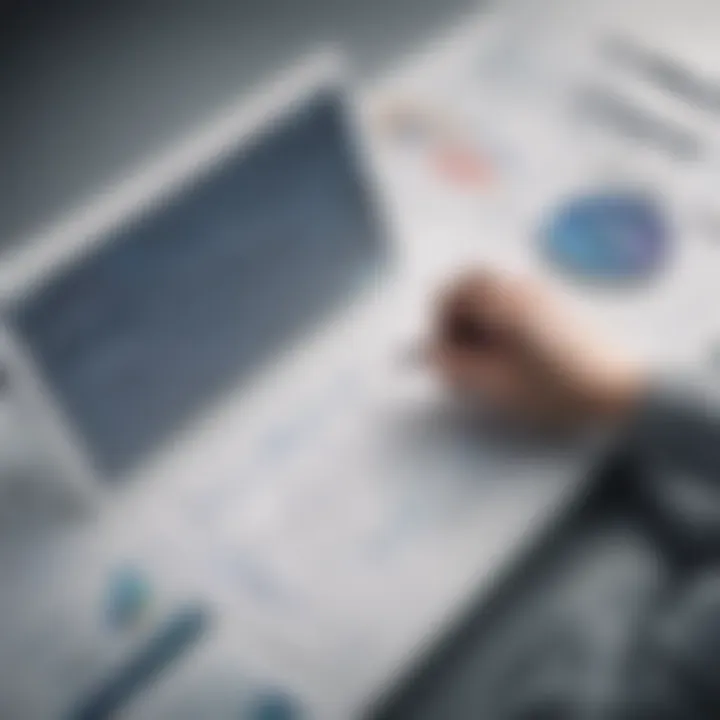
Tracing the evolution of Qlik Predictive Analytics unveils a series of pivotal advancements that have reshaped predictive modeling practices. From basic regression analysis to sophisticated machine learning algorithms, each milestone signifies a milestone in enhancing predictive capabilities and expanding application horizons. The key characteristic of these milestones lies in their adaptability, continually evolving to meet the escalating demands of data analytics in modern business landscapes.
Technological Advancements
The infusion of technological advancements in Qlik Predictive Analytics propels the predictive modeling techniques towards unprecedented efficiency and accuracy. Harnessing the power of cloud computing, artificial intelligence, and big data analytics, these advancements augment the predictive analytics toolkit with enhanced processing capabilities and predictive modeling algorithms. The unique feature of technological advancements lies in their ability to streamline data processing workflows and enhance predictive model performance, paving the way for more insightful and actionable business intelligence.
Benefits of Qlik Predictive Analytics
Qlik Predictive Analytics plays a pivotal role in revolutionizing decision-making processes by offering unparalleled insights into data patterns and trends. Businesses across various sectors are increasingly leveraging the power of predictive analytics to enhance their strategic planning and operational efficiency. The adoption of Qlik Predictive Analytics enables organizations to gain a competitive edge by making informed decisions based on data-driven forecasts and projections, rather than relying on intuition or historical trends. This section will delve into the specific elements that make Qlik Predictive Analytics essential in today's data-driven landscape.
Enhanced Decision-Making
Real-Time Insights
Real-Time Insights provide decision-makers with up-to-the-minute information on key metrics and performance indicators, allowing for swift and well-informed decisions. This aspect of Qlik Predictive Analytics ensures that businesses can adapt promptly to changing market conditions and consumer preferences. The ability to access real-time data enables organizations to fine-tune their strategies in response to immediate feedback, contributing significantly to overall business agility and competitiveness. Despite its undeniable advantages, the constant influx of real-time data poses challenges in terms of data processing speed and resource utilization, which need to be carefully managed for optimal outcomes.
Risk Mitigation Strategies
Risk Mitigation Strategies offered by Qlik Predictive Analytics empower organizations to proactively identify and address potential risks before they escalate. By analyzing historical data trends and patterns, businesses can anticipate risky scenarios and implement preventive measures to safeguard their operations. This strategic approach not only minimizes losses but also enhances overall risk management frameworks. However, the implementation of risk mitigation strategies requires a nuanced understanding of various risk factors and a robust analytical framework to ensure effectiveness and relevance in mitigating potential threats and vulnerabilities. Balancing risk mitigation with innovation and growth remains a continual challenge for organizations seeking to optimize their risk management practices.
