Unlocking the Power of Big Data: A Comprehensive Guide to Strategic Decision-Making
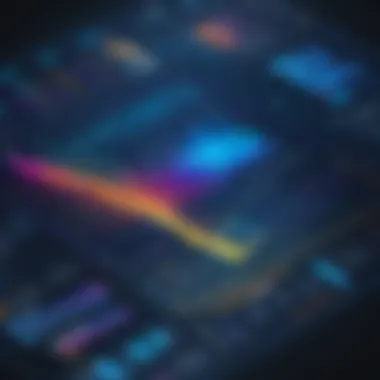
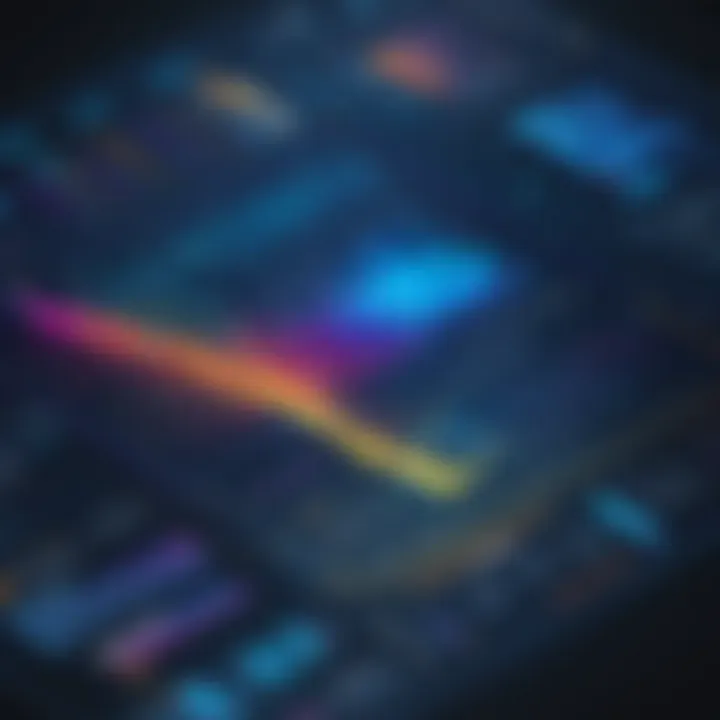
Software Overview
Big data is a revolutionary field that holds immense power for businesses and organizations keen on leveraging data for informed decision-making and innovation. In this comprehensive guide, we delve into the intricate details of big data with a focus on its significance, applications across industries, and the transformative impact it brings. Explore the core features and functionalities of big data software, examine pricing structures and licensing options to align with your business needs effectively. Gain insights into the supported platforms and compatibility requirements to ensure seamless integration into your existing systems.
User Experience
When it comes to user experience, the ease of use and intuitive interface design can significantly impact the efficiency and adoption of big data software. Understanding the customizability and range of user settings empowers businesses to tailor the software to their specific requirements seamlessly. Evaluate the performance and speed metrics to ascertain optimal functionality in handling large datasets and complex analytics tasks efficiently.
Pros and Cons
Unveiling the strengths and advantages of big data software showcases the capabilities that drive strategic decision-making and competitive edges for businesses. Simultaneously, addressing the drawbacks and limitations offers a nuanced perspective on areas that may require enhancements or alternative approaches. Comparing big data software with similar products elucidates key differentiators, aiding in informed decision-making.
Real-world Applications
Exploring industry-specific uses of big data software elucidates the breadth of applications across sectors like healthcare, finance, retail, and more. Engage with compelling case studies and success stories that highlight the practical impact of utilizing big data for optimizing operations and driving business growth. Dive into how the software addresses specific challenges and solves complex problems faced by diverse organizations.
Updates and Support
Regular software updates are crucial for maintaining peak performance and staying ahead of evolving data trends. Assess the frequency of updates to ensure continued relevance and functionality. Explore customer support options to access timely assistance and troubleshoot any issues effectively. Engage with community forums and user resources to enhance expertise and leverage collective knowledge in optimizing big data operations.
