Unraveling the Enigma of Glue Data Lineage: Exploring Its Impact on Software Reviews
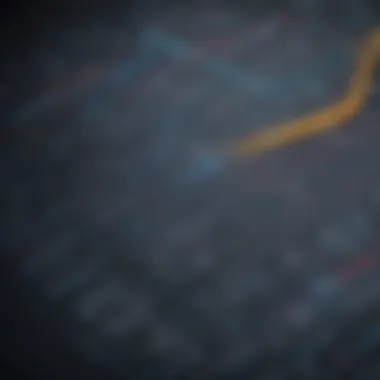
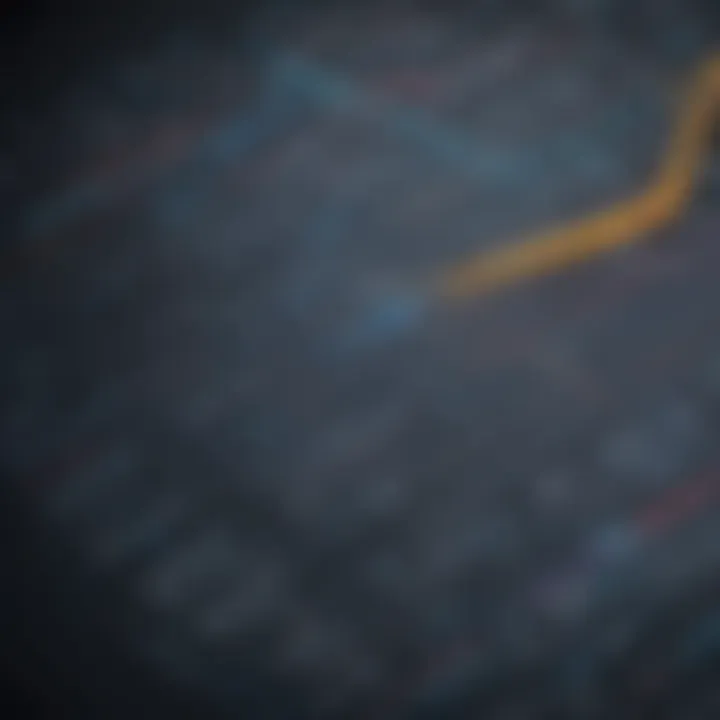
Software Overview
When delving into the intricate world of glue data lineage, understanding the software's fundamental features and functionalities becomes paramount. It is crucial to grasp how the software operates within the ecosystem of software reviews. Examining its pricing and licensing options provides insights into accessibility and affordability, further solidifying its relevance within the realm of data lineage analysis. Supported platforms and compatibility are essential aspects to consider, as they determine the software's versatility across different systems and interfaces.
User Experience
The user experience of software rooted in glue data lineage is a critical factor in analyzing its efficacy. Evaluating the software's ease of use and interface design unveils how seamlessly users can navigate through the complexities of data lineage. Customizability and user settings play a significant role in tailoring the software to meet specific requirements, enhancing user functionality and control. Performance and speed are key indicators of the software's operational efficiency, shedding light on its ability to handle vast amounts of data with precision and speed.
Pros and Cons
Unraveling the mysteries of glue data lineage reveals a myriad of strengths and advantages that the software brings to the table. From enhancing data tracking and analysis to facilitating information flow, the software's benefits are substantial. However, it is crucial to acknowledge the drawbacks and limitations as well. By comparing the software with similar products in the market, a comprehensive evaluation of its standing and unique selling points can be ascertained.
Real-world Applications
In exploring the implications of glue data lineage in real-world scenarios, delving into industry-specific uses becomes imperative. Case studies and success stories offer tangible examples of how the software has revolutionized data analysis and information dissemination. Understanding how the software solves specific problems aids in bridging the gap between theoretical concepts and practical applications, emphasizing its relevance in diverse professional settings.
Updates and Support
The frequency of software updates directly impacts its relevancy and competitiveness in the market. Customer support options and accessibility to community forums and user resources play a vital role in addressing user queries and resolving technical issues promptly. By providing regular updates and fostering a supportive user community, the software can continuously evolve and adapt to the dynamic landscape of data lineage analysis.
