Mastering the Stages of Data Analysis Process
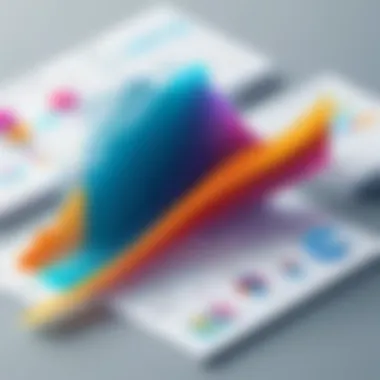
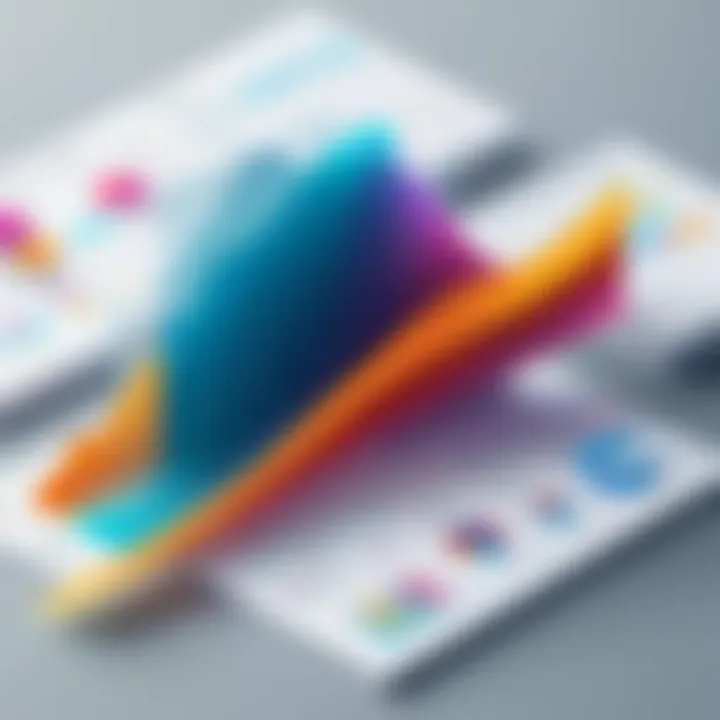
Intro
In today's data-driven world, the ability to effectively analyze data is invaluable. Recognizing patterns, trends, and insights hidden within vast amounts of information is akin to finding a needle in a haystack. This guide aims to walk you through the intricate labyrinth of the data analysis process.
Data analysis isn’t merely about crunching numbers or generating graphs. It encompasses a series of steps that transform raw data into meaningful conclusions. Whether you are a seasoned IT professional or a small business owner venturing into analytics for the first time, understanding each phase will be crucial. We will dissect everything from clearly defining the problem at hand to the final stage of reporting findings.
Moreover, the tools and methodologies utilized are fundamental to the success of any data project. With so many options available in the marketplace, it can often leave folks feeling overwhelmed. Therefore, we will delve into various techniques and software that cater to a myriad of needs and industries. This comprehensive guide is crafted to equip you with the knowledge needed to navigate the complexities of the data landscape with confidence.
By the end, you’ll not only know the processes involved but also how to apply them practically, ensuring your data analysis journey is not just an academic exercise but a real-world application. Let’s roll up our sleeves and embark on this exploration of unlocking the power of data analysis!
Prelude to Data Analysis
Data analysis is the bedrock of decision-making in today’s data-driven world. As businesses and organizations churn out massive amounts of data daily, understanding the data analysis process is no longer a luxury but a necessity. It’s akin to steering a ship with a compass—without it, you're bound to drift aimlessly. This section introduces the essential facets of data analysis, shedding light on its definition and importance.
Defining Data Analysis
At its core, data analysis refers to the systematic approach to interpreting, processing, and deriving insights from raw information. It’s not just about collecting heaps of data and calling it a day; it involves piecing together the puzzle—transforming unrefined information into actionable intelligence. Whether you're tracking sales performance metrics or evaluating customer feedback, the goal of data analysis remains the same: to extract meaningful conclusions that can guide strategic planning.
In simpler terms, think of it like cooking. You start with raw ingredients, and through careful preparation and mixing, you end up with a delicious dish. Similarly, in data analysis, you start with raw data, and through methods such as filtering, aggregation, and transformation, you create insights that can inform business decisions.
Importance of Data Analysis
Understanding the significance of data analysis is paramount for IT professionals, software developers, and businesses of all sizes. The benefits are manifold:
- Informed Decision-Making: Data analysis allows businesses to base their strategies on real evidence rather than hunches. For instance, through analyzing customer purchase patterns, retailers can optimize inventory.
- Identifying Trends: By revealing patterns and trends, data analysis helps businesses anticipate changes in the market. This foresight can make or break a company in today’s fast-paced environment.
- Risk Management: Identifying potential risks before they occur is crucial. An effective data analysis technique can highlight anomalies or trends that might signal upcoming challenges.
