Exploring the Data Quality Lifecycle for Better Insights
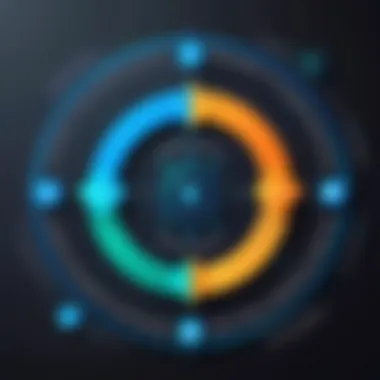
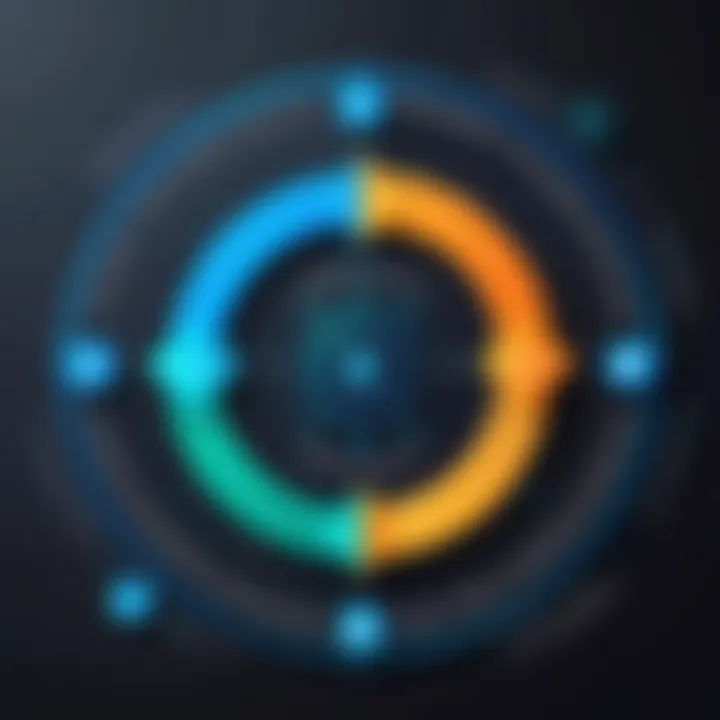
Intro
In the ever-evolving landscape of data management, grasping the data quality lifecycle is fundamental for organizations aiming to make smart, data-driven choices. This lifecycle is not just about acquiring data; it encompasses a continuous process that requires diligent attention through each phase, ensuring that businesses have access to data they can trust. When you consider how organizations from various sectors depend on accurate data to steer their strategies, it becomes evident that a robust understanding of this lifecycle is indispensable.
The data quality lifecycle begins with data creation and collection. This initial phase sets the tone for everything that follows. Without solid and correctly gathered data, later steps can be compromised. After the basics are in place, attention turns to maintenance, where the focus lies on keeping the data clean, relevant, and accurate. Finally, as the segment of data loses its relevance, there's the retirement phase — a often overlooked, yet crucial, part of the process.
As we navigate through the stark realities of this lifecycle, we will examine important touchpoints, highlighting why understanding each segment is critical. This exploration is not merely theoretical; it serves to empower IT professionals, data experts, and decision-makers in a practical sense. By grasping this framework, organizations can build trustworthy data foundations, ultimately enhancing operational efficiency and decision quality.
A thorough discussion will be provided on how these different components interconnect and why they matter, shedding light on the strategies organizations can adopt to secure the integrity of their data. It's about more than just improving numbers; it’s about building a data-centric culture that values quality and reliability.
Software Overview
When discussing the tools and systems that can facilitate data quality management, understanding their features and functionalities is key.
Features and functionalities
Various software solutions are designed to assist organizations in managing data quality. These tools typically come packed with functionalities such as data profiling, cleansing, enrichment, and validation. For example, a data profiling tool might help identify anomalies in datasets by providing insights into data patterns and distributions. In contrast, data cleansing features can automatically correct mistakes or inconsistencies, making the data more reliable.