Introduction to Glue Data Lineage
Glue data lineage serves as a critical component within the realm of software reviews. Understanding the intricate web of data lineage is paramount for software professionals aiming to enhance data accuracy and facilitate data traceability. By delving into the complexities of glue data lineage, IT professionals can gain profound insights into how information flows and evolves across various software platforms. This section will unravel the core aspects and benefits of glue data lineage, shedding light on its pivotal role in the software ecosystem.
Defining Glue Data Lineage
Understanding the concept
Delving into 'Understanding the concept' of glue data lineage reveals a multifaceted approach to tracing data origins and transformations. This specific aspect explores how data flows within software systems, emphasizing the interconnected nature of data sources. The key characteristic of 'Understanding the concept' lies in its ability to provide a comprehensive map of data evolution, enabling software professionals to track and analyze data changes effectively. The unique feature of 'Understanding the concept' is its capacity to enhance data accuracy by offering a clear depiction of data lineage paths, although it may present challenges in interpreting complex data relationships.
Key components
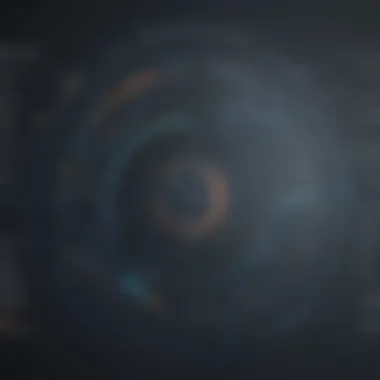
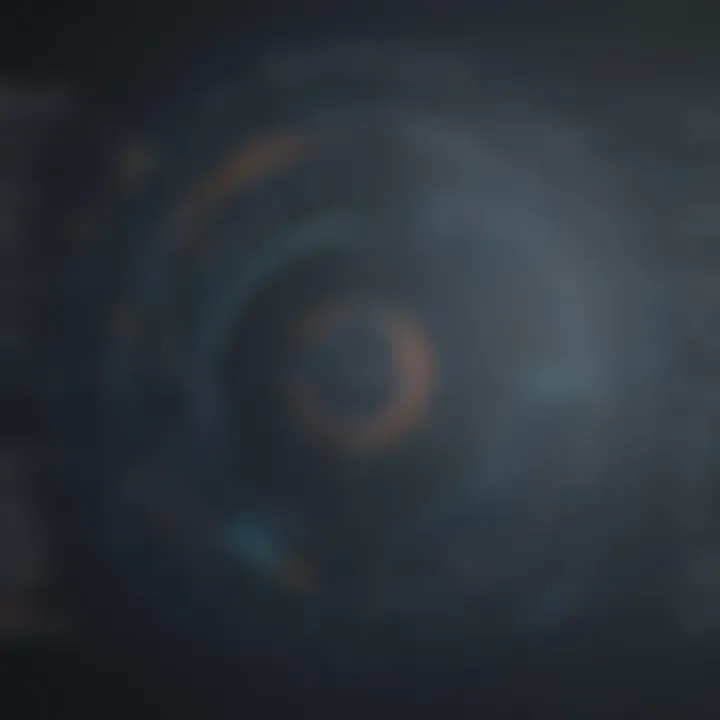
The 'Key components' of glue data lineage focus on the foundational elements that constitute a robust data lineage framework. These components play a crucial role in identifying data sources, transformations, and destinations within a software environment. Highlighting the key characteristic of 'Key components' underscores their significance in establishing a coherent data lineage structure that promotes data accuracy and traceability. Emphasizing the unique features of 'Key components' showcases their advantages in ensuring data integrity and consistency. However, complexities in managing diverse data sources may pose challenges in maintaining an accurate representation of data lineage.