- Enhanced Efficiency: Efficiently managing resources becomes possible when decisions are based on data-driven insights. For example, through analyzing staff performance data, a company can determine if they need to adjust workforce allocations.
"In an age where data is ubiquitous, the ability to analyze it separates successful organizations from those that merely exist."
The Data Analysis Process Overview
The data analysis process is a meticulous journey that transforms raw data into meaningful insights. Understanding this process is paramount for IT professionals, software experts, and businesses, whether large or small. Not only does it provide clarity, but it also equips stakeholders with the tools to make data-driven decisions.
Stages of Data Analysis
Data analysis is rarely a straightforward path. Each stage plays its own role while intertwining with the others. The stages include problem definition, data collection, preparation, analysis, interpretation, reporting findings, and implementing decisions. Here’s a brief rundown of each stage:
- Problem Definition: The first step before diving headfirst into data is to identify specific objectives. What do you want to achieve? This isn't about fishing in murky waters but casting a line with a clear target in mind.
- Data Collection: Gathering the right data requires knowing the type you need—whether it’s quantitative, qualitative, primary, or secondary. This is where surveys, interviews, and existing data sources come into play, each method offering its own strengths and weaknesses.
- Data Preparation: Data isn’t always clean and shiny. Cleaning involves handling missing values and removing duplicates, making sure you're working with accurate information. Data transformation, such as normalization or standardization, follows to ensure consistency for later analysis.
- Data Analysis Techniques: This is where you use statistical tools and models to draw insights. Descriptive statistics paint a picture of the data, while inferential statistics helps to make generalizations. Predictive modeling takes a step further, forecasting future trends based on historical data.
- Interpretation of Results: Here’s where the rubber meets the road. Understanding insights gained and identifying trends can help frame effective decisions. It's like piecing together a puzzle where every insight contributes to the bigger picture.
- Reporting Findings: Crafting effective reports with a clear narrative is essential for communicating results. Use charts, graphs, and dashboards to visualize data—these tools can effectively bring insights to life.
- Making Decisions Based on Data: The ultimate goal is to translate insights into action. Data should influence strategies and operations, allowing for informed decisions that lead to tangible benefits.
- Continuous Improvement: Data analysis doesn’t stop after one cycle. Establishing feedback loops allows for constant refinement, making the process iterative, where each cycle builds on the last.
Understanding these stages isn't just about knowing what goes where; it’s about appreciating how they connect, like cogs in a machine. The interdependence of these stages can often reveal the beauty of data—it's not just numbers, it’s a story waiting to be told.
Common Challenges in Data Analysis
Confronting data analysis comes with its unique set of hurdles. Recognizing these challenges is crucial for successful navigation of the process. Some of them include:
- Data Quality Issues: Poor data can lead to misguided conclusions. Missing values, inaccuracies, or bias in data can skew results.
- Complexity of Tools: With an array of software and techniques, choosing the right tools can be overwhelming. Not every tool fits every task; understanding which to use can save a lot of headaches.
- Resistance to Change: Sometimes, the insights derived from data don’t align with existing beliefs or practices. Getting buy-in from stakeholders can be a challenge in these cases.
- Time Constraints: In our fast-paced world, the demand for quick results often clashes with the thoroughness required in data analysis. Balancing speed with depth can make or break a project.
- Data Security Concerns: With increasing regulations around data protection, ensuring compliance while conducting analysis can complicate matters.
"The road to effective data analysis is often marred by challenges, yet each obstacle is an opportunity for growth and innovation."
Navigating these challenges requires a strategic mindset and a toolkit equipped with knowledge and resources. By recognizing and preparing for these common pitfalls, professionals can foster resilience through the analysis process and harness the true power of data.
Step One: Problem Definition
Defining the problem is, without a doubt, the linchpin of the entire data analysis process. It lays the groundwork for every subsequent step, guiding analysts through the maze of data, ensuring the journey is not just aimless wandering but a methodical exploration aimed at extracting valuable insights. If the problem isn't clearly defined, you're just throwing darts in the dark, hoping to hit a bullseye but likely hitting the wall instead.
Identifying Objectives
To get the ball rolling, one must first identify the objectives. This isn't just about scribbling down vague ideas or wishful thinking; it’s about pinpointing what you want to achieve. For instance, a retail company may want to understand why product sales dipped last quarter. Rather than blathering about sales numbers, they should be asking deeper questions. Is it due to pricing, quality, or perhaps some external factors like competition or changing consumer behavior? Clear objectives help sharpen focus and set the direction for the analysis.
Consider these crucial pointers when identifying objectives:
- Specificity is Key: Clearly state objectives that can be understood without ambiguity.
- Measurable Outcomes: Decide how you will measure success. It’s not about just wanting to increase sales; it’s about how much of an increase you aim for.
- Realistically Achievable: Ensure the objectives set are reachable given your resources and data availability.
"A goal without a plan is just a wish."