Operational Efficiency
Process Optimization
Process Optimization through Qlik Predictive Analytics helps streamline workflows and enhance operational efficiency by identifying bottlenecks, redundancies, and inefficiencies within existing processes. By optimizing workflows based on data-driven insights, organizations can improve productivity, reduce operational costs, and enhance overall business performance. The systematic analysis of operational processes enables businesses to implement targeted improvements that align with strategic goals and maximize operational effectiveness. However, the successful optimization of processes necessitates a comprehensive understanding of organizational workflows, stakeholder collaboration, and a commitment to continuous process enhancement to sustain operational efficiency gains over time.
Cost Reduction
Cost Reduction is a key focus area for organizations leveraging Qlik Predictive Analytics to streamline their cost structures and drive financial sustainability. By identifying cost-saving opportunities, analyzing expenditure patterns, and optimizing resource allocation, businesses can achieve significant cost reductions while maintaining operational excellence. The implementation of cost reduction strategies requires a diligent approach to cost analysis, budget planning, and performance monitoring to ensure cost-saving measures do not compromise quality, innovation, or long-term growth initiatives. Striking a balance between cost-saving measures and value creation remains a critical consideration for businesses aiming to optimize their cost management practices.
Improved Customer Engagement
Personalized Experiences
Personalized Experiences facilitated by Qlik Predictive Analytics enable businesses to customize their interactions with customers based on individual preferences, behaviors, and demographics. By utilizing predictive analytics to segment customer data and personalize marketing messages, businesses can enhance customer satisfaction, loyalty, and lifetime value. The delivery of personalized experiences necessitates a deep understanding of customer preferences, effective data segmentation strategies, and the utilization of advanced analytics tools to tailor offerings to specific customer segments. While personalized experiences can drive customer engagement and brand loyalty, organizations must navigate data privacy regulations and ethical considerations to uphold customer trust and loyalty.
Targeted Marketing Campaigns
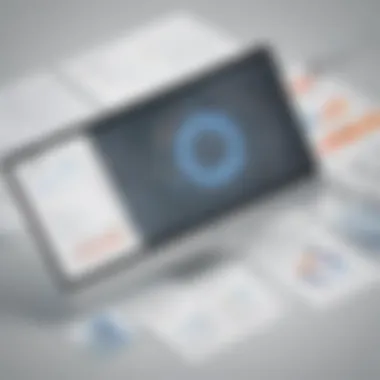
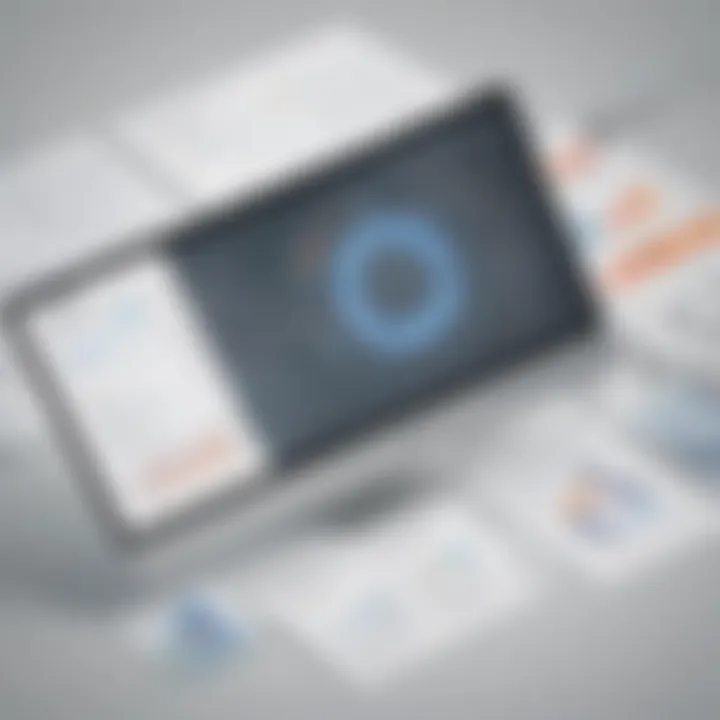
Targeted Marketing Campaigns leverage predictive analytics to identify high-potential customer segments, optimize marketing ROI, and enhance campaign effectiveness. By analyzing consumer behavior patterns, purchase history, and engagement metrics, businesses can tailor marketing strategies to resonate with specific target audiences and improve conversion rates. The efficacy of targeted marketing campaigns relies on accurate data insights, agile campaign management, and continuous performance tracking to refine strategies and ensure desired marketing outcomes. While targeted marketing campaigns can yield impressive results in terms of customer acquisition and retention, striking a balance between personalized marketing and privacy compliance is essential to build sustainable customer relationships.
