Unveiling the Potency of HDInsight Spark in Data Processing and Analytics
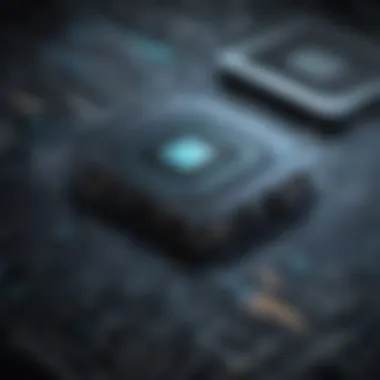
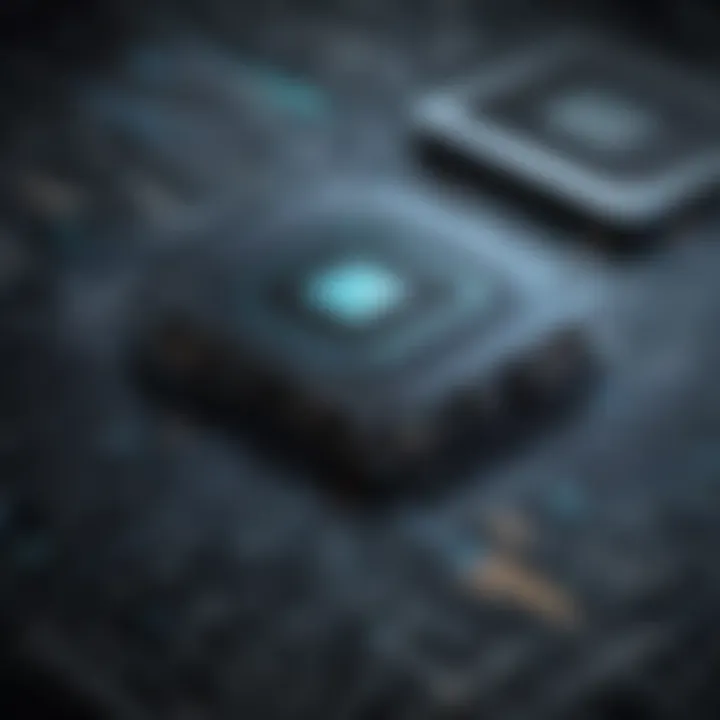
Software Overview
In this section, we will delve into an in-depth exploration of HDInsight Spark, a powerful tool reshaping big data processing and analytics capabilities. HDInsight Spark offers a wide array of features and functionalities that cater to the complex requirements of businesses and developers. Its pricing and licensing options vary depending on the specific needs and scale of operations. Supported across various platforms, HDInsight Spark ensures compatibility with different systems, enhancing its versatility and usability.
User Experience
Transitioning to the user experience aspect, HDInsight Spark excels in providing a seamless and user-friendly interface design. The platform emphasizes ease of use, allowing users to navigate effortlessly through its functionalities. Customizability is a key highlight, enabling users to tailor settings to suit their preferences and requirements. Additionally, HDInsight Spark boasts excellent performance and speed, optimizing data processing efficiency and delivering rapid results.
Pros and Cons
When evaluating the pros and cons, it becomes evident that HDInsight Spark offers various strengths and advantages. Its scalability, robust data processing capabilities, and integration with other tools set it apart in the market. However, some limitations exist, such as the learning curve associated with mastering its full potential and potential compatibility issues. A comparison with similar products showcases HDInsight Spark's unique features and competitive edge.
Real-world Applications
Exploring real-world applications, HDInsight Spark finds extensive use across different industries for diverse purposes. Case studies and success stories highlight the software's ability to solve specific problems effectively. Whether in finance, healthcare, or retail, HDInsight Spark plays a pivotal role in data analytics, decision-making, and enhancing operational efficiency.
Updates and Support
Keeping abreast of updates and support, HDInsight Spark ensures regular software updates to enhance functionality and address any glitches promptly. Customers benefit from a range of support options, including dedicated customer service channels and community forums for troubleshooting and knowledge sharing. This robust support system empowers users to maximize their utilization of HDInsight Spark and stay informed about the latest developments in big data analytics.
Introduction to HDInsight Spark
In the realm of big data processing and analytics, understanding HDInsight Spark is paramount. This section forms the bedrock of our exploration, laying the foundation for a deep dive into its intricacies. HDInsight Spark stands as a powerful tool, revolutionizing how businesses handle data, make strategic decisions, and unlock insights. By comprehending the core principles and functionalities of HDInsight Spark, enterprises can harness its full potential to drive innovation and competitive advantage.