Pricing and licensing options
Pricing can vary widely based on the depth of features offered and the scale of deployment. Some may operate on a subscription basis, while others might employ a one-time licensing fee. This flexibility allows organizations of all sizes to choose a plan that aligns with their goals and budget.
Supported platforms and compatibility
Support for diverse platforms is another important aspect. Leading software in this domain often integrates seamlessly with existing databases, cloud services, and analytics tools, ensuring that companies can leverage their current investments in technology.
Real-world Applications
Understanding how data quality software makes a tangible impact across industries is vital.
Industry-specific uses
In healthcare, for instance, maintaining data accuracy can directly affect patient outcomes. Erroneous patient data may lead to critical treatment delays. In finance, data quality dictates compliance with regulations, affecting an organization's reputation and bottom line. Retailers similarly rely on clean data to tailor their offerings and optimize stock levels, highlighting the lifecycle's expansiveness.
Case studies and success stories
Take the case of a leading airline that adopted a data quality management system for streamlining customer feedback. The results were striking: they achieved a 20% decrease in response times by ensuring that customer queries were routed correctly and efficiently managed. This single adjustment not only improved customer satisfaction but also reflected positively on their overall operational metrics.
Updates and Support
Finally, let's consider the importance of ongoing updates and support.
Frequency of software updates
Software must evolve constantly alongside new data regulations and technological advancements. Regular updates ensure that your tools remain relevant and effective in combating emerging data quality challenges.
Customer support options
Comprehensive customer support is also a must. Whether through direct support teams, community forums, or user resources, having access to help when needed can bolster an organization’s confidence in managing their data effectively.
These discussions serve multiple purposes: they elucidate the crucial stages of data management while also driving home the point that excellent data quality isn't merely about minimizing errors—it's about facilitating informed decision-making that drives growth.
Intro to Data Quality Lifecycle
Defining Data Quality
Data quality isn't simply about having the right numbers on a page. It's a more nuanced concept. To put it simply, data quality can be defined as the condition of a dataset based on various attributes, such as accuracy, completeness, reliability, and relevance. Imagine you’re trying to build a house. If you start with subpar materials, the end product will surely reflect those shortcomings.
In the context of data, if the information collected is incomplete or riddled with inaccuracies, any insights derived from it will ultimately be skewed. For example, if a company's customer database is filled with old addresses and incorrect contact numbers, any marketing initiative based on that data will likely miss the mark.
Significance of Data Quality Lifecycle
The significance of the data quality lifecycle cannot be overstated. In a world that thrives on data-driven strategy, ignoring the lifecycle puts organizations in jeopardy. Not only does it help identify the various stages data undergoes, but it also aids in recognizing how errors can propagate through these stages.
When you have clarity on this lifecycle, you can implement checks and balances at each phase, minimizing the risk of errors and ensuring a smoother process overall. For instance, consider a company rolling out a new product. By paying close attention to the data quality lifecycle, they can gather accurate market research, leading to more targeted advertising and successful product launch.
Moreover, this lifecycle encourages a proactive mindset. Instead of merely reacting to problems as they arise, organizations can adopt strategies that funnel resources toward prevention. This way, the focus shifts from cleaning up messes to crafting systems designed to yield quality data from the get-go.
"Data quality is not just a technical issue; it’s a business asset that can make or break an organization’s success.”
To capture the essence of the data quality lifecycle, it's crucial to recognize its role in fostering a culture focused on quality. Engaging in this lifecycle also enhances accountability among team members, ensuring that everyone contributes toward maintaining data integrity.