Importance in Software Reviews
Enhancing data accuracy
The significance of 'Enhancing data accuracy' in glue data lineage lies in its ability to validate the integrity of data sources and transformations. This aspect focuses on improving the precision and reliability of data within software reviews, ensuring that data-driven decisions are based on accurate information. The key characteristic of 'Enhancing data accuracy' underscores its role in elevating the quality of software evaluations by minimizing data errors and inconsistencies. The unique feature of 'Enhancing data accuracy' is its capacity to enhance the credibility of software reviews through precise data validation, although implementing data accuracy measures may require additional resources.
Facilitating data traceability
'Facilitating data traceability' in glue data lineage plays a vital role in enabling software professionals to track data movements across various systems and platforms. This aspect emphasizes the importance of establishing clear data lineage paths that allow for seamless tracing of data origins and transformations. The key characteristic of 'Facilitating data traceability' highlights its contribution to enhancing transparency and accountability in software reviews by providing a clear roadmap of data flow. The unique feature of 'Facilitating data traceability' lies in its ability to streamline data traceability processes and improve the overall reliability of software evaluations, although complexities in integrating diverse data sources may present challenges in achieving comprehensive data traceability.
Exploring the Dynamics of Glue Data Lineage
In the realm of software reviews, understanding the dynamics of Glue Data Lineage is paramount. This section delves into the intricate complexities of how data lineage operates within the software ecosystem. By exploring this topic, readers can grasp the significance of maintaining accurate data flow and traceability across different platforms. It provides a comprehensive overview of the continuous evolution of information within software systems.
Integration in Software Ecosystem
Role in Data Integration
Data integration plays a crucial role in the seamless operation of software platforms. It acts as a connecting bridge between various data sources, ensuring a harmonious flow of information. The key characteristic of data integration lies in its ability to consolidate and unify disparate data sets, promoting a more cohesive software environment. This feature is highly beneficial for software reviews as it enhances the overall accuracy and reliability of data being analyzed. However, the drawback of data integration lies in the potential complexity it introduces, requiring careful management to prevent data discrepancies.
Impact on System Interoperability
System interoperability is essential for different software systems to communicate and operate cohesively. Data lineage plays a vital role in ensuring that these systems can interact seamlessly, sharing essential information without incompatibility issues. The key characteristic of system interoperability is its capacity to promote a conducive environment for data exchange and collaboration. This feature is particularly popular in software reviews as it enhances the efficiency and effectiveness of data analysis across diverse platforms. Nevertheless, system interoperability may present challenges in terms of ensuring data security and privacy during the exchange process.
Challenges and Solutions
Ensuring Data Consistency
Maintaining data consistency is a critical aspect of data lineage in software reviews. It involves ensuring that data remains accurate and reliable throughout its lifecycle, preventing discrepancies or errors. The key characteristic of data consistency lies in its role in upholding the integrity of information, leading to more robust analytical outcomes. This feature is greatly beneficial in software reviews as it guarantees the trustworthiness of data being evaluated. However, the challenge of ensuring data consistency lies in the continuous monitoring and validation processes required to uphold data quality.
