Mastering Demand Forecasting with Salesforce
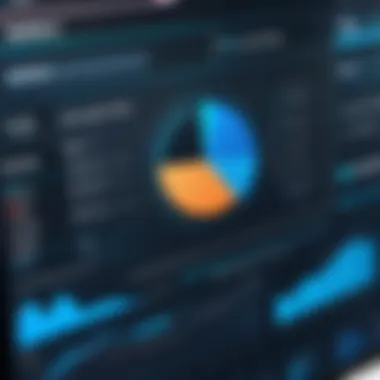
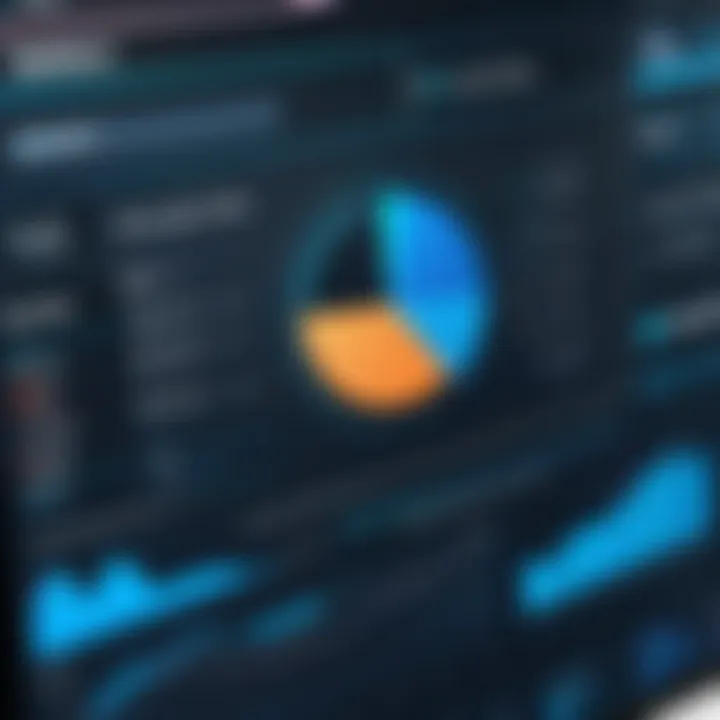
Intro
Demand forecasting is a crucial component for businesses looking to streamline their operations and improve profitability. When delving into this discipline, Salesforce Demand Forecasting emerges as a vital tool that facilitates informed decision-making. Organizations today face an increasingly volatile marketplace; the ability to accurately predict demand can set a business apart from its competitors. Sales forecasting not only encompasses the evaluation of historical sales data but also incorporates future trend analysis, customer behavior, and seasonal fluctuations to refine accuracy.
The intricacies of demand forecasting are further amplified through the lens of Salesforce, a platform renowned for its robust capabilities in managing customer relationships and streamlining various business processes. With the right methodologies in place, Salesforce can transform raw data into actionable insights tailored for distinct business needs. In this exploration, we will dissect the functionalities of Salesforce Demand Forecasting, delve into real-world applications, and discuss methodologies and best practices essential for harnessing its full potential.
Ultimately, the goal is to equip you with a comprehensive understanding of how to leverage Salesforce effectively, boosting operational success and positioning your business for sustained growth in a demanding environment.
Intro to Salesforce Demand Forecasting
Demand forecasting is a cornerstone in the world of business, shaping how organizations manage their resources and plan for the future. In the realm of Salesforce, this practice takes on an even more critical role, driven by its dynamic capabilities that cater to diverse industries. Understanding why effective demand forecasting matters is essential for professionals looking to enhance operational efficiency. When companies predict their demand accurately, they can navigate the rocky waters of supply chain management better, minimize idle stock, and optimize their workforce allocation.
Understanding Demand Forecasting
At its core, demand forecasting involves predicting future customer demand for a product or service. This isn't just a shot in the dark; businesses utilize various methods, both qualitative and quantitative, to enhance the precision of their forecasts. Whether it's analyzing historical sales data or leveraging insights from customer feedback, these forecasts inform strategic decisions across the board. Imagine a retail business that can anticipate which products will fly off the shelves during holiday sales or a manufacturing company that plans its production schedules effectively.