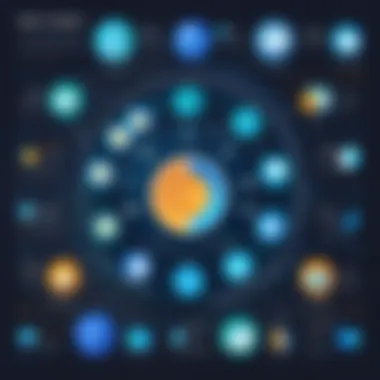
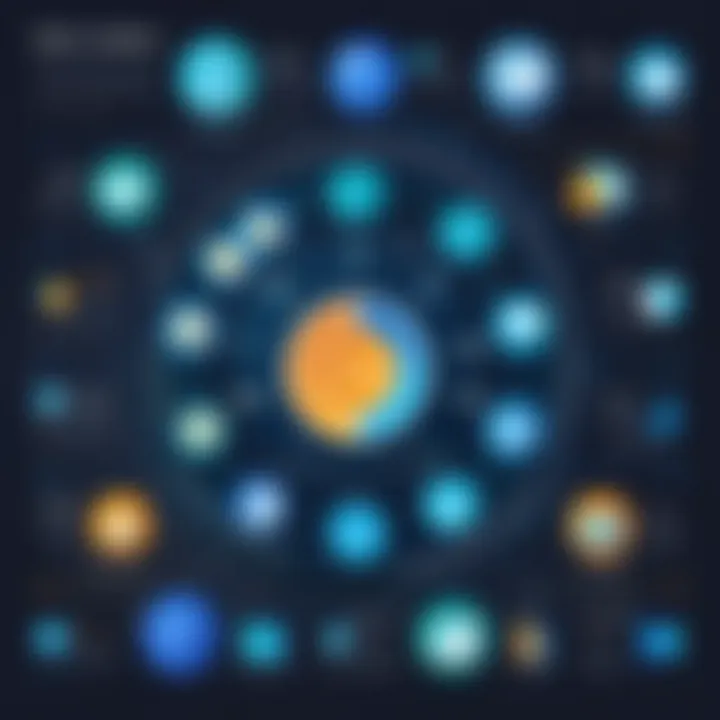
In essence, the data quality lifecycle is like a guidebook that reveals the intricacies of data management. By embracing this concept, organizations not only safeguard themselves against future pitfalls but can also steer their operations toward more informed, data-backed decisions. This comprehensive understanding sets the stage for effectively navigating the stages that follow, shaping the future of business processes.
Stages of the Data Quality Lifecycle
Understanding the stages of the data quality lifecycle is key to mastering how organizations manage their data assets. This framework comprises several vital phases, each strategically designed to uphold data quality. By recognizing and fine-tuning each stage, businesses stand to benefit from enhanced decision-making, increased operational efficiency, and ultimately, a more trustworthy data foundation.
Data Creation
Methods of Data Generation
Data generation is where it all begins. It involves transforming raw materials—whether these are human inputs, sensor readings, or automated processes—into relevant data sets. Prospective methods of data generation can include surveys, IoT devices, and even artificial intelligence, each contributing a rich tapestry of information. They're often chosen for their ability to capture data efficiently while minimizing human intervention.
One compelling characteristic of these methods is their scalability; for instance, deploying IoT devices can gather vast amounts of data over time, which can be particularly crucial for real-time analysis. However, there's always a double-edged sword. The more data we collect, the greater the responsibility to manage it appropriately as it can easily spiral into overwhelming noise without a robust framework.
Key Considerations
When diving into data creation, it’s essential to consider factors like the purpose of the data, its intended use, and how it will be validated. These factors contribute directly to whether the generated data will serve its intended purpose effectively.
One vital aspect of these considerations is relevant context. Data without a frame can lead to misguided analyses. Moreover, being overly focused on quantity risks overlooking the quality. Businesses often find themselves in a precarious position, having amassed a wealth of information that is ultimately unusable.
Data Collection
Sources of Data
Data sources can come in various forms, such as internal systems, third-party providers, or public datasets. Navigating these sources takes skill and foresight. Choosing the right sources ensures a steady stream of relevant, timely, and accurate data.
A significant advantage of leveraging diverse sources is the opportunity to triangulate data. By obtaining the same information from multiple venues, organizations can better verify its authenticity, thus elevating the overall reliability of their datasets.
Yet, it’s not all smooth sailing. Each source may come with its own set of limitations, including potential bias, outdated information, or even legal restrictions on how data can be used, posing challenges that must be managed earnestly.