Introduction to Big Data
In this section of our comprehensive guide, we explore the fundamental aspects of big data. Big data, a term used to describe data sets that are too large and complex for traditional data processing applications, holds immense value in today's data-driven world. Understanding big data is crucial for organizations looking to extract valuable insights from vast amounts of information. In this article, we delve into the nuances of big data, highlighting its importance in decision-making and innovation across various industries.
Defining Big Data
Volume:
Volume is a critical element of big data, referring to the sheer amount of data generated and collected. The sheer volume of data contributes significantly to the challenges and opportunities presented by big data. Large quantities of data facilitate more in-depth analysis and pattern identification, making volume a key consideration in leveraging big data effectively. While handling large volumes of data can be resource-intensive, the insights gained can be immensely valuable, driving strategic decision-making and innovation.
Velocity:
Velocity pertains to the speed at which data is generated and processed. In the realm of big data, real-time or near-real-time data processing is essential for organizations to extract actionable insights promptly. The rapid influx of data from various sources necessitates efficient processing to derive meaningful conclusions. Velocity plays a crucial role in enabling timely decision-making and facilitating agile responses to changing dynamics.
Variety:
Variety represents the diverse types and sources of data that organizations encounter. Big data encompasses structured and unstructured data from multiple channels, including text, audio, video, and social media. Managing the variety of data types poses a significant challenge but also opens up opportunities for comprehensive analysis and holistic decision-making. Embracing data variety allows organizations to gain a more comprehensive understanding of their operations and customers.
Importance of Big Data
Data-Driven Decision Making:
Data-driven decision making involves basing strategic choices on factual analysis and interpretation of data rather than intuition alone. This approach enables organizations to make informed decisions based on quantitative evidence, leading to more precise outcomes and reduced uncertainty. By leveraging data analytics and visualization tools, businesses can enhance their decision-making processes and drive efficiencies across all functions.
Enhanced Customer Insights:
Enhanced customer insights leverage data to understand customer behaviors, preferences, and trends more deeply. By analyzing customer interactions and feedback, businesses can personalize their offerings, improve customer satisfaction, and foster long-lasting relationships. The ability to obtain comprehensive customer insights through data analytics is a game-changer in today's competitive landscape.
Predictive Analytics:
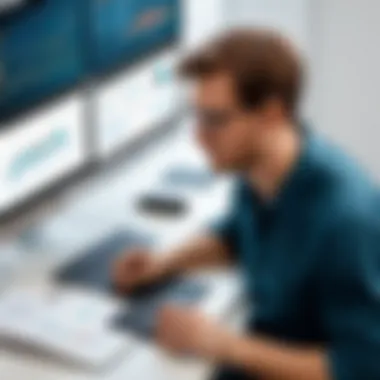
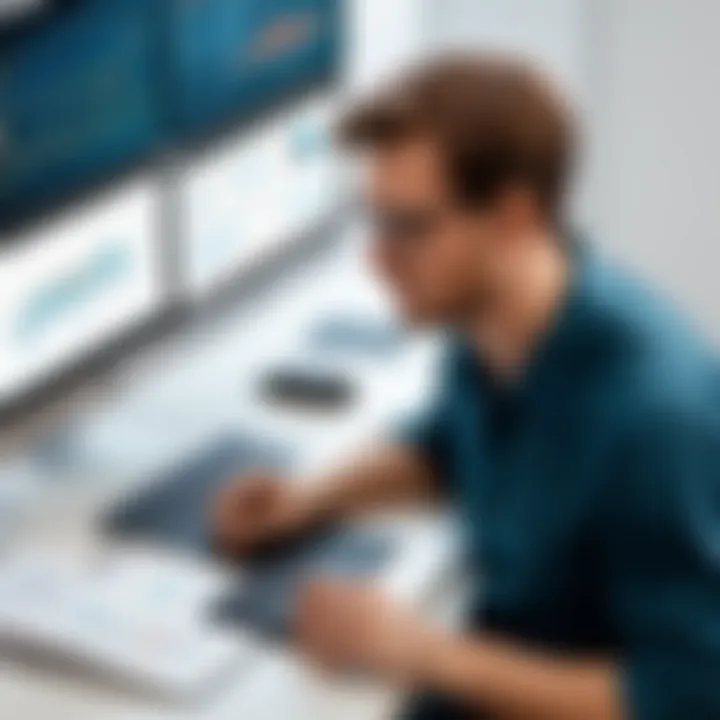
Predictive analytics involves utilizing historical data and statistical algorithms to forecast future outcomes accurately. By identifying patterns and trends within vast datasets, organizations can anticipate market trends, customer behavior, and potential risks. Predictive analytics empowers businesses to proactively address challenges and capitalize on opportunities, driving sustainable growth and innovation.