In a world where change is the only constant, having a reliable demand forecast enables businesses to adapt quickly. For instance, consider a tech company that launches a new gadget. By predicting demand accurately, it can ramp up production without overextending resources. Companies that master this art find themselves several steps ahead of their competition, avoiding the pitfalls of surplus inventory or missed sales opportunities.
The Role of Salesforce in Demand Planning
Salesforce transforms the landscape of demand forecasting by offering robust tools that streamline this process. With features like Salesforce Analytics Cloud, organizations access powerful data visualization resources that help simplify complex data sets. The platform allows for real-time data analysis, making it easier to spot trends or anomalies that require immediate attention.
Moreover, Salesforce integrates smoothly with various data sources, providing a holistic view of demand signals. By tapping into various customer touchpoints and sales information, organizations can build a comprehensive picture of current trends. It’s akin to piecing together a puzzle, where each piece adds to the clarity of the entire image.
"Having an effective demand forecast is like having a map in uncharted territory; it guides your sails and sets your course."
Through the predictive analytics functions embedded within the Salesforce environment, businesses can create sophisticated models that not only forecast demand but also evaluate potential market disruptions. This foresight allows for agile responses to shifts in consumer behavior or purchasing patterns, mitigating risks that often accompany uncertainty. By embracing Salesforce for demand planning, companies unlock a pathway to better decision-making processes, resulting in decreased costs, improved customer satisfaction, and ultimately, higher returns on investment (ROI).
In sum, the integration of Salesforce into demand forecasting helps organizations turn assumptions into actionable insights. This is pivotal not only for immediate decision-making but for long-term strategic planning as well.
Core Principles of Demand Forecasting
Understanding the core principles of demand forecasting is essential for businesses aiming to achieve precise sales predictions and optimize their resource allocation. At its heart, demand forecasting is about estimating future customer demand based on historical data and various influencing factors. This isn’t just a shot in the dark; it encompasses both art and science, blending qualitative insights with raw data. By getting a grip on these principles, businesses can make more informed decisions about inventory levels, staffing, and new product launches.
Qualitative vs Quantitative Methods
When diving into demand forecasting, one must grapple with the choice between qualitative and quantitative methods. Each approach has its own merits and can be leveraged depending on the context or nature of the business.
Qualitative methods rely heavily on subjective judgment and experience. These methods often include expert opinions, surveys, and market research. They shine brightly, particularly in situations where there is little to no historical data available. For instance, if a company is launching a groundbreaking product that has no past market behavior to reference, gathering insights from experienced industry stakeholders can offer invaluable perspectives. However, this approach can sometimes be seen as less reliable due to its inherent subjectivity.
Quantitative methods, on the other hand, use mathematical models and historical data to predict future demand. These include time series analysis, regression analysis, and machine learning algorithms.
- Time series analysis looks at patterns over time, identifying trends and seasonal variations, thus allowing businesses to predict future sales based on past performances.
- Regression analysis can establish the relationships between demand and external variables, such as pricing changes or economic shifts.
While quantitative methods offer a robust framework grounded in data, they are not foolproof. Changes in market conditions, consumer preferences, or sudden disruptions can throw predictions off course.
Ultimately, a hybrid approach often yields the best results. Mixing qualitative insights with quantitative rigor can help paint a more complete picture, allowing businesses to calibrate their strategies with greater accuracy.
Data Sources for Accurate Forecasting
Data quality is the backbone of demand forecasting. Relying on outdated, incorrect, or irrelevant information can lead a company down a slippery slope. Therefore, knowing where to source reliable data is crucial.
Internal data sources often offer a reliable starting point, as they include:
- Sales history: Past sales data helps identify patterns and seasonal fluctuations that can inform future predictions.
- Customer relationship management (CRM) data: Insights from CRM systems, such as customer preferences and behavior, can signal potential buying trends.
- Inventory levels: Understanding current stock can help inform decisions about restocking or clearing out goods before they go stale.
External data sources also play a significant role in enriching forecasts. These include:
- Market research reports: Published studies provide insight into market trends and shifts in consumer behavior.
- Economic indicators: Changes in economic conditions, such as unemployment rates or inflation, can impact consumer spending.
- Social media analytics: Monitoring conversations and sentiments on platforms like Facebook or Reddit can provide real-time insights into changing customer opinions and preferences.