Best Practices for Collection
Utilizing data collection best practices ensures that the gathered data is both representative and accurate. Some essential practices include systematic sampling, consistent methods, and frequent audits of the data collection process.
These practices benefit organizations by allowing them to glean insights more reliably and accurately. However, they also require a commitment of time and resources. The balance between diligence in collecting data and the nuance of operational efficiency can be quite delicate.
Data Storage
Data Management Systems
Data management systems form the backbone of how collected data is stored, retrieved, and managed. Choosing the right system can make all the difference between a smooth operation and a convoluted mess. Popular systems often include relational databases like MySQL, NoSQL alternatives, or even cloud-based solutions such as Microsoft Azure.
The challenge is in identifying the right match for organizational needs. With so many options available, organizations sometimes grapple with selecting a system that meets scalability and performance requirements without breaking the bank.
Storage Techniques
Storing data isn’t merely about putting it somewhere for safekeeping; it requires foresight into retrieval strategies, backup mechanisms, and data segmentation. Techniques such as partitioning or archiving can ensure the integrity of actively used data while keeping the system responsive.
It's vital for organizations to understand that improper storage techniques can lead to data retrieval difficulties or even loss over time, making effective storage an absolute necessity within this lifecycle.
Data Processing
Transforming Raw Data
Once data has been collected and stored, processing is where the real magic happens. It transforms raw data into meaningful insights through cleaning, aggregating, and analyzing.
This stage is crucial for making information actionable. It allows organizations to identify trends, anomalies, and correlations that can inform decision-making. Yet, the complexity of this process often poses challenges as raw data may present inconsistencies or require elaborate configurations to extract usable insights.
Common Processing Challenges
While processing is essential, it’s riddled with potential pitfalls. Common challenges include data alignment issues, processing speed limits, and software compatibility troubles. Businesses often encounter bottlenecks in workflows, leading to delays in actionable insights.
Understanding these challenges up front can help organizations create contingency plans to ensure that the data processing phase is as smooth and efficient as possible.
Data Maintenance
Ensuring Data Accuracy
Maintaining data accuracy is a concerted effort that involves consistently checking, validating, and updating datasets. This ongoing challenge is paramount as inaccurate data can mislead decisions and strategies.
A key characteristic of successful data maintenance is establishing a routine for audits and validation processes, thereby mitigating errors. However, it can’t be ignored that this routine demands a significant investment of time and human resources, which can sometimes pose a barrier for smaller organizations.
Updating and Cleaning Data
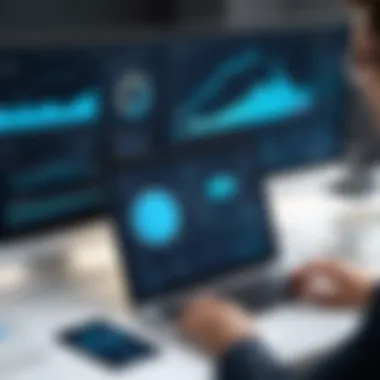
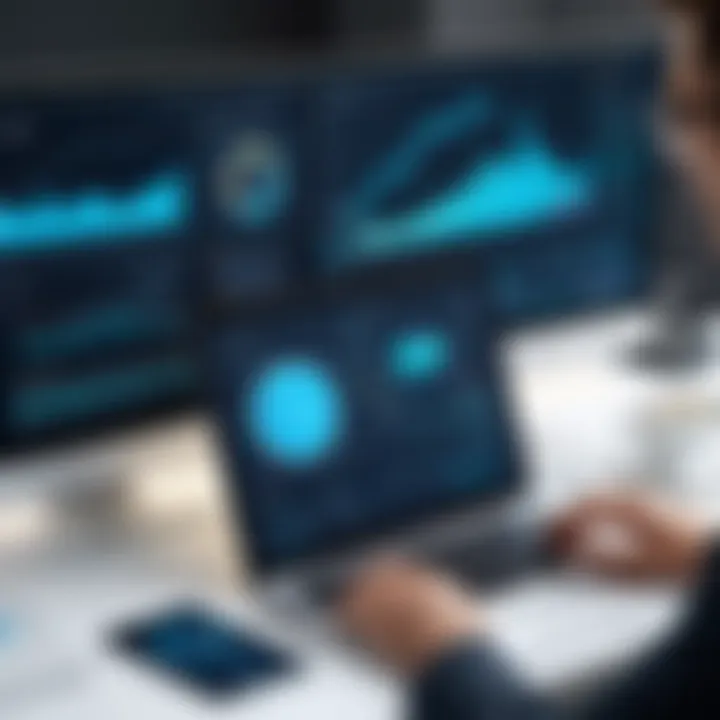
Data does not stay pristine and requires regular cleaning to remain effective. This might entail eliminating duplicate entries, updating outdated information, and establishing rules for periodic reviews.