Applications of Qlik Predictive Analytics
In this section, we explore the significance of Applications of Qlik Predictive Analytics within the broader context of data-driven decision-making. Qlik Predictive Analytics plays a pivotal role in empowering businesses to make informed choices by leveraging predictive insights derived from data analysis. By utilizing advanced algorithms and machine learning techniques, organizations can forecast trends, identify patterns, and optimize operations across various sectors. The ability to analyze historical and real-time data enables companies to streamline processes, enhance efficiency, and gain a competitive edge in the market.
Finance and Banking Sector
Fraud Detection
Fraud Detection stands out as a critical component of Qlik Predictive Analytics, particularly in the finance and banking industry. With the surge in financial crimes and the need to safeguard sensitive information, fraud detection has become increasingly essential for organizations to maintain trust and security. Qlik's predictive capabilities enable financial institutions to detect anomalous activities, flag suspicious transactions, and prevent fraudulent behavior effectively. The adaptive nature of Qlik Predictive Analytics enhances fraud detection accuracy, reducing false positives and minimizing risks associated with fraudulent activities.
Credit Risk Assessment
Credit Risk Assessment is another vital aspect of Qlik Predictive Analytics in the finance and banking sector. By utilizing predictive models and historical data analysis, financial institutions can evaluate the creditworthiness of borrowers, assess potential risks, and make informed lending decisions. Qlik's predictive algorithms provide valuable insights into customers' credit profiles, repayment behaviors, and financial stability, allowing banks to mitigate credit risks effectively. The integration of Qlik Predictive Analytics in credit risk assessment processes enhances decision-making, minimizes defaults, and optimizes loan portfolio management.
Healthcare Industry
Patient Outcome Prediction
Within the healthcare sector, Patient Outcome Prediction serves as a transformative application of Qlik Predictive Analytics. Healthcare providers leverage predictive analytics to forecast patient outcomes, identify high-risk individuals, and personalize treatment plans for better care delivery. By analyzing patient data, medical histories, and treatment responses, healthcare professionals can anticipate disease progression, allocate resources efficiently, and improve patient outcomes significantly. Qlik's predictive capabilities enable healthcare organizations to enhance medical decision-making, optimize resource utilization, and deliver personalized healthcare services.
Disease Diagnosis
Disease Diagnosis is another critical use case of Qlik Predictive Analytics in the healthcare industry. By leveraging predictive models and clinical data, medical practitioners can expedite the diagnostic process, improve accuracy, and provide proactive healthcare interventions. Qlik's advanced analytics tools facilitate early detection of diseases, risk assessment, and treatment planning, leading to timely interventions and better patient outcomes. The integration of Qlik Predictive Analytics in disease diagnosis enhances diagnostic accuracy, reduces misdiagnosis rates, and ultimately improves patient care.
Retail and E-Commerce
Inventory Management
In the realm of retail and e-commerce, Inventory Management emerges as a strategic application of Qlik Predictive Analytics. Businesses utilize predictive analytics to optimize inventory levels, forecast demand, and prevent stockouts or overstocking scenarios. By analyzing sales data, seasonal trends, and customer behavior, companies can enhance inventory planning, reduce carrying costs, and maximize operational efficiency. Qlik's predictive models enable retailers to implement agile inventory management strategies, improve supply chain responsiveness, and enhance customer satisfaction through timely product availability.
Sales Forecasting
Sales Forecasting represents a crucial aspect of Qlik Predictive Analytics for retail and e-commerce enterprises. By leveraging predictive analytics, businesses can predict sales trends, identify growth opportunities, and optimize marketing strategies to drive revenue generation. Qlik's predictive algorithms help retailers analyze market dynamics, customer preferences, and external factors influencing sales performance effectively. The integration of Qlik Predictive Analytics in sales forecasting processes empowers businesses to make data-driven decisions, capitalize on market trends, and enhance sales performance through targeted promotional campaigns.