Evolution of Big Data Technologies
Hadoop:
Hadoop, an open-source framework, revolutionized big data processing by enabling distributed storage and processing of large datasets across clusters of computers. Its scalability, fault tolerance, and cost-effectiveness make it a preferred choice for organizations dealing with massive volumes of data. Hadoop's architecture allows for parallel processing, enhancing performance and optimizing resource utilization for data-intensive tasks.
Apache Spark:
Apache Spark is a powerful data processing engine known for its speed and versatility in handling complex data processing tasks. By leveraging in-memory computation and advanced analytics capabilities, Spark facilitates rapid data processing and real-time analytics. Its compatibility with diverse data sources and streamlined data pipeline management make it a top choice for organizations seeking efficient data processing solutions.
NoSQL Databases:
No SQL databases offer flexible, schema-less data storage solutions that can handle large volumes of unstructured data. Unlike traditional relational databases, NoSQL databases provide scalability and agility, enabling organizations to store and retrieve data efficiently. With features like high availability and horizontal scaling, NoSQL databases have become integral to big data applications that require dynamic and diverse data storage requirements.
Applications of Big Data
Big Data application is a vital aspect in the realm of data analytics. Understanding the significance of leveraging vast amounts of data for strategic decision-making and business innovation is crucial. In this article, we will delve into the various elements, benefits, and considerations of applying Big Data in real-world scenarios across different industries.
Financial Services
Risk Management
Risk management plays a fundamental role in the financial services sector by assisting organizations in identifying and mitigating potential risks. The key characteristic of risk management lies in its ability to proactively address uncertainties that could impact business operations. In this article, we will explore why risk management is a popular choice for mitigating financial risks and delve into its unique features, outlining the advantages and disadvantages of incorporating risk management strategies.
Fraud Detection
Fraud detection is a critical component of financial services aimed at identifying and preventing fraudulent activities. The primary focus of fraud detection is on analyzing patterns and anomalies within financial transactions to pinpoint potential instances of fraud. This article will highlight the significance of fraud detection in enhancing financial security, delving into its key characteristics, and discussing why it is a favored approach in combating fraudulent activities.
Customer Segmentation
Customer segmentation is an essential practice in financial services for targeted marketing and personalized customer experiences. By categorizing customers based on specific characteristics, financial institutions can tailor their services to meet individual needs effectively. In this article, we will shed light on the benefits of customer segmentation, explore its unique features, and evaluate the advantages and disadvantages of implementing customer segmentation strategies.
Healthcare
Personalized Medicine
Personalized medicine revolutionizes healthcare by providing tailored treatment plans based on an individual's genetic makeup and medical history. It offers precise healthcare solutions that cater to the unique needs of patients. This article will delve into the advantages and disadvantages of personalized medicine, highlighting its key characteristics and explaining why it is a valuable approach in healthcare innovation.
Disease Outbreak Prediction
Disease outbreak prediction utilizes data analytics to forecast and monitor the spread of diseases, enabling proactive measures to contain potential epidemics. By leveraging advanced algorithms and real-time data analysis, healthcare professionals can identify disease trends and take timely action. This section will explore the role of disease outbreak prediction in public health, emphasizing its key characteristics and discussing the benefits and drawbacks of predictive analytics in disease surveillance.
Healthcare Management
Efficient healthcare management is paramount for ensuring seamless operations within healthcare facilities. By optimizing resource allocation, scheduling, and patient care processes, healthcare management enhances overall service delivery. This article will elucidate the benefits and challenges of healthcare management, highlighting its key features and exploring how it contributes to improved healthcare outcomes.