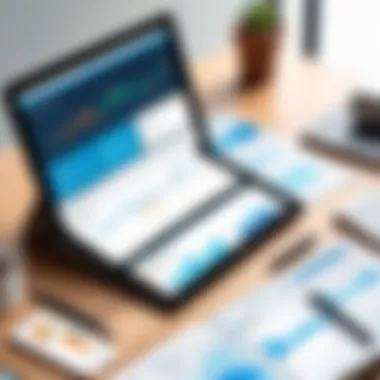
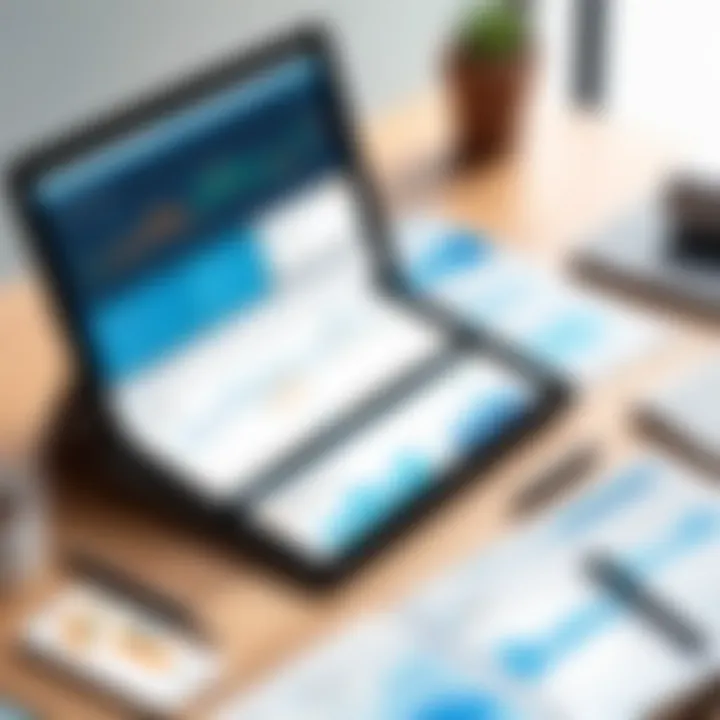
Integrating diverse data sources allows organizations to backstop their forecasts with a wealth of relevant information. This multifaceted approach not only boosts the accuracy of predictions but also aids in better strategic planning. Remember, a good forecast is built on solid data foundations.
Salesforce Features Supporting Demand Forecasting
Salesforce offers a range of powerful features that streamline demand forecasting processes. These tools not only enhance accuracy but also improve collaboration among teams. Understanding how to leverage these capabilities can propel businesses toward more reliable planning and operational success.
Salesforce Analytics Cloud
At the core of effective demand forecasting is data analysis, and Salesforce Analytics Cloud stands as a pivotal resource in this regard. This cloud-based platform provides organizations the ability to visualize and interpret data in real-time. With intuitive dashboards and robust reporting tools, users can easily navigate complex datasets to spot trends and make informed decisions. Here are a few benefits that stand out:
- Enhanced Data Visualization: The platform helps in transforming raw data into easy-to-understand visuals, aiding quicker decision-making.
- Real-time Insights: Immediate access to analytics allows businesses to react swiftly to market changes, rather than waiting for periodic summaries to inform strategy.
Due to its integration with the rest of the Salesforce ecosystem, it naturally pulls in customer, sales, and inventory data, making forecasting a more cohesive and holistic approached.
Predictive Analytics Functions
Predictive analytics is another cornerstone of Salesforce’s demand forecasting capabilities. Here, machine learning models analyze historical data to project future demand trends. These predictive functions help businesses answer crucial questions:
- What will the demand look like for our products next quarter?
- How can we optimize inventory to avoid shortages or overstock?
With tools like Salesforce Einstein, organizations apply predictive models effortlessly, leading to more accurate forecasts and better business outcomes. Key functionalities include:
- Automated Forecasting: By automating forecasts based on past patterns, it saves time and reduces human error in manual analysis.
- Scenario Planning: Users can simulate various business conditions to assess their potential impact on demand, enabling proactive adjustments.