The unique feature of this stage is its interplay between automation and human oversight. While automation can expedite the cleaning process, human expertise is often necessary to ensure comprehensive assessments. However, relying too heavily on one over the other can diminish overall effectiveness.
Data Usage
Leveraging Data for Decisions
In the end, all efforts in the previous stages aim to serve this critical purpose: leveraging data for informed decision-making. Data-driven decisions can enhance strategies, reveal consumer preferences, and ultimately improve business outcomes.
One of the standout characteristics here is the potential for actionable insights. With well-maintained data, organizations can make strategic pivots or enhancements with confidence. Still, not all data is created equal, and decisions based on weak datasets can lead to misguided directions.
User Roles in Data Interpretation
Different stakeholders within an organization will interpret data in varied ways based on their roles and goals. Whether it’s data analysts, marketing teams, or upper management, each group approaches data interpretation with a unique lens.
This diversity in interpretation can either add depth to insights or lead to conflicting understandings. Engaging users early on in data discussions can help clarify intents and align interpretations more harmoniously.
Data Retention and Disposal
Strategies for Data Retention
Strategies for data retention should not be overlooked as they play a crucial role in compliance and accessibility. Organizations must balance keeping necessary information without becoming burdensome.
One of the most important characteristics of effective retention strategies is determining the lifecycle of data. Knowing when to keep data and when to purge it can help organizations avoid unnecessary complications, both in terms of cost and risk management. However, establishing these strategies can sometimes lead to tough decisions and complexities.
Ethics of Data Disposal
Ethical considerations surrounding data disposal cannot be neglected. This stage involves understanding not just how to dispose of data, but when and in what manner. Following ethical guidelines—such as anonymization or secure deletion—protects sensitive information from misuse and ensures compliance with regulations.
Focusing on the responsibilities that come with data disposal can prevent potential legal ramifications and strengthen trust with stakeholders.
In sum, navigating the stages of the data quality lifecycle provides an organized approach to maintaining data integrity and ensures that organizations can depend on their data for critical decisions.
Challenges in Managing Data Quality
Data quality management is no walk in the park, especially in a world flooded with information. Organizations often stumble into various hurdles that could compromise their data's integrity and reliability. This section navigates through some of the significant challenges faced in managing data quality. By recognizing these obstacles, data professionals can devise targeted strategies to mitigate their impact and foster a robust environment for high-quality data.
Data Integration Issues
Data integration is the backbone of data quality. However, it often presents a maze of challenges. Different systems, platforms, or departments may use varied formats, languages, or structures to store information. This fragmentation not only complicates data aggregation but also raises the specter of inconsistencies.
For instance, an organization might pull sales data from an old legacy system while simultaneously pulling customer feedback from a modern cloud-based solution. Disparities can arise, leading to conflicts in metrics and analyses. Integrating disparate data sources requires a deep understanding of each system's functionality and data structure. Moreover, with APIs frequently evolving, maintaining integration becomes a constant project requiring continuous attention.
To tackle these challenges, a few approaches can be beneficial:
- Establish Standard Protocols: Create consistent data formats and structures across all platforms.
- Invest in Middleware Solutions: Adopt software that facilitates the seamless movement of data between different systems.
- Prioritize Data Governance: A governance framework ensures data accuracy and consistency across the board.