E-Commerce
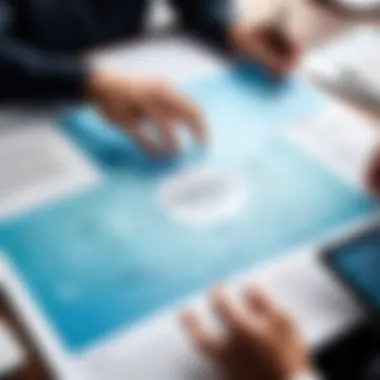
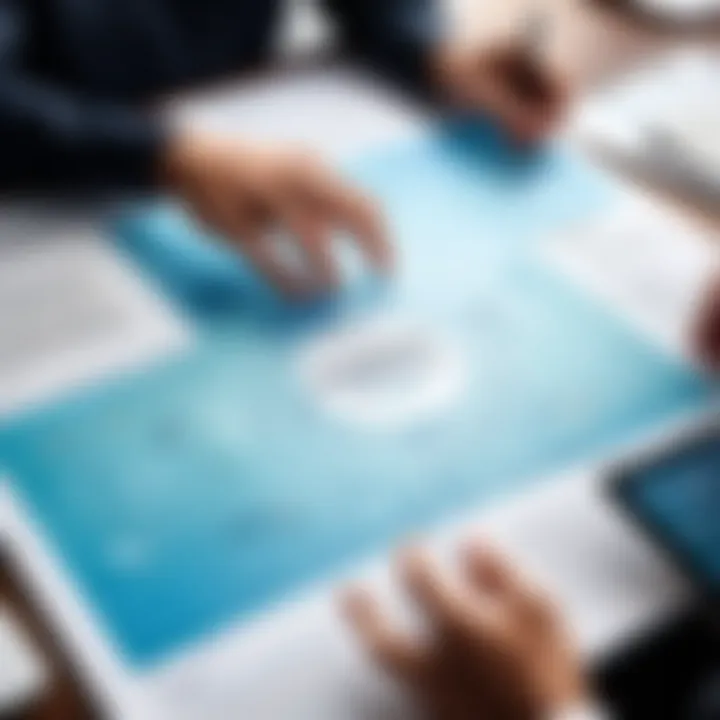
Recommendation Systems
Recommendation systems are integral to e-commerce platforms, providing personalized product suggestions to enhance the shopping experience for customers. By analyzing user behavior and preferences, these systems offer tailored recommendations that drive sales and customer satisfaction. In this section, we will examine the significance of recommendation systems in e-commerce, discuss their key features, and assess the pros and cons of incorporating recommendation systems in online retail strategies.
Market Basket Analysis
Market basket analysis uncovers patterns in consumer purchasing behaviors to optimize product placement and promotions. By understanding product associations, e-commerce businesses can devise targeted marketing strategies to increase sales and customer engagement. This article will delve into the unique features of market basket analysis, explain why it is a valuable tool for e-commerce optimization, and outline the advantages and limitations of employing market basket analysis techniques.
Dynamic Pricing
Dynamic pricing mechanisms adjust product prices in real-time based on market demand, competitor pricing, and other variables. This pricing strategy allows e-commerce businesses to optimize profit margins and remain competitive in the digital marketplace. We will explore the role of dynamic pricing in e-commerce, analyze its key characteristics, and discuss the benefits and challenges associated with implementing dynamic pricing models.
Challenges and Opportunities
When delving into the complexity of big data, it is crucial to address the multifaceted nature of challenges and opportunities that come with it. Understanding the nuances of data security, skills gap, and ethical considerations is paramount for organizations aiming to leverage big data effectively. By focusing on these specific elements within the realm of challenges and opportunities, businesses can enhance their decision-making processes and drive innovation.
Data Security
Privacy Concerns
Privacy concerns play a pivotal role in the realm of big data, emphasizing the significance of protecting sensitive information amidst massive data streams. The key characteristic of privacy concerns lies in safeguarding personal and confidential data from unauthorized access or misuse. This aspect is a critical choice for this article as it sheds light on the essential need for secure data handling, especially in an era where privacy breaches are a growing concern. The unique feature of privacy concerns is their ability to uphold individual rights while promoting data integrity and trust within organizations.