Grassroots understanding of such features helps companies not only predict demand but also adapt strategies dynamically as new patterns emerge.
Integration with Other Salesforce Tools
Integration is where Salesforce truly shines, as various tools work together to create a seamless demand forecasting experience. Whether it is connecting with Sales Cloud for sales data or Service Cloud for customer feedback, this interconnectedness allows for a comprehensive understanding of factors influencing demand. Here are the considerations:
- Holistic Data Access: With integration, users have all the relevant data at their fingertips, enabling more informed decisions based on both sales history and customer interactions.
- Improved Collaboration: Teams using different Salesforce tools can easily share insights and forecasts, fostering a collaborative atmosphere that enhances forecasting accuracy.
By utilizing the full suite of Salesforce tools, businesses build a more robust approach to demand forecasting that can adapt to an ever-changing market landscape.
"Effective demand forecasting through Salesforce ultimately leads to better customer satisfaction and increased profitability."
As organizations focus on leveraging these Salesforce features, they lay the groundwork for a more resilient and forward-thinking business model.
Methodologies for Effective Demand Forecasting
Effective demand forecasting hinges on employing the right methodologies that align with an organization’s unique needs. Different techniques can provide insights into future demand patterns, which is crucial for decision-making processes in businesses of all sizes. When properly implemented, these methodologies enable firms to optimize inventory, enhance customer satisfaction, and boost profitability. Incorporating well-rounded approaches ensures that both internal data and external factors, like market trends, are accounted for. In this section, we’ll explore two prevalent methods used in Salesforce demand forecasting: Time Series Analysis and Causal Models.
Time Series Analysis
Time series analysis is a statistical technique that assesses data points collected at regular intervals over time. This method is especially valuable in demand forecasting because it allows organizations to understand trends, cycles, and seasonal variations. One significant advantage of time series analysis is its ability to sift through historical data, revealing patterns that can indicate future demand.
Key elements of Time Series Analysis include:
- Trend Identification: Recognizing whether demand is increasing, decreasing, or remaining stable over time.
- Seasonality: Understanding fluctuations that occur at specific intervals, such as higher sales in December for retail businesses.
- Cycle: Analyzing longer-term fluctuations that may not follow a fixed schedule.
Through Salesforce’s advanced analytics tools, businesses can harness the power of time series analysis. For example, a company can visualize demand data through Salesforce dashboards to help stakeholders easily identify trends.
"Historical data isn't just numbers; it's a treasure trove of insights waiting to be harnessed for future success."
Causal Models
Causal models adopt a different approach from time series analysis by focusing on the relationships between different variables to predict demand. This methodology posits that various factors, such as economic indicators, marketing strategies, and changes in pricing, directly influence customer demand. By understanding these relationships, businesses can effectively tailor their strategies to maximize sales.
Consider the following components of causal models:
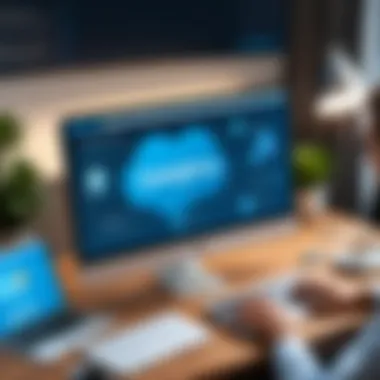
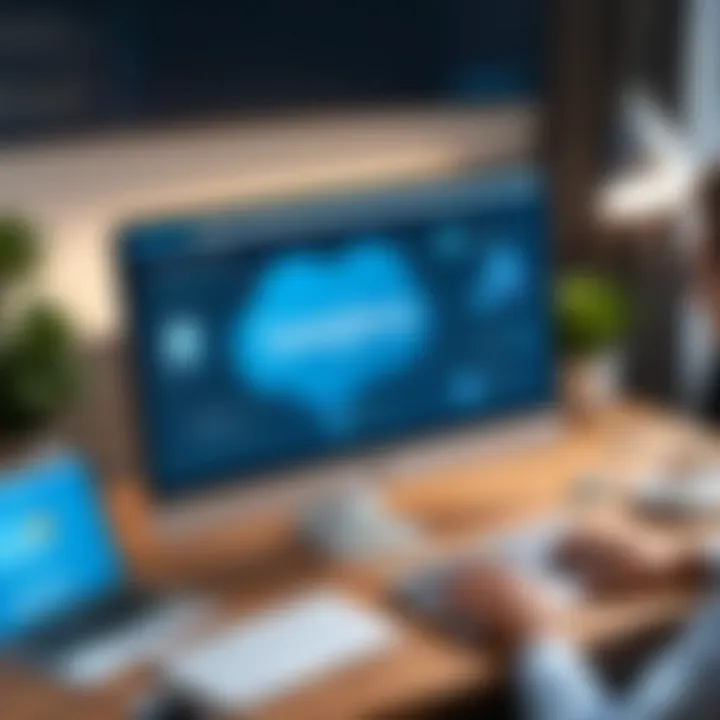
- Independent Variables: These are the factors that may influence demand, like advertising spend or economic conditions.