Understanding the Basics
The Evolution of HDInsight Spark
Delving into the history of HDInsight Spark unveils a trajectory marked by continual advancements in data processing technologies. The Evolution of HDInsight Spark has been characterized by a relentless pursuit of efficiency, scalability, and real-time capabilities. Its evolution stems from the necessity of businesses to handle vast amounts of data with speed and precision, positioning it as a preferred choice for modern data analytics. The unique feature of the Evolution of HDInsight Spark lies in its adaptability to changing data landscapes, ensuring that businesses stay ahead in the data-driven age.
Key Features and Functionality
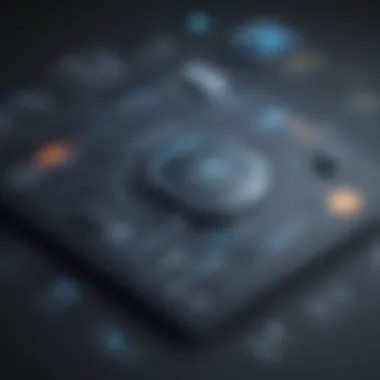
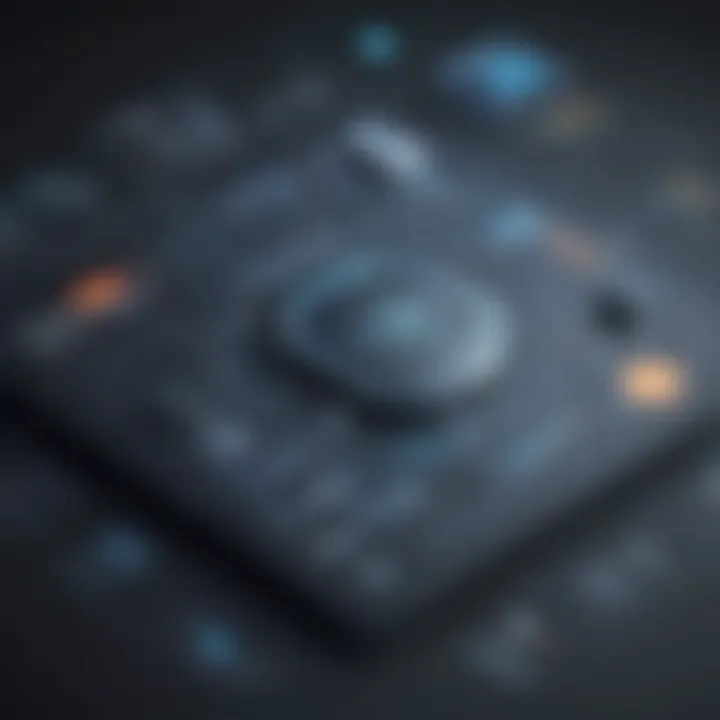
Exploring the Key Features and Functionality of HDInsight Spark reveals a comprehensive suite of tools designed to streamline data processing and analytics tasks. The crux of its appeal lies in its robust set of features that offer unmatched performance, scalability, and versatility. The Key Features and Functionality of HDInsight Spark empower organizations to tackle complex data challenges with ease, facilitating the extraction of valuable insights from structured and unstructured data sources. Despite its advantages, considerations around resource optimization and data governance must be meticulously managed to maximize the benefits of this cutting-edge technology.
Advantages of HDInsight Spark
Scalability and Performance
The scalability and performance prowess of HDInsight Spark underpin its position as a game-changer in the realm of big data analytics. Its ability to seamlessly scale resources based on workload demands ensures that organizations can efficiently process and analyze massive datasets without compromising speed or accuracy. The key characteristic of Scalability and Performance in HDInsight Spark is its elastic nature, allowing for dynamic resource allocation to handle fluctuating workloads effortlessly. However, achieving optimal scalability and performance requires careful planning and resource allocation to prevent bottlenecks and optimize system efficiency.
Real-time Processing Capabilities
Real-time Processing Capabilities signify the responsiveness and agility embedded within HDInsight Spark, enabling organizations to analyze data streams instantaneously. This critical feature empowers businesses to make informed decisions in real-time, harnessing the power of data as events unfold. The unique advantage of Real-time Processing Capabilities lies in its ability to support continuous data processing, ensuring that organizations can derive actionable insights with minimal latency. While real-time analytics offer a competitive edge, proper monitoring and fine-tuning are essential to maintain processing integrity and accuracy in high-velocity data environments.