This adage resonates profoundly in data analysis. Without a defined roadmap, even the best efforts might yield little more than frustrating roadblocks.
Articulating Research Questions
Once objectives are laid out, it’s time to dive into articulating research questions. These questions serve as the guiding star, helping analysts navigate through the data landscape effectively. Each question should be crafted to drive the analysis forward, ensuring that they address the objectives identified earlier.
For example, if the objective was to boost customer retention, the research questions might include:
- What do our loyal customers value most about our products?
- What factors led to the loss of customers in the last six months?
- How does our customer service feedback correlate with customer retention rates?
The key here is to craft questions that are clear and focused. Vague questions risk leading to irrelevant data analysis without proper context.
Some practical tips for articulating effective research questions include:
- Keep it Simple: Avoid jargon or complex phrasing to ensure clarity.
- Focus on Actionable Insight: Questions should help uncover information that leads to decisions.
- Iterative Process: Don’t hesitate to refine your questions as you gather initial findings. Sometimes, insights can change the scope of inquiry.
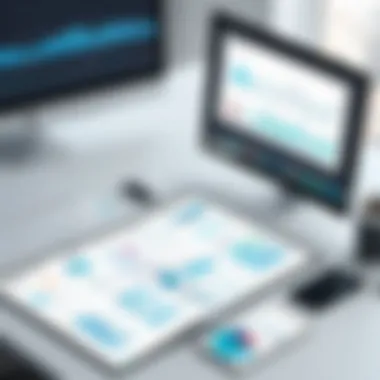
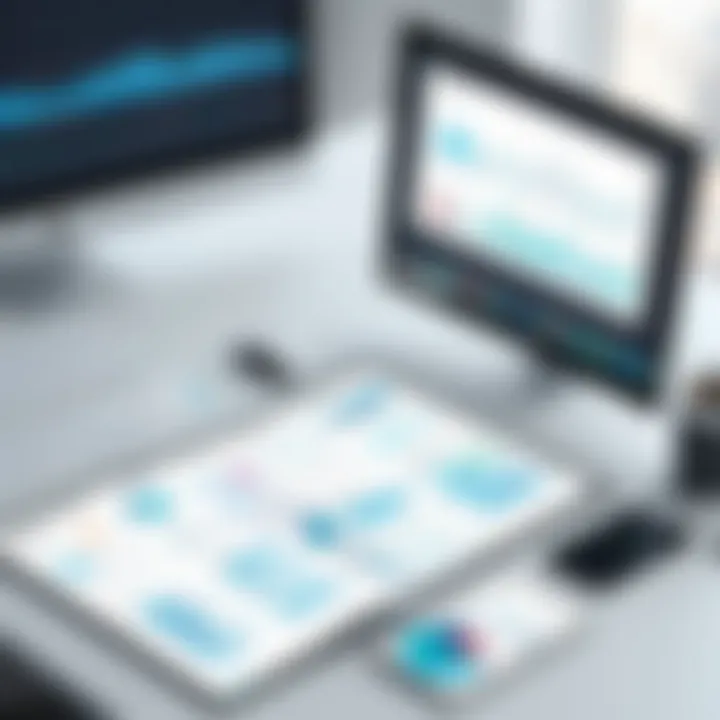
By establishing your objectives and clarifying research questions, the groundwork is set. It’s like setting sail on a ship; without a clear destination, crew members may get seasick and deter from the journey altogether, but a well-defined course keeps everyone on track. These foundational steps prepare the path ahead, making the entire data analysis process more streamlined and effective.
Step Two: Data Collection
Data collection is a pivotal phase within the overall data analysis process. It's where the raw materials for any analytical endeavor are gathered, setting the stage for all subsequent actions. Good data collection determines the integrity and reliability of the analysis. Without proper data, any insights drawn may resemble a house built on a shaky foundation, susceptible to collapse.
The effectiveness of the data collection stage lays the groundwork for achieving accurate outcomes. In this step, considerations such as the types of data being collected and the methods employed come into play.
Types of Data
Understanding the types of data is essential, as it influences how analysis will unfold.
Quantitative vs. Qualitative
The difference between quantitative and qualitative data is significant in the realm of data analysis. Quantitative data refers to information that can be quantified, often in numerical form. For example, sales figures or customer counts are classic cases of quantitative data. This type of data is popular for its ability to convey trends and patterns in a straightforward way. Its key characteristic is its measurability, making it an effective tool for statistical analyses. However, while quantitative data can tell you how many or how much, it often lacks depth in understanding the why behind the numbers.
On the other hand, qualitative data captures the nuances of human experience. Think of focus groups or open-ended survey responses—these provide insight into feelings, thoughts, and motivations. The unique feature of qualitative data is its richness, fostering deeper understanding of the context and complexities of certain phenomena. However, it can be more challenging to analyze and quantify, introducing a layer of complexity that must be dealt with during the analysis.