Cybersecurity Threats
Within the landscape of big data, cybersecurity threats pose a significant challenge to data integrity and system reliability. The key characteristic of cybersecurity threats rests in identifying and mitigating potential risks associated with data breaches and malicious activities. This aspect is crucial for this article as it highlights the importance of maintaining robust cybersecurity measures in an increasingly digital environment. The unique feature of cybersecurity threats lies in their proactive approach towards preempting cyber attacks and ensuring data security compliance.
Regulatory Compliance
The adherence to regulatory compliance standards is essential in navigating the intricate web of big data governance and legal frameworks. The key characteristic of regulatory compliance centers around aligning data practices with established guidelines and laws to ensure transparency and accountability. This aspect is a valuable choice for this article as it emphasizes the significance of regulatory frameworks in mitigating risks and promoting responsible data management. The unique feature of regulatory compliance lies in its ability to establish a structured framework for data handling that balances innovation with legal requirements.
Skills Gap
Need for Data Scientists
The evolving landscape of big data underscores the critical need for skilled data scientists who can interpret and derive valuable insights from complex data sets. The key characteristic of the need for data scientists lies in their expertise in data analysis, algorithm development, and statistical modeling. This aspect is instrumental for this article as it highlights the pivotal role of data scientists in driving data-driven decision-making processes. The unique feature of the need for data scientists is their capacity to bridge the gap between raw data and actionable business intelligence, providing a competitive edge to organizations.
Training Programs
Effective training programs play a pivotal role in equipping professionals with the necessary skills and knowledge to harness the power of big data effectively. The key characteristic of training programs lies in their ability to impart specialized training in data analytics, machine learning, and data visualization techniques. This aspect is a beneficial choice for this article as it emphasizes the importance of continuous learning and skill development in the realm of big data. The unique feature of training programs is their structured approach towards cultivating expertise in data-related technologies, enabling professionals to stay abreast of industry trends and best practices.
Upskilling Workforce
The process of upskilling the workforce involves enhancing their existing skill sets to meet the evolving demands of the digital era driven by big data. The key characteristic of upskilling the workforce lies in promoting a culture of continuous learning and professional growth within organizations. This aspect is a popular choice for this article as it underlines the imperative of investing in human capital to maximize the potential of big data initiatives. The unique feature of upskilling the workforce is its transformative impact on employee productivity, organizational agility, and competitive advantage in the data-driven landscape.
Ethical Considerations
Data Bias
Addressing data bias is crucial in ensuring the fairness and accuracy of insights derived from big data analytics. The key characteristic of data bias lies in identifying and mitigating bias that may skew analytical outcomes or perpetuate existing disparities. This aspect is a beneficial choice for this article as it emphasizes the ethical responsibility of organizations to uphold impartiality and equality in data-driven decision-making processes. The unique feature of data bias awareness is its capacity to foster a culture of inclusivity and integrity within data-centric operations.