Getting Started with HDInsight Spark
In the realm of big data processing and analytics, embarking on the journey with HDInsight Spark is not only crucial but also transformative. Setting the foundation right from the start can determine the success of the entire data operation. Getting Started with HDInsight Spark entails establishing a robust infrastructure that can handle vast amounts of data efficiently and effectively. It involves configuring and deploying a Spark cluster optimized for performance and scalability, thus laying the groundwork for seamless data processing and analytics. Initiating one's exploration of HDInsight Spark with a solid setup ensures that subsequent tasks and analyses can be conducted with precision and speed, maximizing the potential insights to be derived from the data.
Setting Up a HDInsight Spark Cluster
Configuration and Deployment
Configuring and deploying a HDInsight Spark cluster is a pivotal aspect of integrating Spark into the data ecosystem. The configuration process involves fine-tuning various parameters such as cluster size, instance types, and storage options to meet the specific requirements of the data workload. Deploying the cluster ensures that the chosen configurations are implemented and operational, ready to handle data processing tasks. The key characteristic of this configuration is its flexibility, allowing organizations to customize the cluster according to their data processing needs. By tailoring the cluster setup, users can optimize resource utilization and maximize the performance of Spark applications. While the configuration and deployment process may require careful consideration and expertise, the benefits of a well-optimized cluster are manifold, resulting in faster processing times, efficient resource allocation, and overall cost-effectiveness.
Optimizing Cluster Performance
Optimizing cluster performance is essential to leverage the full potential of HDInsight Spark for big data processing. By fine-tuning various parameters such as executor memory, core allocation, and inputoutput configurations, organizations can enhance the efficiency and speed of data processing tasks. The key characteristic of performance optimization lies in achieving the best balance between resource utilization and task execution speed. By optimizing the cluster performance, organizations can ensure that Spark jobs are executed swiftly and accurately, leading to improved analytics outcomes. One unique feature of optimizing cluster performance is the ability to monitor and adjust performance parameters in real-time, allowing for dynamic optimization based on workload demands. While there may be some challenges in finding the optimal configurations, the advantages of improved cluster performance, such as reduced processing times and enhanced scalability, make the effort worthwhile.
Exploring Data Processing with Spark
Data Ingestion and ETL Processes
Data ingestion and ETL (extract, transform, load) processes are foundational steps in data processing with Spark. Ingesting data involves importing raw data from various sources into the Spark environment for analysis. ETL processes encompass transforming the raw data into a structured format that is optimized for analytics. The key characteristic of data ingestion and ETL processes is their role in preparing the data for downstream analysis, ensuring that the data is clean, consistent, and ready for processing. One unique feature of these processes is their ability to handle large volumes of data efficiently, enabling organizations to analyze massive datasets with ease. While there may be challenges in managing complex data transformations and ensuring data quality, the advantages of streamlined data processing and analysis outweigh the difficulties, leading to more accurate insights and informed decision-making.
Running Spark Jobs
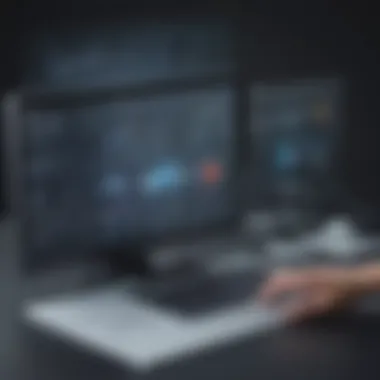
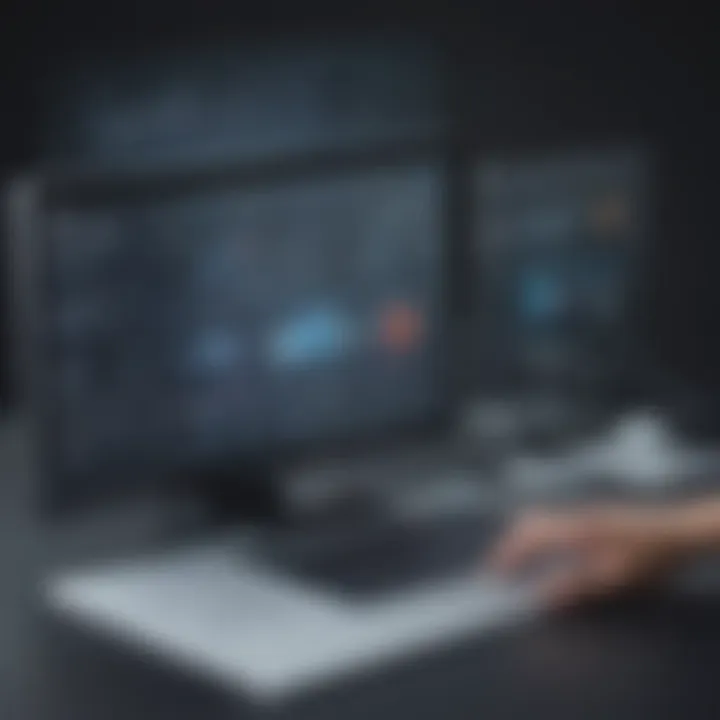
Running Spark jobs is where the magic of data analytics truly comes to life. By executing Spark jobs, organizations can perform various data processing tasks, from simple data transformations to complex machine learning algorithms. The key characteristic of running Spark jobs is their versatility, allowing users to run different types of analytics and computations on diverse datasets. One unique feature of running Spark jobs is the ability to achieve high performance and scalability, thanks to Spark's in-memory processing capabilities. While there may be considerations around job scheduling, fault tolerance, and job monitoring, the advantages of running Spark jobs include faster data processing, real-time insights, and the ability to handle large-scale analytics tasks effectively.