Primary vs. Secondary
The distinction between primary and secondary data also plays a critical role in the collection phase. Primary data is collected firsthand for a specific analysis purpose. Surveys and experiments, for example, fall under this category. The key characteristic of primary data is its originality; it caters directly to the analysis needs. It holds up well because it specifically addresses the research questions at hand. However, collecting primary data can be time-consuming and costly.
In contrast, secondary data refers to information that has already been collected, often for different purposes. This could be existing studies, reports, or datasets from previous research. The benefit of secondary data lies in its availability and cost-effectiveness; you don’t have to start from scratch. Yet, it can be problematic if the data isn't aligned with current research needs or if it carries biases from the original collection.
Data Collection Methods
The methods utilized for data collection are equally critical, influencing both the depth and breadth of collected information.
Surveys
Surveys are a highly popular method for data collection. They range from simple questionnaires to intricate forms tailored to gather specific data points. A key characteristic of surveys is their scalability; they can effectively gather data from a large audience. Their unique feature is that they can provide both quantitative and qualitative data when structured properly. However, poorly designed surveys may lead to bias or misinterpretation of responses, which can skew the analysis.
Interviews
Interviews are another valuable method used in data collection. They allow for direct interaction, which can yield insightful qualitative data through conversation. The benefit of interviews is that they enable deeper probing into responses, helping to unearth perspectives that are often overlooked in other methodologies. However, they can be time-consuming and may not always be easily generalizable to larger populations.
Observations
Observations involve systematically recording behavior as it occurs. This method allows analysts to gather data in real-time and context, avoiding potential biases intrinsic in self-reported data. A key characteristic of observations is their ability to capture details that might not come across in surveys or interviews. However, they can be biased by the observer’s own perspective and may not cover all relevant variables.
Existing Data Sources
Finally, tapping into existing data sources can be an excellent way to gain insights without the effort of gathering data from scratch. This method often encompasses a combination of secondary data from studies and databases. The key characteristic here is accessibility; existing data can allow for quick analysis. However, one must handle this data with caution, ensuring that it's relevant and accurate for current analysis needs.
"The right data collection method can be the difference between insight and confusion. Choose wisely!"
Step Three: Data Preparation
Data preparation is a critical phase in the data analysis journey. It's like laying the groundwork before building a house. You wouldn’t want to put up walls on shaky foundations, right? Without proper data preparation, your analysis could end up being skewed or fundamentally flawed. This step essentially cleans, organizes, and transforms data into a format that is suitable for analysis, leading to valuable insights and accurate conclusions.
Cleaning the Data
Data cleaning is the first hurdle in data preparation, and it can be quite a task. If you think about it, raw data is like an unedited manuscript—comforting to gather, but messy and hard to read. When it comes to data analysis, the presence of errors, duplicates, or missing values can severely distort findings.
Handling Missing Values
Handling missing values is about deciding how to treat those pesky gaps in your dataset. Missingness can arise due to various reasons such as non-responses in surveys or data collection errors. The main characteristic of handling missing values is to maintain the integrity of your analysis. It’s a popular choice because it directly impacts the dataset's reliability.
To tackle missing values, you can do several things. For instance, you might choose to delete records with missing data, which is straightforward but can lead to loss of valuable information. Alternatively, techniques like imputation — replacing missing values with mean or median values — could be utilized to retain data integrity. However, be cautious: over-reliance on imputation can mask underlying trends, leading to inaccurate conclusions.
Removing Duplicates
Removing duplicates is all about ensuring that each piece of data is unique. Duplicates can muddle your analysis by making patterns seem more significant than they really are. By eliminating redundancy, you enhance the quality of your dataset. This practice is beneficial because it streamlines the analysis, leading to clearer results.
One unique aspect of removing duplicates is that it also aids in identifying potential data errors. For example, if twenty entries for the same individual pop up, you might have a data input issue that needs addressing. However, on the flip side, careful consideration is required. In some cases, duplicate entries can be valid—for instance, if multiple transactions from the same user provide valuable insights in a business context.
Data Transformation
Data transformation is where the magic truly happens. Think of it as translating a book into a different language to make it more accessible. This step is vital for shaping the data into formats suitable for analysis.
Normalization
Normalization is a technique that adjusts the scale of your data. It’s used primarily when you're working with different units or scales and want to make them comparable. This characteristic of normalization helps in scenarios where relationships in data might be distorted due to varying ranges.
It’s a beneficial choice often employed in machine learning to ensure that the algorithms function properly. A unique feature of normalization is that it compresses data into a common range. Still, it's important to note that it might not always be suited for categorical variables or data where relationships should remain intact. This can lead to loss of information regarding those relationships, which may not always be ideal.