- Dependent Variable: Demand itself, which is expected to change in response to the independent variables.
- Model Equation: Often modeled using regression analysis, where relationships between variables are mathematically expressed.
Causal models can also be integrated into Salesforce with its AI-powered predictive capabilities, allowing companies to forecast demand based on real-time data. By understanding how varying factors impact demand, businesses can make more informed decisions on everything from resource allocation to strategic planning.
Incorporating both time series analysis and causal models into Salesforce can create a synergistic effect. When used together, these methodologies paint a detailed picture of demand forecasting, guiding organizations towards more precise and profitable outcomes.
Best Practices in Salesforce Demand Forecasting
Salesforce Demand Forecasting is not just a fancy tool, but it’s an essential framework for businesses aiming to streamline their operations and make data-driven decisions. The practices surrounding it can significantly influence how well a company anticipates demand, manages inventory, and ultimately impacts customer satisfaction. Getting it right is paramount, and understanding how to implement best practices can mean the difference between two entities in the same industry—one flourishing and the other floundering.
Engaging Stakeholders for Input
Engagement of stakeholders isn’t just ticking a box on a checklist. It’s about crafting an inclusive environment where their insights can shape forecasts meaningfully. Stakeholders often hold invaluable insights into market trends and customer preferences that numbers might miss.
Considerations for Engagement:
- Cultivate Open Communication: Create channels where stakeholders can voice their opinions without fear. Regular brainstorming sessions can spark creative solutions and insights.
- Vary Perspectives: Involve individuals from different departments such as marketing, sales, and customer service. Their unique viewpoints can yield richer data aggregation.
- Establish Trust: When stakeholders see that their input is considered in forecasts, they’ll be more likely to participate actively in the future.
This collective brainstorming not only improves the accuracy of forecasts but also fosters a sense of ownership among stakeholders.
Iterative Forecast Process
Demand forecasting is not a one-and-done deal. The iterative process allows for refinements based on real-time data and changing variables. Salesforce offers functionalities that support this cycle, enabling businesses to adapt quickly to new information.
Steps in the Iterative Process:
- Initial Data Analysis: Start by gathering historical data — past sales figures, seasonal variations, and market trends.
- Draft Forecast: Leverage predictive analytics to create a preliminary forecast.
- Stakeholder Review: Share the forecast with stakeholders for feedback, insights, and potential inaccuracies.
- Adjustments: Make modifications based on feedback and new data inputs.
- Execute and Monitor: Once executed, keep an eye on real-time results to glean insights for the next cycle.
This continuous improvement cycle will maximize accuracy over time and aligns closely with Salesforce's agility in managing data.
Monitoring and Adjusting Forecasts
Even the most robust forecasting methods can falter if the required adjustments aren’t made in response to new information. The importance of monitoring cannot be overstated; it acts as a safety net ensuring that forecasts remain aligned with reality.
Key Monitoring Techniques:
- Regular Review Meetings: Plan monthly or quarterly meetings dedicated strictly to review forecasting performance against actual figures.
- Utilize Dashboards: Implement Salesforce dashboards to visualize performance metrics, allowing quick identification of discrepancies.
- Feedback Loops: Establish mechanisms for feedback where employees can report anomalies in the forecast versus actual sales.
%> "Adapting swiftly to changes in the market is not just good practice; it’s a necessity in today’s business climate."