Utilizing HDInsight Spark for Big Data Analytics
In the realm of big data analytics, the utilization of HDInsight Spark plays a pivotal role in driving insights and deriving valuable information from massive datasets. HDInsight Spark offers a sophisticated framework that empowers businesses to perform complex data analytics tasks efficiently and effectively. By harnessing the power of HDInsight Spark for big data analytics, organizations can uncover patterns, trends, and correlations within their data, enabling data-driven decision-making processes.
Machine Learning Applications
Implementing MLlib for Predictive Analytics
Implementing MLlib for Predictive Analytics within the HDInsight Spark environment is a game-changer for organizations looking to leverage machine learning capabilities. MLlib, a scalable machine learning library, provides a wide range of algorithms and tools for building predictive models and performing advanced analytics. The key characteristic of Implementing MLlib for Predictive Analytics lies in its ability to handle large-scale datasets efficiently, making it a preferred choice for predictive analytics projects. The unique feature of MLlib is its seamless integration with Spark, allowing for distributed processing of machine learning tasks across multiple nodes. This integration enhances performance and scalability, making MLlib a valuable asset for predictive analytics initiatives.
Feature Engineering with Spark
Feature Engineering with Spark is an essential component of enhancing the quality and efficacy of machine learning models. By extracting relevant features and transforming data for better model performance, Feature Engineering with Spark optimizes the predictive capabilities of machine learning algorithms. The key characteristic of Feature Engineering with Spark is its ability to preprocess data, handle missing values, and engineer new features that improve model accuracy and efficiency. This process is crucial for developing robust machine learning models that can generalize well to unseen data. The unique feature of Feature Engineering with Spark is its flexibility in feature selection and transformation, enabling data scientists to experiment with different feature sets to enhance model performance.
Real-time Data Analytics
Streaming Analytics with Spark Streaming
Streaming Analytics with Spark Streaming revolutionizes real-time data processing by enabling real-time insights and decision-making capabilities. The key characteristic of Spark Streaming lies in its micro-batch processing approach, which allows for the seamless integration of streaming data with batch data processing. This hybrid approach ensures low latency and high throughput, making it a beneficial choice for real-time data analytics in diverse applications. The unique feature of Spark Streaming is its fault tolerance and exactly-once processing semantics, ensuring data reliability and consistency in real-time analytics scenarios.
Interactive Data Exploration
Interactive Data Exploration with HDInsight Spark provides a dynamic and intuitive way to analyze and visualize data in real time. By offering interactive query capabilities and visualizations, Interactive Data Exploration empowers users to explore data insights rapidly and iteratively. The key characteristic of Interactive Data Exploration is its interactive query performance and scalability, allowing users to seamlessly navigate vast datasets and conduct ad-hoc analyses with ease. This feature makes it a popular choice for data analysts and business users seeking to gain actionable insights quickly and efficiently.
Optimizing Performance and Efficiency
In our comprehensive exploration of HDInsight Spark, the section on Optimizing Performance and Efficiency takes center stage to elucidate crucial strategies that ensure seamless operations and enhanced productivity. This pivotal topic delves into the intricacies of fine-tuning the Spark applications to maximize efficiency and performance. Understanding the nuances of optimization techniques and resource management strategies is paramount in harnessing the full potential of HDInsight Spark for big data processing and analytics.
