AWS SageMaker Pricing Explained: Your Comprehensive Guide
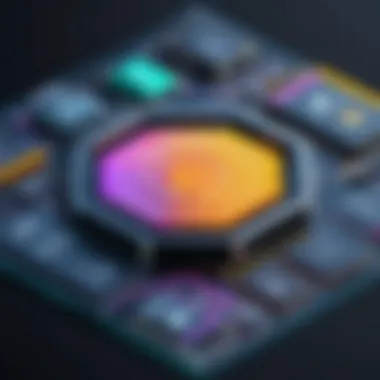
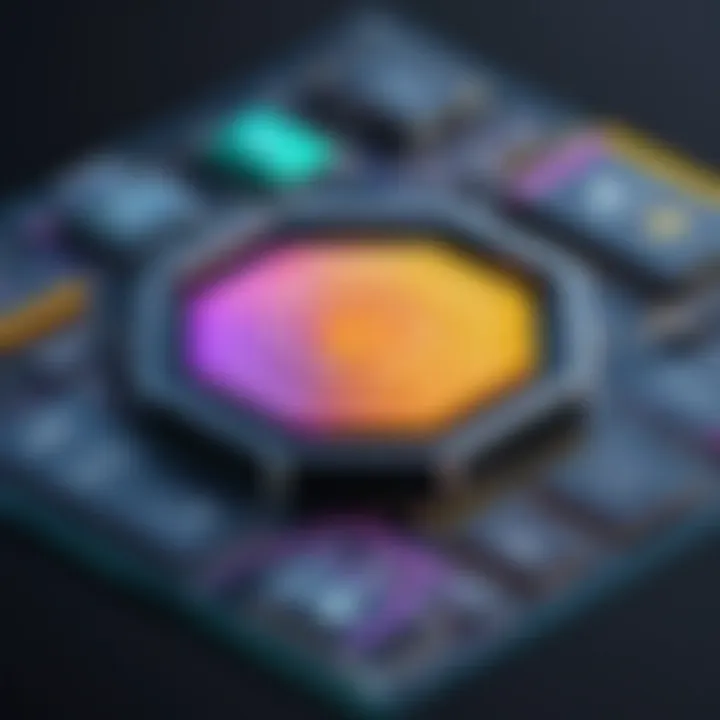
Intro
Understanding the pricing structure of AWS SageMaker is crucial for those looking to implement machine learning solutions effectively. The platform stands out with its extensive features that cater to various users, from small enterprises to large organizations. Grasping the pricing model can pave the way for efficient budget management and project planning, ultimately impacting a company’s return on investment in AI technologies.
AWS SageMaker provides an environment that empowers developers to build, train, and deploy machine learning models at scale. The costs associated with using this service can be influenced by a myriad of factors, including instance types, data storage, and additional services that one might opt for. A detailed look at these components not only demystifies the overall expenditure involved but also helps users make strategic decisions tailored to their project needs. By the end of this guide, readers will appreciate the intricacies of SageMaker's pricing, enabling them to tailor their usage efficiently.
Software Overview
Features and functionalities
AWS SageMaker is much more than just a machine learning platform; it encompasses a whole suite of services designed to facilitate every stage of a machine's life cycle. Key features include:
- Built-in algorithms: From linear regression to deep learning, SageMaker provides various pre-built algorithms to kickstart your projects.
- Model training: With the option to choose from different instance types, users can scale model training according to their needs.
- Notebook Instances: These provide a simpler way to interact with data and work on models in an intuitive interface.
Additionally, SageMaker integrates seamlessly with other AWS services like S3 for storage and CloudWatch for monitoring, creating a comprehensive ecosystem for AI solutions.
Pricing and licensing options
The pricing model of AWS SageMaker can be broken down into components like:
- Instance Pricing: Based on the type of instance being used, whether for training or inference. Different instances come with varying costs.
- Data Storage: Costs for data stored in S3 and data transfer fees.
- Training Jobs: Charged based on the time taken and resources utilized during training sessions.
AWS SageMaker follows a pay-as-you-go model, ensuring users only pay for the resources they actually use. This makes it notably flexible, accommodating a range of budgets and project scopes.
Supported platforms and compatibility
SageMaker operates within the AWS ecosystem, therefore, it is fully compatible with multiple AWS services. Its interoperability extends to popular data formats and frameworks, including TensorFlow, PyTorch, and MXNet. This means it's quite adaptable to the existing workflows of many businesses looking to leverage machine learning.
User Experience
Ease of use and interface design
The platform is designed with user-friendliness in mind. Navigating AWS SageMaker is relatively straightforward, even for those who may not be familiar with all aspects of machine learning. The interface promotes accessibility, with various shortcuts and features laid out logically. The overall experience encourages experimentation without feeling cumbersome.
Customizability and user settings
AWS SageMaker allows users to tailor rules and settings to match their specific project needs. For example, you can customize instance types when deploying models or adjust training parameters to enhance performance.
Performance and speed
Performance is where AWS SageMaker shines. Users have reported fast model training times, particularly when using appropriate instances. For high-end processes, SageMaker offers options such as distributed training, which can significantly cut down the waiting time involved in training large models.