With these monitoring measures in place, companies can quickly pivot in response to shifts in demand, thus maximizing the effectiveness of their forecasting efforts.
In summary, adhering to best practices in Salesforce Demand Forecasting is about honing a process that actively engages stakeholders, fosters an iterative approach to forecasting, and is vigilant in monitoring performance. These strategies collectively enhance the accuracy and reliability of forecasts, enabling businesses to meet customer needs more effectively.
Challenges in Demand Forecasting
Understanding the challenges in demand forecasting is a fundamental part of maximizing the effectiveness of Salesforce's capabilities. After all, if the underpinnings of your forecasts are weak, the outcomes may not only miss the mark but can also lead to misguided strategies and wasted resources. This section sheds light on two primary hurdles often encountered: data quality and integrity issues, and the unpredictable nature of market volatility. Recognizing and addressing these challenges is essential for achieving accurate predictions and enhancing overall business performance.
Data Quality and Integrity Issues
When it comes to forecasting demand, the phrase "garbage in, garbage out" rings particularly true. The essence of effective forecasting sits squarely on data quality. If the data fed into Salesforce lacks accuracy or completeness, the forecasts produced may be more fiction than fact. Here are several aspects to consider:
- Inconsistent Data Formats: Data migration often leads to discrepancies. If the sales data generated by different sources is not standardized, it can create confusion and compromise analytic integrity.
- Outdated Information: In a fast-paced sales environment, using stale data throws a wrench in your forecasting efforts. Timely updates are key to maintaining relevance in predictions.
- Human Error: Errors during data entry or processing can easily skew results. Regular audits can help mitigate these risks but require a dedicated effort.
Addressing these issues isn’t just about making improvements but rather ingraining data vigilance into the company culture. Teams should prioritize setting clear guidelines for data entry and regularly check for inconsistences. Moreover, tools within Salesforce like Data.com can help cleanse data before it's analyzed, enhancing the reliability of forecasts considerably.
Market Volatility and Its Effects
Market volatility can feel like trying to hit a moving target. Various factors like economic shifts, competitive pressures, and even seasonal fluctuations can dramatically alter demand. Understanding these dynamics can be tricky but is crucial for accurate forecasting. Here are a few elements to keep in mind:
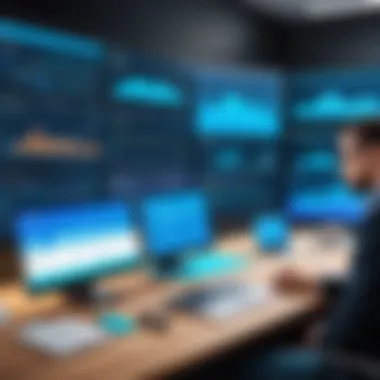
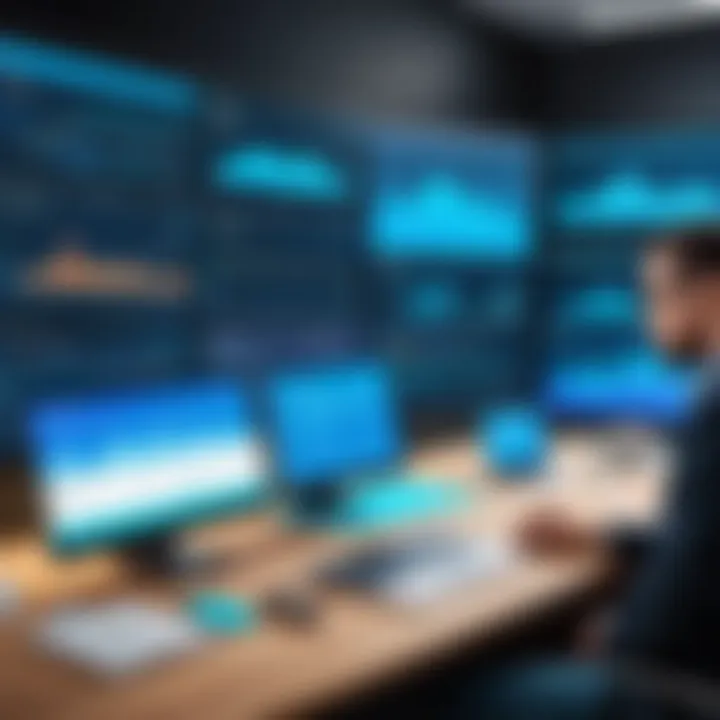
- Economic Influence: Economic downturns or booms can impact purchasing behavior unpredictably. This factor requires continuous monitoring to adapt strategies accordingly.