Fine-tuning Spark Applications
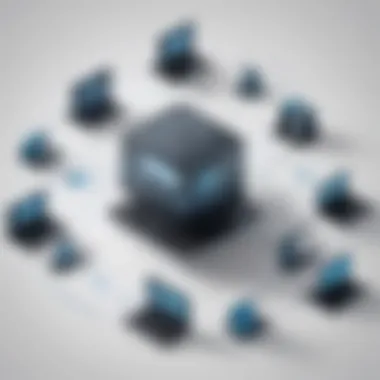
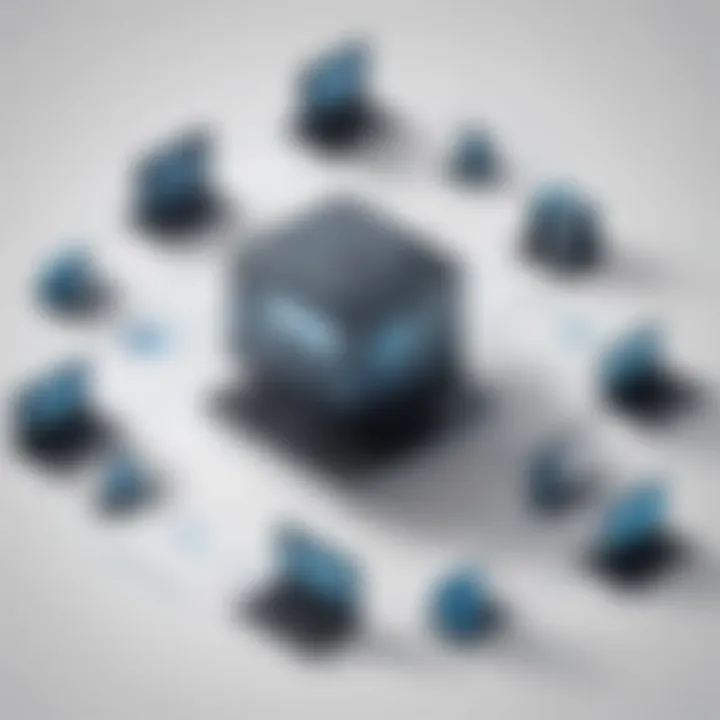
Optimization Techniques
When delving into optimization techniques within the realm of HDInsight Spark, it is imperative to grasp the essence of fine-tuning algorithms and processes to achieve peak performance. These techniques play a critical role in streamlining data processing, improving response times, and overall system efficiency. The key characteristic of optimization techniques lies in their ability to decipher complex data sets swiftly and accurately, significantly boosting the analytical capabilities of Spark applications. Their unique feature lies in their adaptability to diverse data structures and volumes, rendering them as a popular choice for enhancing performance in varied scenarios. While optimization techniques offer undeniable advantages in accelerating data processing and enhancing analytical precision, their meticulous application is essential to avoid potential bottlenecks or performance inconsistencies.
Resource Management Strategies
Resource management strategies form the backbone of efficient Spark applications, dictating how computing resources are allocated and utilized within the cluster environment. These strategies optimize resource allocation, ensuring that tasks are executed with minimal latency and maximal throughput. The key characteristic of resource management strategies is their ability to dynamically adjust resources based on workload demands, promoting optimal performance under varying conditions. Their unique feature lies in the capacity to prioritize critical tasks, allocate resources judiciously, and prevent resource contention, thereby elevating the stability and reliability of Spark clusters. While resource management strategies offer unparalleled benefits in enhancing scalability and performance, careful implementation and monitoring are vital to prevent resource wastage or underutilization.
Monitoring and Troubleshooting
Under the overarching theme of optimizing performance and efficiency, Monitoring and Troubleshooting emerge as indispensable facets in maintaining the robustness and reliability of HDInsight Spark environments. These aspects focus on constant surveillance, identification of potential issues, and prompt resolution to uphold seamless operations and mitigate downtime effectively.
Logging and Alerting
Logging and Alerting
Logging and alerting mechanisms within HDInsight Spark serve as the eyes and ears of the system, capturing critical information about cluster activities, alerting administrators to anomalies, and facilitating proactive response to emerging issues. The key characteristic of logging and alerting lies in their role as real-time diagnostic tools, enabling swift detection of errors, performance degradation, or security breaches. Their unique feature encompasses customizable alert thresholds, automated notification systems, and comprehensive log storage functionalities, making them indispensable for ensuring operational transparency and timely intervention. While logging and alerting mechanisms offer intrinsic advantages in maintaining system health and performance integrity, effective configuration and periodic review are essential to optimize their efficacy and minimize false positives.