Addressing Data Lineage Gaps
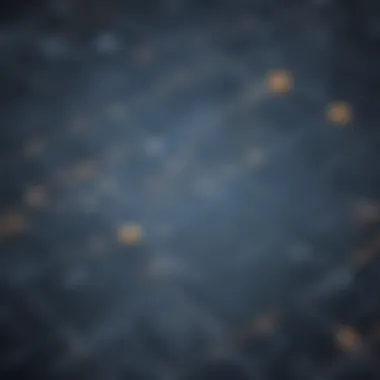
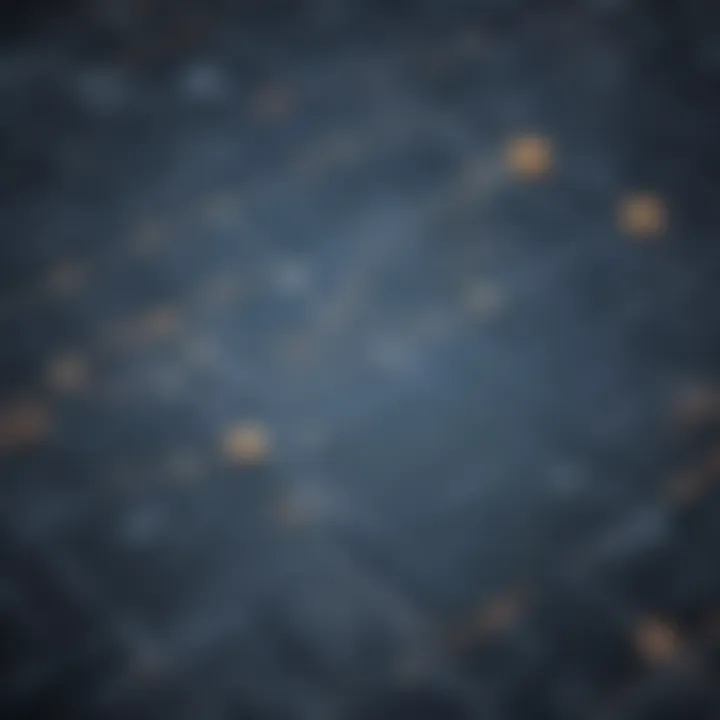
Addressing data lineage gaps is essential for bridging missing links in the flow of data within software systems. It involves identifying and rectifying any breaks or inconsistencies in data lineage, ensuring a complete and transparent data flow. The key characteristic of addressing data lineage gaps is its ability to enhance the accuracy and reliability of data analysis, promoting more informed decision-making processes. This feature is a popular choice in software reviews as it fosters a more comprehensive understanding of the data being assessed. Nonetheless, addressing data lineage gaps may pose challenges in terms of resource allocation and time constraints in resolving complex data discrepancies.
The Significance of Glue Data Lineage in Peer Reviews
In the realm of software reviews, the significance of glue data lineage cannot be overstated. It serves as the backbone, ensuring transparency and accuracy in the evaluation process. Understanding the intricate web of data lineage is crucial for assessing the reliability of information presented in reviews. Glue data lineage plays a vital role in enhancing the credibility and trustworthiness of peer reviews in the software domain.
Transparency in Review Process
Evaluating Data Sources
Evaluating data sources is a critical aspect of the review process. It involves scrutinizing the origin and quality of the data used in software assessments. By discerning the reliability of data sources, reviewers can make well-informed judgments and provide accurate evaluations. The key characteristic of evaluating data sources lies in its ability to verify the authenticity and validity of information, ensuring that reviews are based on credible data. This meticulous evaluation process strengthens the overall integrity of peer reviews, enabling readers to trust the insights provided.
Verifying Data Accuracy
Verifying data accuracy is paramount in upholding the quality of peer reviews. This process entails confirming the correctness and precision of the data presented in evaluations. By verifying data accuracy, reviewers can eliminate errors and inconsistencies, maintaining the authenticity of reviews. The key characteristic of verifying data accuracy lies in its ability to enhance the reliability and credibility of the information shared. While this process may be time-consuming, its contribution to review accuracy is invaluable, making it an essential component of the review process.
Impact on Review Credibility
Building Trust with Readers
Building trust with readers is a fundamental aspect of review credibility. By establishing a sense of reliability and honesty in reviews, trust is cultivated among the audience. The key characteristic of building trust with readers is its capacity to engage and connect with the audience, fostering a positive relationship through transparency and integrity. This approach not only enhances the credibility of reviews but also encourages readers to rely on the insights provided, creating a loyal reader base.
Enhancing Review Authenticity
Enhancing review authenticity is essential for strengthening the integrity of peer reviews. By ensuring that reviews are genuine, original, and free from bias, authenticity is established. The key characteristic of enhancing review authenticity is its ability to provide a unique and unbiased perspective, offering readers a fresh insight into software assessments. While maintaining authenticity may pose challenges, its role in enriching the quality of reviews is indispensable, resonating with readers and elevating the overall standard of peer evaluations.
Ensuring Data Integrity Through Glue Data Lineage
In the expansive realm of software reviews, the topic of Ensuring Data Integrity Through Glue Data Lineage holds profound significance. It serves as the bedrock of reliability in data flow and integrity maintenance. When delving into data lineage, the priorities shift towards maintaining the consistency and accuracy of information traversing through complex software ecosystems. The concrete pillars of Ensuring Data Integrity lie in tracking data transformations and identifying data anomalies.