Standardization
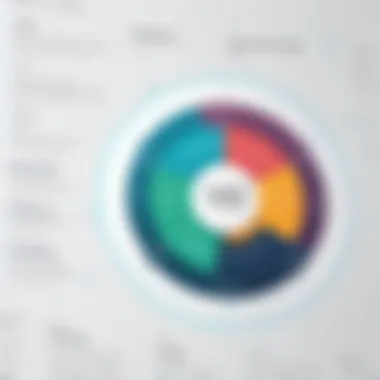
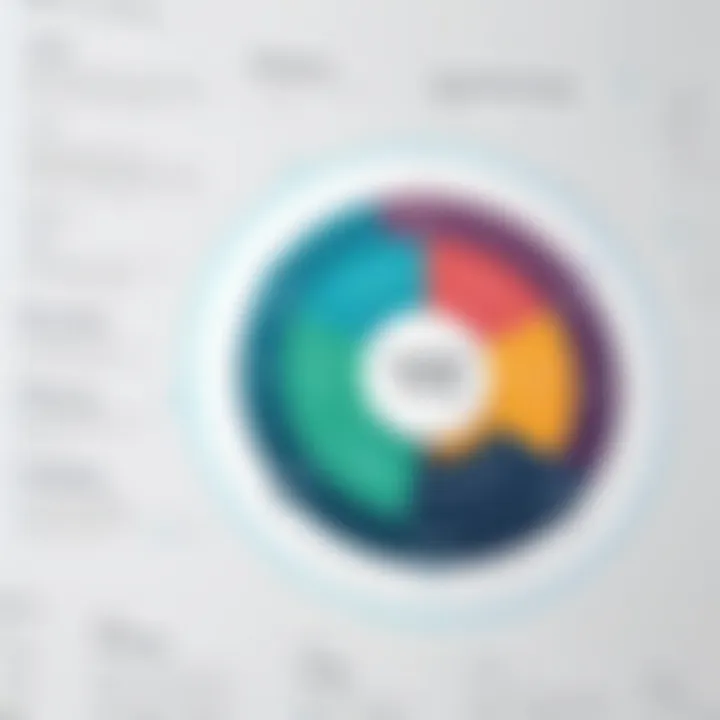
Standardization, on the other hand, is about converting your data to a standard scale—mean of zero and a standard deviation of one. This becomes crucial when analyzing data with different distributions. It helps in aligning data and ensuring that outliers are not unduly influential. People favor standardization as it enables various algorithms, especially in statistics and machine learning, to perform better by treating all features equally.
One unique feature of standardization is its applicability to both normally distributed data and even non-normally distributed cases, where trying to normalize could distort their structure. Though it boasts numerous advantages, it might faze some users at first, especially those unaccustomed to thinking in terms of z-scores and bell curves.
In summary, Step Three in the data analysis process not only clarifies defects within your dataset but also transforms it into a foundation upon which thorough and accurate analyses can be performed. The strength of your analysis largely depends on how effectively you handle these preparation tasks.
Step Four: Data Analysis Techniques
Data analysis techniques are a crucial part of understanding and interpreting the information gleaned from datasets. When diving into data analysis, it’s like peeling an onion. Each layer reveals more insights, but it can also lead to tears if one is not prepared. Various techniques exist, each suited to different types of analysis and objectives. By mastering these techniques, IT professionals, software specialists, and businesses can extract profound meaning and actionable insights from raw data.
Descriptive Statistics
Descriptive statistics serve as the bedrock of data analysis. Imagine trying to describe a vibrant painting using only black and white—this is what data analysis would be like without descriptive statistics. These techniques summarize and organize the characteristics of your dataset, giving you a clearer picture of what you're working with.
- Key Elements: Descriptive statistics include measures such as mean, median, mode, standard deviation, and range. These elements portray data distributions comprehensively.
- Benefits: It simplifies large amounts of data into understandable figures, allowing for quick comprehension. When businesses want a snapshot of their performance—such as average sales for the quarter—descriptive statistics deliver precisely that, thus permitting swift decisions based on straightforward insights.
- Considerations: However, relying solely on descriptive statistics can be misleading. They don’t provide insights into the relationships between variables, and statistical outliers can skew the results significantly.
Inferential Statistics
Inferential statistics kick things up a notch. Instead of merely describing the data, inferential methods allow analysts to make predictions or generalizations about a larger population based on a sample. Think of it as a game of poker; you base your bets on what you know but also on what you infer from your opponents.
- Key Elements: Techniques within this realm include hypothesis testing, confidence intervals, and regression analysis. These allow you to draw broader conclusions from a specific dataset.
- Benefits: This approach makes it possible to ascertain relationships between variables and determine cause-and-effect, making it a powerful tool for IT and business strategists. For instance, businesses can project future trends using past sales data, informing decisions such as stock purchases or marketing strategies.
- Considerations: Yet, the reliability of inferential statistics depends on the representativeness of the sample. If the sample is biased, the conclusions drawn may lead you astray, like relying on a gnarled compass.
Predictive Modeling
Predictive modeling takes statistical analysis into the future. It’s akin to trying to predict the weather based on historical data; the more accurate your previous data is, the better your predictions will be. Predictive techniques utilize past data to forecast future outcomes, which can be a game-changer for businesses and IT professionals alike.