Challenges and Considerations in Implementing Qlik Predictive Analytics
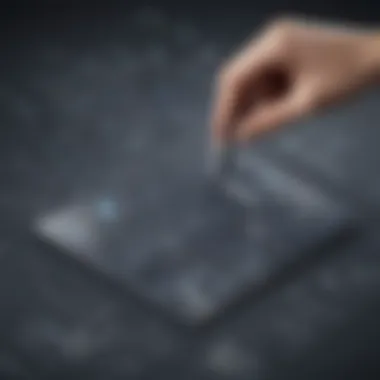
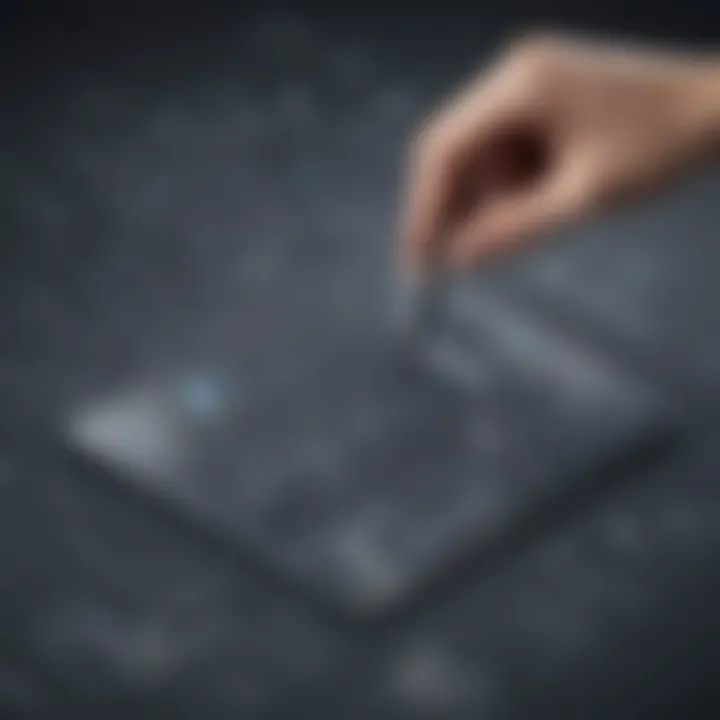
When delving into the realm of Qlik Predictive Analytics, it's crucial to consider the challenges and considerations that come with its implementation. These aspects play a pivotal role in determining the success and effectiveness of utilizing predictive analytics within an organization's data ecosystem. By addressing these challenges proactively, businesses can optimize their predictive analytics strategies and ensure that they yield actionable insights for informed decision-making processes. Whether it's navigating data quality concerns or allocating resources efficiently, understanding and mitigating these challenges is essential for harnessing the full power of Qlik Predictive Analytics.
Data Quality and Integration
Data Cleansing
Data cleansing stands out as a critical component in the realm of utilizing predictive analytics effectively. This process involves identifying and rectifying errors or inconsistencies in the data, ensuring that the information used for predictive modeling is accurate and reliable. By cleansing the data, organizations can enhance the quality of their analytics outputs, leading to more precise insights and informed decision-making. The meticulous nature of data cleansing not only improves the overall data integrity but also minimizes the risk of misleading conclusions drawn from flawed data sets. While data cleansing requires significant time and effort, its benefits in increasing the reliability of predictive analytics outcomes make it a cornerstone in any data-driven strategy.
Data Governance
Data governance plays a crucial role in ensuring that data assets are managed effectively throughout the predictive analytics lifecycle. This structured approach to data management establishes guidelines and protocols for data handling, accessibility, and security, promoting consistency and accuracy in analytical processes. Implementing robust data governance frameworks helps organizations streamline data integration, maintain compliance with regulatory requirements, and enhance data quality and reliability. While data governance may introduce some level of rigidity in data management practices, its positive impact on data quality and integrity far outweighs any perceived disadvantages. Embracing data governance is essential for organizations looking to leverage Qlik Predictive Analytics seamlessly and effectively.