Maintaining Data Consistency
Tracking data transformations:
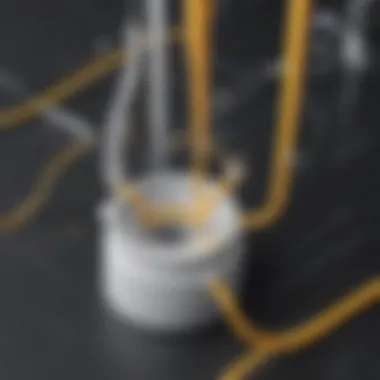
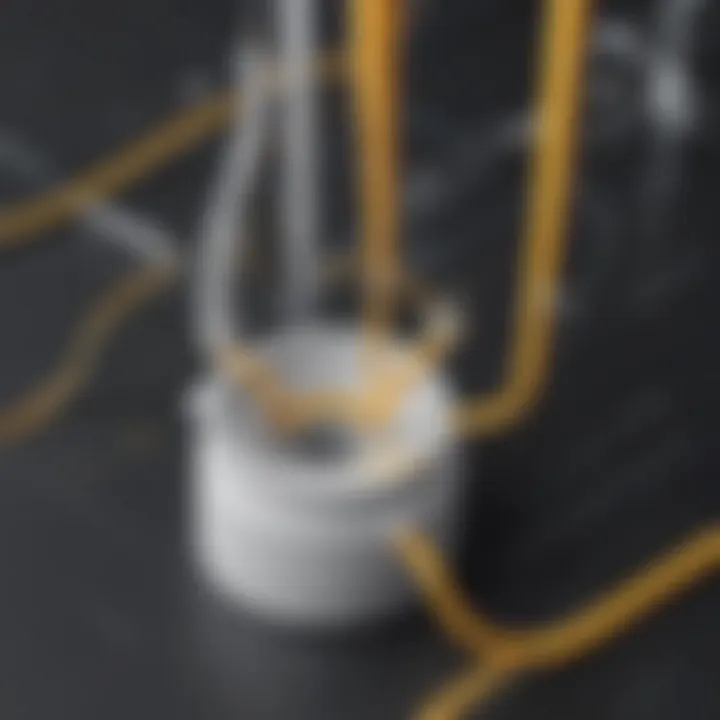
The meticulous process of Tracking data transformations is pivotal in the overarching goal of Ensuring Data Integrity Through Glue Data Lineage. This facet accentuates the ability to monitor and document the metamorphosis of data as it traverses various nodes. The distinctive feature of Tracking data transformations lies in its capacity to offer a transparent audit trail of data evolution, pivotal for maintaining reliability and accuracy. This methodological approach enables seamless identification of data lineage inconsistencies, ensuring operational excellence in data processing.
Identifying data anomalies:
In the pursuit of data integrity, Identifying data anomalies plays a pivotal role in fortifying the data lineage framework. It illuminates deviations or irregularities within the data flow, empowering efficient troubleshooting and enhancement of data accuracy. The essence of Identifying data anomalies lies in its capability to discern subtle irregularities amidst massive data sets, fostering a culture of precision and proactive data management. Despite its benefits, this process may pose challenges in distinguishing between intentional anomalies and inadvertent errors; thus, necessitating a nuanced approach to anomaly detection.
Safeguarding Against Data Loss
Implementing data recovery measures:
Undoubtedly, Implementing data recovery measures represents a critical component in the spectrum of Ensuring Data Integrity Through Glue Data Lineage. This strategic approach focuses on preemptive measures to counteract potential data loss scenarios, safeguarding the integrity and availability of critical information. The intrinsic value of Implementing data recovery measures lies in its proactive stance towards data preservation, ensuring swift mitigation of data loss incidents. However, the complexity of data recovery mechanisms may introduce overhead in terms of resource allocation and operational overhead, requiring a balanced approach to data protection.