Identifying Performance Bottlenecks
Identifying performance bottlenecks is a fundamental aspect of troubleshooting in HDInsight Spark, aimed at pinpointing inefficiencies or constraints that impede optimal performance. This process involves analyzing various system components, data flow paths, and resource utilization patterns to isolate bottlenecks and address them proactively. The key characteristic of identifying performance bottlenecks is its diagnostic precision, providing insights into areas of contention, latency, or inefficiency within the Spark environment. Their unique feature lies in the ability to correlate performance metrics across different layers of the ecosystem, facilitating root cause analysis and targeted optimizations. While identifying performance bottlenecks offers invaluable advantages in streamlining operations and enhancing system resilience, continuous monitoring and refinement are crucial to address evolving performance challenges.
Enhancing Data Security with HDInsight Spark
In the realm of big data processing and analytics, enhancing data security with HDInsight Spark emerges as a critical facet to safeguard sensitive information and uphold data integrity. Security measures play a pivotal role in ensuring that data remains protected from unauthorized access or breaches, instilling trust in the system and compliance with industry regulations and standards. By focusing on the security aspect of HDInsight Spark, businesses and developers can fortify their data infrastructure against potential threats and vulnerabilities, thereby enhancing overall data security posture.
Security Best Practices
Data Encryption and Access Control
Data encryption and access control stand out as fundamental components of data security within the HDInsight Spark framework. Encryption involves encoding data in such a way that only authorized parties with the decryption key can access and decipher it, thereby mitigating the risks associated with data interception or theft. Access control mechanisms dictate who can view, modify, or delete specific data within the system, preventing unauthorized users from tampering with sensitive information. The use of data encryption and access control in HDInsight Spark ensures confidentiality, integrity, and availability of data, contributing to a robust security architecture that aligns with best practices in data protection.
Securing Data in Transit and at Rest
Securing data in transit and at rest is paramount in safeguarding data as it moves between various components of the HDInsight Spark ecosystem and while it resides in storage. Data in transit refers to information being transmitted between nodes or systems, necessitating encryption and secure communication protocols to prevent unauthorized interception or tampering during transmission. On the other hand, data at rest pertains to stored data that is not actively moving across the network, requiring encryption mechanisms to protect it from unauthorized access or retrieval. By implementing data security protocols for both data in transit and at rest, organizations can uphold confidentiality, data integrity, and regulatory compliance standards within their HDInsight Spark environment.
Compliance and Governance
In the landscape of data security and privacy, compliance and governance mechanisms serve as key pillars in ensuring that organizations adhere to legal regulations, industry standards, and internal policies governing data handling and protection. Regulatory compliance considerations entail aligning data practices with relevant laws and regulations specific to the industry or region in which the organization operates. By adhering to regulatory mandates, businesses can avoid potential penalties, sanctions, or reputational damage resulting from non-compliance. On the other hand, data privacy frameworks offer a structured approach to managing and safeguarding sensitive data, encompassing procedures, policies, and mechanisms to ensure data privacy and confidentiality are preserved throughout the data lifecycle. By integrating compliance and governance practices into the HDInsight Spark environment, organizations can establish a culture of data accountability, transparency, and ethical data management that builds trust among stakeholders and instills confidence in data security practices.
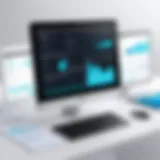
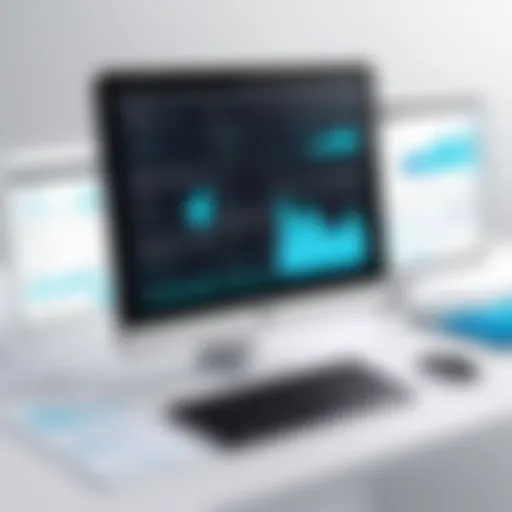