Human Error in Data Handling
When it comes to data management, human error is an ever-present shadow. Even the most skilled professionals can slip up. An employee might accidentally record sales figures incorrectly, or another may fail to update customer details after an interaction. The implications can be far-reaching. Poor data can distort analytics and lead to misguided decisions, ultimately affecting the bottom line.
To combat this, organizations must cultivate a culture of awareness and responsibility around data entry and management. Simple steps can go a long way:
- Regular Training and Workshops: Continuously educate staff on data handling best practices.
- Implement Tight Controls: Use approval systems for critical data entries to catch mistakes before they cause problems.
- Promote Team Collaboration: Encourage cross-departmental reviews to observe data entries and ensure they've been recorded accurately.
Evolving Data Standards
The landscape of data is an ever-evolving one. As technology advances, so too do the standards for data quality. What was considered optimal just a year ago can quickly become outdated. For instance, regulations like GDPR and CCPA have altered how businesses approach data privacy and quality. Adapting to these changing standards is not just a matter of keeping up with the trends - it’s essential for compliance, risk mitigation, and trust-building with stakeholders.
Organizations must not only adjust internally but also keep an eye on the broader industry trends that may affect their operations. Key actions to consider include:
- Stay Informed: Regularly check updates from authoritative bodies to align with new standards.
- Revise Practices Accordingly: Tailor data practices to ensure compliance with evolving regulations.
- Engage in Industry Dialogues: Participate in conferences or forums where changes in standards are discussed—this can foster awareness and best practices.
"Understanding the challenges of data quality management is the first step in creating resilient data practices. Recognizing these barriers allows organizations to turn potential pitfalls into opportunities for improvement."
By actively addressing these challenges, businesses can carve a smoother path to high-quality data, bolstering their decision-making processes and maintaining the trust in the data they rely upon.
Measuring Data Quality
Measuring data quality is like checking the pulse of an organization’s most valuable asset: its data. Without proper measurement, it’s hard to determine if the information being processed and analyzed is up to snuff. This section seeks to shed light on the significance of quantifying data quality levels, illuminating the common pitfalls and best practices for ensuring that the data can really be trusted to inform decisions.
Effective data measurement is not just about picking out random numbers and calling it a day. It’s about establishing a comprehensive framework that incorporates specific indicators, enabling organizations to understand various facets of data health. Not only does this help in identifying areas for improvement, but it also illustrates the direct line of impact that quality data can have on trust and decision-making processes.
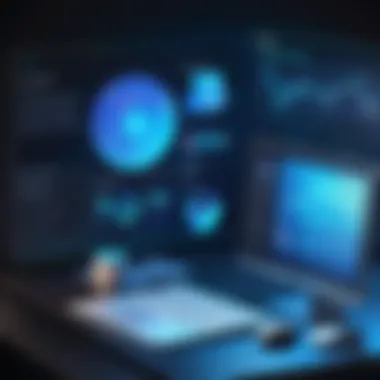
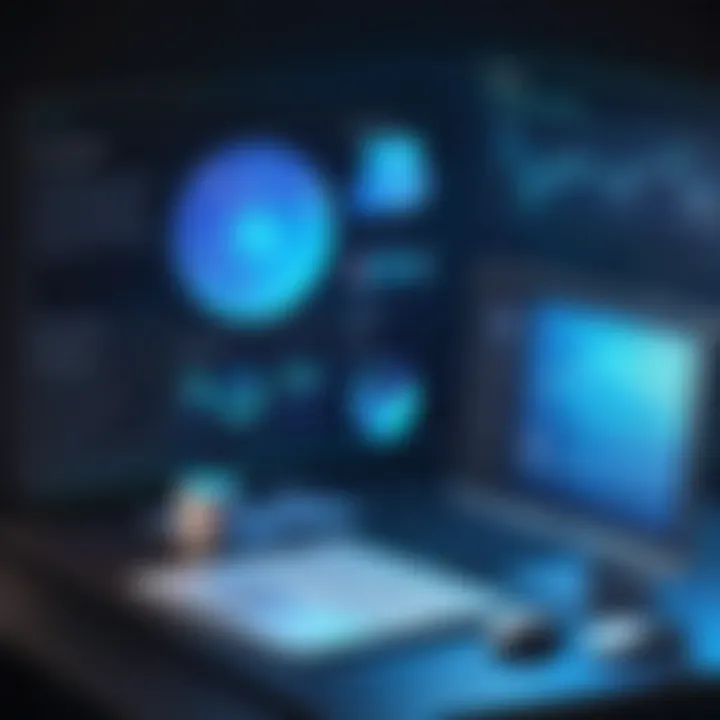
Key Performance Indicators for Data Quality
When it comes to assessing data quality, a few key performance indicators or KPIs stand out. They serve as the benchmarks that inform stakeholders about the present state of their data, guiding any necessary adjustments. Here’s a focus on three critical KPIs:
Accuracy
Accuracy refers to how closely data values align with the true values or the target condition. In any type of analysis, wrong assumptions to incorrect conclusions stem from flawed data accuracy. In terms of contribution, accuracy helps build the foundational trust that analysts require when interpreting data.