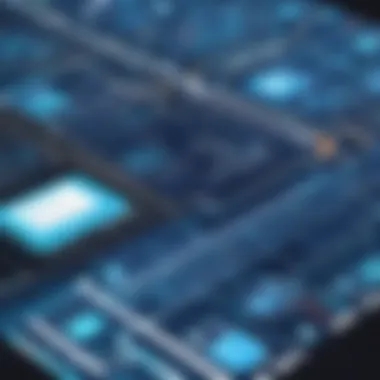
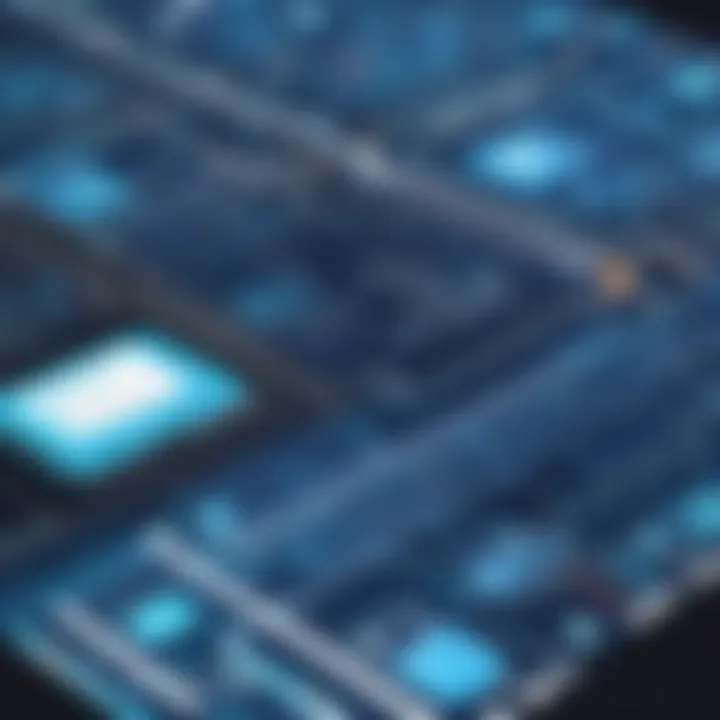
Algorithmic Fairness
Algorithmic fairness emphasizes the importance of developing algorithms that mitigate bias and promote equitable outcomes for all user groups. The key characteristic of algorithmic fairness lies in designing algorithms that prioritize fairness, accountability, and transparency in data processing and decision-making. This aspect is a popular choice for this article as it highlights the ethical dimensions of algorithm development and deployment in the context of big data analytics. The unique feature of algorithmic fairness is its potential to enhance the credibility and trustworthiness of automated decision systems, fostering public confidence and stakeholder engagement.
Transparency
Transparency in data processes and decision-making ensures accountability and trustworthiness in the utilization of big data technologies. The key characteristic of transparency lies in promoting openness, clarity, and disclosure in data practices to enhance stakeholder understanding and confidence. This aspect is a valuable choice for this article as it underscores the importance of fostering a culture of transparency and ethical conduct in the realms of data collection, analysis, and utilization. The unique feature of transparency is its capacity to enhance data governance practices, uphold regulatory compliance, and safeguard organizational reputation in an increasingly data-centric environment.
Future Trends in Big Data
Big Data analytics have seen a significant shift towards the integration of Machine Learning, paving the way for substantial advancements in data analysis techniques and predictive capabilities. This evolution stands as a testament to the transformative power of harnessing data-driven insights. Machine Learning integration within Big Data not only allows for automated insights but also emphasizes deep learning applications and predictive modeling, revolutionizing decision-making and strategic planning processes.
Machine Learning Integration:
Automated Insights:
Automated Insights, a crucial component of Machine Learning Integration, plays a pivotal role in extracting actionable intelligence from vast datasets efficiently. By automating the data analysis process, organizations can uncover trends, patterns, and correlations that facilitate informed decision-making. The key characteristic of Automated Insights lies in its ability to identify valuable information swiftly, enabling businesses to stay agile and responsive in today's dynamic market landscape. The unique feature of Automated Insights is its capacity to process immense data volumes at remarkable speeds, propelling organizations towards optimized operational efficiency.
Deep Learning Applications:
Deep Learning Applications within Machine Learning Integration signify a sophisticated approach to data processing, enabling the extraction of intricate insights from complex datasets. The key characteristic of Deep Learning lies in its neural network-based algorithms, which mimic human brain functionality to analyze data layers comprehensively. This approach is favored in the article for its adeptness in handling unstructured data and recognizing intricate patterns, fostering enhanced decision-making based on nuanced insights. The advantage of Deep Learning lies in its ability to uncover hidden trends that traditional analytics might overlook, empowering businesses to gain a holistic perspective for strategic initiatives.
Predictive Modeling:
Predictive Modeling, a fundamental aspect of Machine Learning Integration, enriches data analysis by forecasting future trends and outcomes based on historical data patterns. The essence of Predictive Modeling lies in its ability to provide probabilistic projections that guide strategic planning and risk mitigation strategies. The advantage of Predictive Modeling is its capability to enhance decision-making by offering data-driven recommendations, giving organizations a competitive edge in dynamic market scenarios. However, a potential disadvantage of Predictive Modeling could be inaccuracies stemming from varied data sources or evolving market dynamics.