- Key Elements: Common models include decision trees, regression models, and neural networks. Each of these aims to find patterns that provide insights about what might happen next.
- Benefits: The ability to predict outcomes enables organizations to proactively address issues, optimize operations, or enhance customer experiences. For instance, a retail business can anticipate peak shopping periods, thus ensuring adequate inventory and staffing.
- Considerations: However, predictive models require meticulous tuning and validation to ensure accuracy. Erroneous assumptions or flawed input data can skew results, leading businesses down the garden path.
Step Five: Interpretation of Results
In the data analysis journey, Step Five plays a pivotal role. Once all the calculations and modeling have been carried out, it's time to make sense of it all. This step, interpretation of results, is where raw data transforms into actionable insights. It’s not just about numbers and statistics anymore; it’s about weaving a narrative that describes what the data is really saying.
Understanding Insights
The primary goal of interpreting results is to derive insights that can inform decision-making. Essentially, insights are those valuable pieces of information gained from data analysis that can guide future actions. For instance, a retail company might discover through its customer purchase data that certain products are consistently bought together. This insight could lead to strategic decisions around marketing, promotions, and inventory management.
When approaching the interpretation, several factors come into play:
- Contextual Awareness: Understanding the context from which the data stems is crucial. This requires knowledge about the industry, market trends, and the specific issues at hand.
- Stakeholder Needs: Different stakeholders might look for different insights. Whether it's decision-makers, marketing teams, or operational managers, tailoring your insights to fit their focus can facilitate better decision-making.
- Clarity: Presenting insights clearly and straightforwardly is paramount. Complex jargon may muddle understanding. Clear communication ensures that insights resonate with all audiences, regardless of their data expertise.
Additionally, it’s important to keep an analytical mindset. Engaging with the data deeply allows analysts to ask the right questions, leading to interpretations that are not only accurate but also valuable.
Identifying Trends and Patterns
Recognizing trends and patterns within the analyzed data is a key component of the interpretation phase. Trends indicate a general direction in which something is developing, while patterns are recurring sequences that can suggest underlying behaviors. For instance, in smartphones, data might reveal a trend showing that younger generations prefer more visually appealing interfaces, indicating potential shifts in product design.
To successfully identify these factors, several strategies can be employed:
- Visualizations: Creating visual aids such as line charts, bar graphs, or heat maps can significantly aid in uncovering trends. For example, if sales figures exhibit a significant rise during holiday seasons consistently over the years, a line chart can clarify this upward trend over time.
- Statistical Techniques: Utilizing methods like time series analysis or regression can also help pinpoint trends and understand the strength of relationships between different variables.
- Comparative Analysis: Sometimes, comparing data across different periods or groups reveals patterns that might not be immediately apparent. For example, analyzing customer feedback before and after a product launch can highlight changes in consumer sentiment.
"The ability to interpret data with a keen eye for trends can mean the difference between mere statistics and informed strategy."
Step Six: Reporting Findings
Reporting findings is a critical aspect of the data analysis process. The objective here is not just to present raw data but to convey meaningful insights in a way that resonates with the target audience. Well-structured reports can transform data into actionable knowledge, making it pivotal for decision-making.
Crafting an Effective Report
Creating an effective report involves several key considerations. First and foremost, determine the audience's level of expertise. A report aimed at data scientists may dive deep into statistical methodologies, while one for business executives might focus on high-level insights and implications.
Utilizing a clear structure is essential. Start with an executive summary that highlights the main conclusions and recommendations. Follow this with sections on methodology, key findings, and supporting data. Ensure your writing is concise and jargon-free to maintain engagement.
Also, employing storytelling techniques can enhance the narrative. Weaving data points into a relatable context can make the report more engaging. For example, instead of just reporting a 20% increase in sales, explain what that means in terms of customer satisfaction or market trends.
Visualizing Data
Visual aids can significantly enhance the understanding of complex datasets. This section will discuss three prominent visualization types: Charts, Graphs, and Dashboards.
Charts
Charts are a staple in data visualization. They break down intricate data into digestible visual formats which makes insights easier to understand. A key characteristic of charts is their ability to succinctly represent relationships between variables. For instance, a pie chart can quickly convey percentage distributions within a dataset, making it an efficient choice in many reports.
Nonetheless, while charts are advantageous for summarizing data, one must be cautious about oversimplifying. A chart can sometimes obscure nuances within the data, so providing thorough context is essential.
Graphs
Graphs take a broader approach by illustrating trends over time or comparisons among different variables. A line graph, for instance, can showcase growth trajectories that are easy to interpret. The strength of graphs lies in their flexibility; they can convey a plethora of information while maintaining clarity.