The hallmark of accuracy is its precision. For instance, tracking employee performance might hinge on reported sales figures; if these numbers are off, so too are the decisions made based on them. One notable aspect of accuracy is its ability to drive actionable insights, allowing organizations to pivot promptly when faced with inaccuracies.
However, accuracy can be a double-edged sword. High accuracy is an expensive venture, often requiring regular updates and checks, which can overwhelm smaller organizations or those with limited resources. Thus, businesses must strategically weigh the costs against their specific needs and capabilities.
Completeness
Completeness is about ensuring that all necessary data is available for analysis. Think of it this way: if a puzzle is missing a few pieces, the picture is incomplete. The contribution of completeness to an organization is profound; it allows for a holistic view of situations without leaving gaps that could lead to misinterpretation.
A defining feature of completeness is the extent to which all pertinent data is recorded. For example, if a marketing team lacks data from a certain demographic, their strategies may miss the mark entirely. This aspect is often popular as it plays a critical role in ensuring that business analytics are robust and reliable.
The downside? Achieving complete data can be a labor-intensive mission. Gathering further data might involve additional tools, methods, and, almost certainly, costs. Hence, companies need to navigate these trade-offs carefully, weighing quality against the operational impact of pursuing completeness.
Consistency
Consistency refers to the uniformity of data across different datasets, systems, or processes. It’s all about ensuring that data remains reliable over time. When a dataset displays consistent values, stakeholders can trust its findings to drive decisions.
One key trait of consistency is its relational aspect; if a customer's address is recorded differently in various systems, confusion arises, leading to mishaps in service delivery. Thus, consistent data is crucial for operational fluidity. It acts as a bedrock for integrated systems, where data entered at one point feeds into another without discrepancies.
Yet, achieving consistency can be daunting, especially in large organizations with multiple systems. It requires ongoing vigilance and perhaps tools that monitor and enforce consistency across platforms. While it’s core to maintaining quality, organizations must invest in practices that support this continuous oversight.
"Staying consistent is key to trust – without it, your data is like a ship without a rudder."
Tools for Assessing Data Quality
To effectively measure data quality, a suite of tools is often required. These tools can analyze, design, and improve your data, helping businesses to maintain the necessary quality standards. Consider leveraging software solutions such as Talend, Informatica, and Trifacta for robust data quality assessments.
Moreover, methodologies such as Six Sigma and Total Quality Management can provide frameworks that align data measurement with broader quality initiatives in an organization. This aligns the data assessment process with strategic goals, ensuring that data quality isn’t just an isolated endeavor but part of a larger quality-centric ethos.
By prioritizing these tools and methodologies, organizations can create a systematic approach to data quality that aligns with their operational goals and industry standards.
Strategies for Enhancing Data Quality
Enhancing data quality is like tuning a finely crafted instrument; it requires continuous attention and a structured approach. In today’s rapidly evolving data landscape, businesses must undertake strategies aimed at enhancing data quality to ensure effective decision-making and operational efficiency. This section focuses on two critical components that can make a significant impact: data governance frameworks and training and awareness programs.