Edge Computing:
Edge Computing, a cutting-edge concept in Big Data architecture, focuses on processing data closer to its source to minimize latency and enhance real-time insights. By incorporating Io T devices, real-time data processing, and reduced latency mechanisms, Edge Computing streamlines data analysis and empowers organizations with instantaneous decision-making capabilities for improved operational efficiency.
IoT Devices:
The integration of Io T Devices in Edge Computing signifies a breakthrough in data transmission and analytics, leveraging interconnected sensors and devices to collect and disseminate real-time information. The key characteristic of IoT Devices lies in their ability to facilitate seamless data exchange and communication between interconnected devices, enabling comprehensive data analysis across diverse operational aspects. Organizations benefit from the unique feature of IoT Devices by gaining unparalleled visibility and control over disparate data sources, optimizing decision-making processes by leveraging real-time insights promptly.
Real-time Data Processing:
Real-time Data Processing within Edge Computing emphasizes the swift analysis of data streams to derive insights instantaneously and respond to emerging trends promptly. The key characteristic of Real-time Data Processing lies in its continuous monitoring and analysis capabilities, enabling organizations to detect anomalies and patterns in real-time. This approach is advantageous for the article due to its capacity to deliver actionable insights rapidly, enhancing decision-making accuracy and operational efficiency. However, a potential disadvantage of Real-time Data Processing could be the complexity of managing data streams effectively under high data volumes and processing requirements.
Reduced Latency:
Reduced Latency, a critical element of Edge Computing, minimizes the time delay in data transmission and processing, ensuring swift decision-making based on up-to-date information. The key characteristic of Reduced Latency lies in its ability to optimize data transfer speeds and processing times, enabling organizations to access real-time insights without delays. The advantage of Reduced Latency is its capacity to enhance operational responsiveness and customer service by enabling near-instantaneous data processing. Nonetheless, a potential disadvantage of Reduced Latency may be the resource-intensive nature of maintaining low latency levels across diverse data sources and applications.
Industry 4. Transformation:
Industry 4.0 Transformation redefines traditional manufacturing processes through the adoption of smart factories, digital twins, and supply chain optimization, ushering in a new era of streamlined production, enhanced efficiency, and predictive maintenance in industrial settings.
Smart Factories:
Smart Factories represent the pinnacle of Industry 4.0 Transformation, integrating cutting-edge technologies like Io T, AI, and robotics to automate and optimize manufacturing processes. The key characteristic of Smart Factories lies in their interconnected systems that enable seamless data exchange and operational coordination, resulting in efficient production cycles and minimized downtime. The unique feature of Smart Factories is their capacity to adapt to dynamic production demands through real-time data analysis, enhancing productivity and resource allocation for maximum efficiency.
Digital Twins:
Digital Twins embody a revolutionary approach within Industry 4.0 Transformation, creating virtual replicas of physical assets to monitor, analyze, and optimize operational performance. The key characteristic of Digital Twins lies in their ability to simulate real-world scenarios, enabling predictive maintenance and performance enhancements through data-driven insights. The advantage of Digital Twins is their potential to revolutionize fault detection and predictive analytics, mitigating risks and optimizing resource utilization. However, a potential disadvantage of Digital Twins could be the complexity of integrating virtual models with physical assets across diverse industrial domains.
Supply Chain Optimization:
Supply Chain Optimization signifies the strategic enhancement of logistical operations through predictive analytics, real-time tracking, and data-driven decision-making. The key characteristic of Supply Chain Optimization lies in its holistic approach to streamlining production, inventory management, and distribution processes, reducing costs and improving customer satisfaction. The advantage of Supply Chain Optimization is its potential to minimize inefficiencies and bottlenecks, fostering agility and competitiveness in the market. Nevertheless, a potential disadvantage of Supply Chain Optimization may be the substantial investment required for implementing advanced analytics tools and technologies across complex supply chain networks.