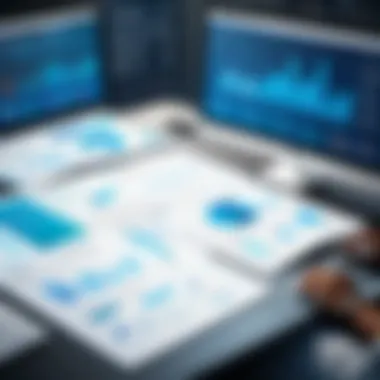
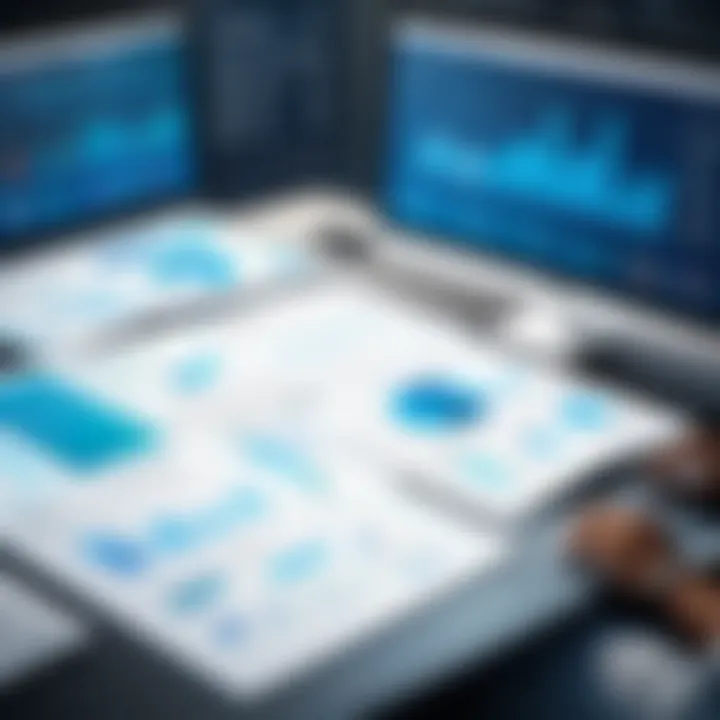
However, it’s important to note that graphs can become cluttered with too much data, potentially leading to confusion instead of clarity. Keeping them simple is oftentimes best, focusing on the most pertinent information.
Dashboards
Dashboards are a comprehensive tool that aggregates key metrics and visualizations in one place. They're especially useful in business environments where real-time decision-making is necessary. A dashboard’s key characteristic is its interactivity; users can often drill down into specific data points for deeper insights.
The major advantage of dashboards is that they provide a holistic view of data performance in a concise manner. However, a downside is the technological barrier; not all stakeholders may be comfortable navigating complex dashboards. Offering training and support can mitigate this issue.
"Data can tell us a story, but it is up to us to narrate it effectively through reporting and visualizations."
Step Seven: Making Decisions Based on Data
Making decisions based on data is a vital step in the data analysis process. At this stage, practitioners distill insights from the data, turning figures and trends into actionable steps. The importance of this step cannot be overstated. It reflects a transition from mere analysis to influencing actual outcomes—be it in business, healthcare, or any other field. Good data-driven decisions have the potential to optimize performance, reduce costs, and ultimately enhance productivity.
Data helps shed light on what works and what doesn’t. It’s not just about generating numbers or statistics; it’s about equipping yourself with knowledge that can steer your direction. Businesses can better allocate resources, tailor products to meet customer needs, and enhance their strategies through informed decision-making.
"In God we trust; all others bring data." – W. Edwards Deming
Translating Data into Action
The translation of data insights into actions involves a nuanced understanding of both the data and the operational environment. First off, this means prioritizing the insights that most align with your strategic goals. There’s no point in acting on every small trend if it doesn’t move the needle significantly.
When deciding on actions:
- Identify Key Insights: Look for patterns that stand out. This could be a sudden spike in customer complaints or an uptick in sales for a specific product line.
- Prioritize Actions: Not all insights are equally important. Focus on those that have align with business objectives or could potentially yield high returns.
- Communicate Clearly: Make sure everyone involved understands the decisions being made and the reasoning behind them. Clear communication fosters alignment and drives action.
Evaluating Outcomes
Once actions based on data have been implemented, the next step is the evaluation of outcomes. This is essential because it creates a feedback loop that informs future decisions. Assessment should not be a one-off task; rather, it should be an ongoing process where results are continually monitored and analyzed.
Effective evaluation considers:
- Set Clear Metrics: Determine what success looks like upfront. Whether it's increased sales, improved efficiency, or higher customer satisfaction, having defined metrics will guide your evaluation.
- Use Comparison: Compare outcomes against the baseline or the expected results. This can help understand whether the changes made had the desired effect.
- Gather Comprehensive Feedback: Assess not just the quantitative metrics but also qualitative feedback. Stakeholder input can provide invaluable context that raw data might miss.
Step Eight: Continuous Improvement
In the intricate realm of data analysis, continuous improvement stands as a cornerstone. This is not just a buzzword floating around in corporate jargon; it embodies a pursuit to refine and enhance methodologies, tools, and outcomes. By embedding this principle into the workflow of data analysis, organizations can ensure they are on a pathway to maximizing effectiveness and efficiency.