Data Governance Frameworks
A robust data governance framework lays the foundation for effective data quality management. It's crucial to establish a clear structure that dictates how data is managed, where accountability lies, and how quality is maintained throughout its lifecycle.
Some essential elements include:
- Policies and Standards: These should establish guidelines for data entry, storage, processing, and sharing. Specify clear definitions of data quality metrics and set high standards.
- Roles and Responsibilities: Defining who is accountable for data quality can help eliminate overlaps and gaps. Assign roles so that data stewards, quality analysts, and other stakeholders know their part in maintaining high standards.
- Audit and Compliance Mechanisms: By instituting regular audits, you can systematically identify weaknesses. This way, organizations can prevent data quality degradation before it becomes a major issue.
- Stakeholder Engagement: Involve various departments across the organization in governance discussions. Engage IT, compliance, marketing, and analytics teams to develop a shared understanding of what quality data means.
By weaving these elements into the fabric of the organization, companies can build a sustainable approach to data quality that evolves alongside changing business needs. In doing so, they not only improve the credibility of their data but also foster a culture of accountability.
"A solid data framework does not just safeguard quality, it also cultivates a culture of respect for data among employees."
Training and Awareness Programs
Even the best frameworks can fall flat without a workforce that grasps the principles of data quality. Training and awareness programs play a pivotal role in making every employee an effective steward of data. These programs should prioritize:
- Foundational Knowledge: Establish courses that cover basic data concepts, types of data, and importance of quality. Take time to explain why data integrity matters on both an organizational and individual level.
- Hands-On Training: Real-life scenarios and case studies can be invaluable. Encourage employees to engage in exercises that require them to test their decision-making skills based on the data they handle.
- Feedback Mechanisms: Create channels for employees to report issues or provide feedback on existing processes. This can result in useful insights on how to better manage data quality from those who work directly with it.
- Ongoing Learning: Data quality isn’t a one-and-done affair. Continual education should be part of the organization’s culture. Online courses, workshops, and certifications can help keep skills sharp.
Incorporating these training initiatives helps foster an environment where data quality is everyone’s responsibility. It cultivates a community that actively seeks to understand and improve the data they interact with, leading to noticeable enhancements in data quality across all levels.
Together, data governance frameworks and training programs create a synergistic effect that uplifts data quality practices. As data continues to be an integral asset for organizations, implementing these strategies will fortify their data landscape, paving the way for sound decision-making and trust in data-driven insights.
Closure and Future Perspectives
The data quality lifecycle stands as a pillar for organizations navigating the vast ocean of information in today’s digital age. It emphasizes how crucial each stage is, from the initial creation of data to its eventual retirement. As companies continue to become data-centric, the imperative to maintain data quality becomes all the more pressing. Poor data translates to misguided decisions, which can lead to significant financial losses and tarnished reputations. Therefore, understanding the dynamics of this lifecycle ensures that data is not only accurate but also reliable and actionable.
The Evolving Nature of Data Quality
The landscape of data quality is ever-shifting. With advancements in technology and the explosion of data types, organizations must adapt their strategies continually. Old methods may not suffice against the backdrop of real-time data processing and machine learning algorithms. The introduction of the cloud has reshaped how data is managed, necessitating a reexamination of traditional approaches to ensure quality remains front and center. Moreover, regulations like GDPR further complicate data management, demanding robust frameworks that promote not just compliance but also ethical handling of data.
In this context, organizations must foster a culture of continuous improvement, where regular assessments of their data practices become standard.
Final Thoughts
Navigating this complex landscape might seem daunting, but the rewards—better decisions, enhanced productivity, and a competitive edge—are well worth the effort. Going forward, embracing a mindset of adaptability and vigilance will be key to mastering data quality in an uncertain future.
"To succeed in the modern world, data quality mustn't just be a goal; it should be a guiding principle."
Embracing this perspective will not only fortify decision-making processes but also foster an environment where data is held as a strategic asset. Ultimately, the future favors those organizations that are willing to invest in the continuous pursuit of data excellence.