Resource Allocation
Skills Training
Skills training represents a vital component in the successful implementation of Qlik Predictive Analytics within an organization. Equipping personnel with the necessary skills and knowledge to leverage predictive analytics tools and techniques empowers them to extract meaningful insights from data sets efficiently. By providing comprehensive training programs on data analysis, statistical modeling, and predictive algorithms, businesses can enhance their employees' analytical capabilities and decision-making acumen. The continuous investment in upskilling employees in predictive analytics not only boosts operational efficiency but also fosters a data-driven culture that embraces innovation and experimentation.
Infrastructure Investment
Infrastructure investment plays a pivotal role in laying the foundation for robust predictive analytics capabilities. This includes acquiring the necessary hardware, software, and IT infrastructure to support data processing, modeling, and visualization requirements effectively. Ensuring that the IT infrastructure is scalable, secure, and agile is essential for accommodating the data-intensive nature of predictive analytics workloads. Organizations that prioritize infrastructure investment gain a competitive edge in leveraging Qlik Predictive Analytics to derive actionable insights and drive strategic decision-making. While significant upfront costs may be associated with infrastructure investment, the long-term benefits in terms of improved performance and efficiency make it a strategic investment for sustainable growth and innovation.
Future Trends and Innovations in Qlik Predictive Analytics
In the realm of Qlik Predictive Analytics, the discussion of future trends and innovations holds a paramount significance. As technology continues to evolve rapidly, staying abreast of the latest advancements is crucial for businesses and individuals aiming to maximize the potential of predictive analytics tools. The exploration of future trends in this domain enables organizations to anticipate upcoming developments, adapt their strategies, and gain a competitive edge in the dynamic landscape of data analytics. Addressing the emerging trends and innovations in Qlik Predictive Analytics facilitates informed decision-making, fosters continuous improvement, and drives success in various industries.
AI and Machine Learning Integration
Automated Insights:
The integration of Artificial Intelligence (AI) and Machine Learning (ML) algorithms into Qlik Predictive Analytics tools has revolutionized the generation of automated insights. Automated insights refer to the ability of AI-driven systems to extract meaningful patterns, trends, and forecasts from vast datasets with minimal human intervention. This automation accelerates the decision-making process, enhances efficiency, and provides organizations with timely and accurate predictions for informed actions. The key characteristic of Automated Insights lies in its capacity to sift through massive data sets swiftly, uncover hidden correlations, and deliver actionable intelligence in real-time. This feature proves beneficial for organizations looking to optimize operations, identify opportunities, and mitigate risks effectively.
Predictive Modeling Advancements:
Predictive modeling advancements represent a crucial facet of leveraging AI and ML integration in Qlik Predictive Analytics. These advancements enhance the accuracy, reliability, and scalability of predictive models, enabling organizations to make data-driven decisions with greater confidence. The key characteristic of predictive modeling advancements is the utilization of advanced algorithms and computational techniques to forecast outcomes, detect patterns, and improve predictive performance. This enhanced modeling capability empowers businesses to anticipate trends, optimize processes, and capitalize on emerging opportunities. While the advantages of predictive modeling advancements include enhanced predictive accuracy and streamlined forecasting processes, considerations such as model interpretability and computational complexity require careful evaluation in implementing these advancements.
Predictive Analytics as a Service (PAaaS)
Cloud-Based Solutions:
The adoption of Predictive Analytics as a Service (PAaaS) through cloud-based solutions offers organizations a flexible and scalable approach to accessing predictive analytics capabilities. Cloud-based solutions provide on-demand access to predictive modeling tools, data processing resources, and analytical insights without the need for extensive on-premise infrastructure. The key characteristic of cloud-based solutions in PAaaS is the seamless integration of analytics platforms with cloud computing technologies, facilitating rapid deployment, resource scalability, and cost-effectiveness. This approach proves beneficial for businesses seeking to leverage predictive analytics without heavy initial investments, enabling efficient resource allocation and operational agility.
Scalability and Flexibility:
Scalability and flexibility stand out as essential features of PAaaS, allowing organizations to adjust their predictive analytics resources according to dynamic business requirements. The scalability aspect enables seamless expansion or contraction of computational resources based on changing data volumes, analytical needs, or organizational growth. The key characteristic of scalability and flexibility is the ability to scale predictive analytics operations up or down swiftly, ensuring optimal resource utilization and performance efficiency. While the advantages of scalability and flexibility include improved operational agility and cost optimization, factors like data privacy and security concerns necessitate careful planning and management in deploying these features in predictive analytics platforms.