- Consumer Trends: Shifts in consumer preferences can happen overnight. What was trending last season may flop now, affecting this quarter's forecasts severely.
- Global Events: Events like pandemics or geopolitical tensions can create ripples throughout supply chains and consumer confidence alike, making predictions almost a shot in the dark.
As market environments remain fluid, using Salesforce's robust analytical tools can aid businesses in adapting strategies in real-time. Predictive models should be regularly refined based on the latest data inputs. Implementing flexibility allows businesses to pivot and re-assess when faced with sudden changes in demand.
Real-World Applications of Salesforce Demand Forecasting
In a world where businesses are always hustling to stay ahead, understanding the practical aspects of Salesforce Demand Forecasting is crucial. Applying theory to real-world scenarios reveals the undeniable impact that accurate demand forecasting has across industries. This section dives into the vital insights derived from real-world applications, showcasing how Salesforce serves as a catalyst for achieving operational efficiency and enhancing decision-making processes.
Salesforce Demand Forecasting isn't just a theoretical exercise; instead, it equips businesses with tools that allow them to anticipate customer needs, manage inventory levels, and ultimately drive profitability. By leveraging demand forecasts, organizations can prevent overstocking or stockouts, both of which can be financially draining. The benefits extend beyond mere numbers—aligned demand forecasting can significantly elevate customer satisfaction by ensuring that products are in stock, thereby nurturing loyalty.
Case Studies and Success Stories
Take, for instance, a mid-sized retailer that harnessed the power of Salesforce. By integrating demand forecasting capabilities through Salesforce Analytics Cloud, they transformed their inventory management strategy. The retailer had struggled with excess stock of seasonal items, leading to excessive markdowns. Once they began to utilize Salesforce's data-driven approach, they analyzed purchasing trends and historical sales data, allowing them to forecast demand accurately.
"Adopting Salesforce Demand Forecasting was like flipping a light switch. Suddenly, we could see operational efficiencies lighting up the room, and our inventory issues began to fade."
— Retail Operations Manager
Another notable example can be found within the tech industry. A prominent software company used Salesforce to tailor its demand forecasting towards product launches. By collaborating with various departments to share insights and real-time updates regarding market trends, they successfully aligned their supply chain operations. Instead of facing a surge in demand that left the customer waiting, they achieved timely product availability, enhancing customer satisfaction and fostering brand loyalty.
Industry-Specific Applications
The relevance of Salesforce Demand Forecasting spans multiple sectors, each with distinct applications:
- Retail: Companies are using the platform to assess consumer buying patterns, parse seasonal trends, and optimize their inventory accordingly.
- Manufacturing: Facilities harness forecasting to align production schedules with projected sales figures, ensuring a smooth operation without surplus production.
- Healthcare: With ever-changing patient demands, healthcare providers utilize Salesforce to predict necessary resources, staffing, and equipment to ensure quality patient care.
- Restaurant: Food and beverage establishments apply demand forecasting to avoid food waste and manage staffing during peak times effectively.
In a nutshell, whether you're in retail or healthcare, the real-world applications of Salesforce Demand Forecasting demonstrate its pivotal role in navigating the unpredictable waters of today’s marketplace. By adopting these methodologies, companies reap the rewards of precision, reliability, and customer loyalty—demonstrating that the shift toward informed forecasting is not merely advantageous but essential for sustained success.
The Future of Demand Forecasting with Salesforce
As businesses navigate an increasingly complex and fast-paced market landscape, the importance of effective demand forecasting cannot be overstated. Within this framework, Salesforce is poised to play a critical role in shaping the methodologies and technologies that will define demand forecasting in the coming years. In this section, we will explore the future of demand forecasting through Salesforce's lens, focusing on key elements such as emerging technologies and the growing influence of artificial intelligence.