Mitigating data risks:
Mitigating data risks emerges as a proactive strategy intertwined with the core objective of Ensuring Data Integrity Through Glue Data Lineage. This process encompasses the identification and mitigation of potential threats to data integrity, fostering a resilient data ecosystem. The salient characteristic of Mitigating data risks lies in its capacity to proactively address vulnerabilities and fortify data protection mechanisms. While it enhances data resilience, the rigorous implementation of risk mitigation strategies may introduce operational constraints and require continuous monitoring and refinement for sustained efficacy.
Future Trends in Glue Data Lineage
In this article, an in-depth exploration of the future trends in glue data lineage is paramount. Understanding these future trends is crucial for IT and software professionals to stay ahead in the ever-evolving technological landscape. The incorporation of automation and AI integration marks a significant shift in data management strategies. By automating processes and integrating AI algorithms, organizations can streamline data lineage processes, leading to improved accuracy and efficiency. This evolution towards automation and AI integration is driven by the rising volume and complexity of data in modern software ecosystems, highlighting the necessity of embracing technological advancements to ensure data reliability and integrity.
Automation and AI Integration
Enhancing data lineage processes
Enhancing data lineage processes through automation plays a pivotal role in streamlining data flow and tracking mechanisms. By automating data lineage processes, organizations can reduce manual errors, improve data accuracy, and enhance overall operational efficiency. The key characteristic of enhancing data lineage processes lies in its ability to automate data tracing, lineage mapping, and impact analysis across diverse data sources. This automated approach not only accelerates data lineage activities but also minimizes the risk of human-induced errors, ensuring data consistency and reliability. Despite its undeniable benefits, organizations must carefully evaluate the scalability and compatibility of automated solutions to ensure optimal integration and seamless operation within their existing software infrastructure.
Predictive analytics in data lineage
Predictive analytics in data lineage revolutionizes how organizations anticipate data flow patterns and potential risks. By leveraging advanced analytics tools, organizations can proactively identify data anomalies, predict data lineage disruptions, and optimize data management strategies. The key characteristic of predictive analytics in data lineage is its ability to forecast future data lineage scenarios based on historical data trends and patterns. This predictive capability empowers organizations to make informed decisions, preempt data inconsistencies, and enhance overall data quality. However, the successful implementation of predictive analytics in data lineage hinges on the availability of high-quality data, robust analytical models, and continuous refinement of predictive algorithms to adapt to evolving data environments. Organizations must also address potential challenges such as data privacy concerns, model accuracy, and interpretability to harness the full potential of predictive analytics in data lineage.
Adaptation to Evolving Software Landscape
Incorporating new data sources
The adaptation to an evolving software landscape necessitates the seamless integration of new data sources into existing data lineage frameworks. By incorporating new data sources, organizations can enrich their data repositories, gain comprehensive insights, and enhance decision-making processes. The key characteristic of incorporating new data sources lies in its ability to expand data diversity, capture emerging data trends, and facilitate cross-platform data integration. This deliberate inclusion of new data sources enables organizations to stay abreast of changing data requirements and leverage diverse data sets to unlock new business opportunities. However, organizations must carefully evaluate the compatibility of new data sources with existing infrastructure, assess data quality and reliability, and establish robust data governance practices to ensure the seamless integration and utilization of diverse data sets.
Agile data lineage frameworks
Agile data lineage frameworks represent a paradigm shift towards dynamic and adaptive data management practices. By embracing agility in data lineage frameworks, organizations can respond promptly to changing data norms, accommodate evolving data structures, and foster iterative data processing methodologies. The key characteristic of agile data lineage frameworks lies in their flexibility, scalability, and responsiveness to changing data dynamics. This agile approach enables organizations to quickly adapt to new data requirements, modify data lineage processes on-the-fly, and efficiently handle data lineage gaps or inconsistencies. While agile data lineage frameworks offer enhanced agility and responsiveness, organizations must ensure robust change management protocols, stakeholder engagement, and data lineage documentation to facilitate smooth transitions and sustainable data lineage practices in dynamic software environments.