The essence of continuous improvement revolves around the understanding that no process is ever static. The landscape of data analytics is perpetually evolving, driven by advancements in technology, shifting market dynamics, and emerging analytical techniques. Thus, businesses must cultivate a mindset that embraces change and adaptation. Here are some specific elements that underline the importance of continuous improvement:
- Enhancing Data Quality: By regularly assessing and refining data collection methods, businesses can increase the accuracy and relevancy of their datasets. This, in turn, leads to more informed decision-making.
- Reducing Waste: Continuous improvement methodologies, such as Lean and Six Sigma, can help in identifying steps in the analytical process that do not add value. Streamlining these processes reduces time, effort, and resources wasted on unnecessary tasks.
- Responding to Feedback: Leveraging feedback from all levels can propel a culture of innovation and responsiveness within teams, ultimately leading to better analytical outcomes.
"Continuous improvement is better than delayed perfection."
— Mark Twain
Feedback Loops
Feedback loops are integral to any continuous improvement strategy in data analysis. These loops create a powerful mechanism for organizations to harness insights gained from previous analyses. Implementing feedback loops encourages teams to regularly solicit input from stakeholders and end-users regarding data usability, visualizations, and overall analytical outputs.
To establish effective feedback loops, consider the following:
- Regular Check-Ins: Schedule periodic reviews of analytical outcomes with relevant stakeholders to discuss what worked, what didn't, and why.
- Using Metrics: Evaluate performance through predefined metrics, such as accuracy rates, response times, and user satisfaction. This quantitative data can help surface issues that need addressing.
- Surveys and Interviews: Utilizing both quantitative and qualitative feedback collection methods will provide a holistic view of stakeholder experiences.
By creating a culture where feedback is actively sought and valued, organizations not only foster continuous improvement but also create resilient data teams that thrive on collaboration and learning.
Iterative Processes in Data Analysis
Iterative processes form the backbone of continuous improvement in data analysis. Unlike a one-and-done approach, an iterative framework allows teams to refine their analyses through multiple cycles of development and evaluation. The iterative process can be likened to a sculptor chiseling away at a block of marble; each pass refines the end result.
The key benefits of utilizing iterative processes include:
- Adaptability: As new data emerges or market conditions change, teams can adapt their analyses swiftly, harnessing the latest insights.
- Incremental Improvement: Each iteration builds upon the last, ensuring a cumulative increase in the quality and impact of the data analysis.
- Engagement with Stakeholders: Iterative cycles often involve client or stakeholder feedback at various stages, ensuring that the outcomes align with their needs and expectations.
The End
In wrapping up, it's essential to grasp the significance of the conclusion in the realm of data analysis. This final section ties together the various threads woven throughout the article. It offers a moment to reflect on what we've learned, reinforcing not just the importance of mastering data analysis skills, but also how they can fundamentally change the way we understand and interpret vast amounts of information.
The Evolving Landscape of Data Analysis
Over time, data analysis has changed quite a bit. When we think about the old days, data analysis often relied heavily on static tools and methods. Today, that's hardly the case. The tools at our disposal have evolved incredibly. Cloud computing, for instance, has made it easier for businesses of all sizes to access and analyze data. Whether it's about integrating Artificial Intelligence, Machine Learning, or even more straightforward statistical tools, the landscape is wide and varied.
Consider this: companies that embrace these advancements can turn data into actionable insights much quicker than their competitors. This adaptability is not just an option—it's a necessity.
Moreover, understanding the evolving landscape helps in avoiding pitfalls that can come from sticking with outdated practices. Data's nature is changing, with larger volumes pouring in at a faster pace. This necessitates staying updated, not just with tools but also with methodologies.
Becoming adept at navigating these changes lays the groundwork for a more data-driven decision-making process. Companies, and professionals alike, are recognizing that in an era where data is king, the ones who can analyze it effectively will hold the upper hand.
Future Directions for Data Analysis
Looking ahead, we face exciting prospects in the world of data analysis. As technology continues to advance, so will the methods and tools used for analysis. One significant area worth keeping an eye on is automation. With the implementation of automated data analysis tools, mundane tasks will take less time, allowing analysts to focus on deeper analysis and interpretation.
Furthermore, the integration of ethical considerations in data analytics is becoming more prominent. Organizations are starting to realize that how they gather, store, and analyze data must align with ethical standards and regulations. Ensuring transparency and data privacy will likely become a crucial part of the future data analysis dialogue.
Also, consider the role of collaboration. The future of data analysis isn’t just about working in silos. Collaboration across departments and even among companies could lead to richer insights. In an increasingly connected world, the collective intelligence may pave the way for more profound understanding and innovation.
In summary, the importance of the conclusion ties back to these dimensions. A comprehensive understanding of the current landscape and its likely future directions arms professionals and businesses with the foresight needed to succeed. The road ahead is indeed exciting, filled with opportunities for those prepared to adapt and innovate.