Emerging Technologies Impacting Demand Forecasting
The landscape of demand forecasting is evolving rapidly with the advent of new technologies. A few key trends are worth observing:
- Big Data Analytics: The sheer volume of data available today is staggering. Businesses can now collect data from numerous touchpoints, including customer interactions, social media engagement, and transaction records. This data can be harnessed to reveal patterns and trends that were previously too intricate to analyze thoroughly.
- IoT Integration: With the Internet of Things, businesses can access real-time data from connected devices. For example, IoT sensors in the supply chain can provide instant updates on stock levels, helping to enhance accuracy in demand forecasting. This real-time capability can significantly expedite responses to market changes.
- Cloud Computing: The move to cloud platforms is revolutionizing how businesses manage their data. Salesforce's cloud-based ecosystem allows for seamless integration and scalability, ensuring that organizations can optimize their forecasting processes more efficiently.
"Harnessing emerging technologies is akin to having a crystal ball; it allows businesses to foresee shifts in demand and adapt accordingly."
These emerging technologies collectively enable a more dynamic approach to demand forecasting, empowering businesses to respond more nimbly to consumer needs and market fluctuations.
The Increasing Role of Artificial Intelligence
Artificial Intelligence (AI) stands at the forefront of transforming demand forecasting practices. Its role extends far beyond simply analyzing data; it is about making sense of complex datasets and predicting future trends.
- Predictive Modeling: AI can help construct predictive models that are far more accurate than traditional methods. By learning from historical data, AI algorithms can forecast future demand with remarkable precision. In practical terms, if you run a retail operation, AI could analyze past sales data alongside external factors like economic indicators or seasonal shifts to predict your upcoming needs more reliably.
- Automating Insights: Instead of manual analysis, AI enables the automation of insights, saving time and reducing the margin for error. This allows staff to focus on strategy and decision-making rather than getting lost in spreadsheets.
- Personalization of Demand Plans: AI facilitates the personalization of demand forecasting, providing insights tailored to specific customer segments. For instance, a company could receive recommendations about which products are likely to appeal to certain demographic groups, based on robust data analysis.
The integration of artificial intelligence into the forecasting process means that predictions can become not only more accurate but also more quickly actionable. As a result, businesses can optimize inventory, minimize waste, and enhance customer satisfaction.
Epilogue
In wrapping up our exploration of Salesforce Demand Forecasting, it's imperative to underscore the topic's multifaceted importance. Demand forecasting stands as a linchpin in operational success, enabling businesses to navigate the murky waters of market fluctuations and customer behavior with greater precision. As organizations face increasing pressure to operate efficiently while retaining flexibility, the insights derived from effective demand forecasting become invaluable.
The engagement with Salesforce tools elevates this practice by providing robust analytics and seamless integrations, facilitating informed decision-making. Companies can harness these insights to anticipate demand, maintain optimal inventory levels, and ultimately enhance customer satisfaction. This leads us to consider essential elements such as collaboration across departments, the significance of data integrity, and the necessity for continuous learning and adaptation in implementing forecasting strategies.
Summation of Key Insights
- Holistic Approach: The article articulates that demand forecasting is not merely a technical exercise but necessitates a collaborative and strategic approach across various departments, ensuring that all stakeholders contribute their unique perspectives.
- Leveraging Technology: Salesforce's capabilities serve as a digital backbone, allowing organizations to integrate various data sources for synthesized insights, reducing the chance for discrepancies or oversight during the forecasting process.
- Adaptive Strategies: The discussion emphasizes the need for businesses to adopt adaptive strategies in their demand forecasting methodologies, taking into account external factors like market volatility and shifts in consumer preferences.
By distilling these key themes, we see a route forward where businesses can leverage technology and collaborative insights for better demand prediction and operational efficiency, setting the stage for sustainable growth.
Final Thoughts on Implementation
As businesses look to implement effective demand forecasting strategies using Salesforce, it's crucial to embrace a mindset geared towards adaptability and continuous improvement. The road to successful implementation may not be devoid of challenges, yet acknowledging them upfront prepares organizations to face hurdles head-on.
Start with a clear framework and objectives, ensuring that everyone involved understands the purpose and expected outcomes of the forecasting initiative. Regular training and engagement with these tools will foster a culture of data-driven decision-making.
Also, it's wise to routinely evaluate the forecasting models and processes. An iterative approach will allow businesses to fine-tune their methodologies based on real-world outcomes and changes in the market landscape.