Pros and Cons
Strengths and advantages of the software
- Comprehensive tools: Provides an all-in-one solution for the machine learning life cycle.
- Scalability: Users can easily scale their resources up or down depending on project demands.
- Integration: Works smoothly with other AWS services.
Drawbacks and limitations
- Complex pricing: Users often find the pricing for different services can be overwhelming at first.
- Learning curve: While user-friendly, there is still a learning curve associated with fully leveraging the platform's capabilities.
Comparison with similar products
Compared to similar platforms like Google Cloud AI and Microsoft Azure ML, AWS SageMaker clearly presents its own unique offerings, particularly in how well it integrates within the AWS ecosystem and its pay-as-you-go pricing model, which can be appealing to many users.
Real-world Applications
Industry-specific uses
Various industries, from healthcare to finance, have found utility in AWS SageMaker. It has proven effective in predictive modeling, data analysis, and automating workflows.
Case studies and success stories
Many companies have successfully implemented AWS SageMaker for their machine learning projects. For example, Netflix uses the platform for recommendation systems, improving user experiences through tailored content suggestions.
How the software solves specific problems
One of the significant advantages of using AWS SageMaker is its ability to handle large data sets efficiently. This capacity allows businesses to draw insights and derive value from their data more effectively.
Updates and Support
Frequency of software updates
AWS regularly updates SageMaker, introducing new features and improvements based on user feedback. Keeping abreast of these changes is essential for maximizing the tool's potential.
Customer support options
AWS offers support through various channels, including documentation, forums, and customer service representatives. Users can typically find answers to common queries without much hassle, which is ideal for both novices and seasoned professionals.
Community forums and user resources
Ethical discussions and shared experiences can be found throughout the AWS community pages. Many users take to platforms like Reddit to share their findings and solutions, creating a collaborative environment that benefits everyone involved.
Foreword to AWS SageMaker Pricing
In the realm of machine learning, AWS SageMaker stands out as a powerful platform, offering numerous tools and capabilities for building, training, and deploying models. However, understanding the pricing model of AWS SageMaker is essential for anyone planning to leverage its features. Pricing can be a maze with its various components, and getting a grip on it can save both time and money. This section aims to illuminate the key aspects that contribute to AWS SageMaker's pricing, making it clearer for IT professionals, businesses, and software developers alike.
What is AWS SageMaker?
AWS SageMaker is a fully managed service that allows developers and data scientists to build, train, and deploy machine learning models quickly. It brings together a variety of services and tools designed to simplify the machine learning workflow. These include data labeling, model training, and model tuning, among others. Think of it as a one-stop shop for all your machine learning needs, giving users the ability to focus more on innovation rather than the complex underlying infrastructure needed for machine learning tasks.
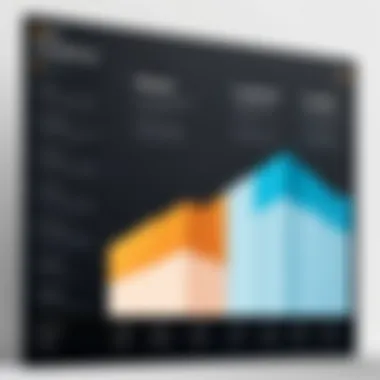
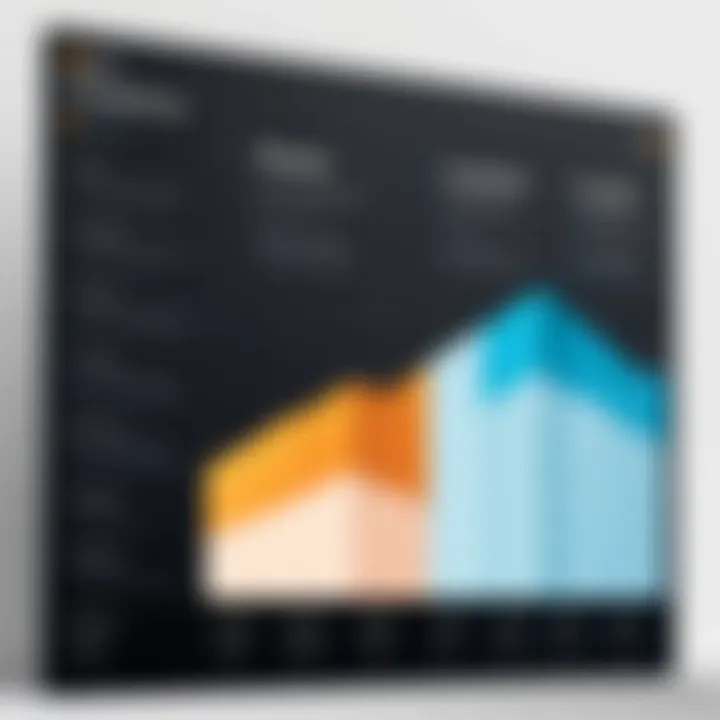
Importance of Understanding Pricing
Grasping the nuances of AWS SageMaker pricing is not just a nice-to-have; it’s imperative.
- Budgeting Effectively: Knowing how much different services cost helps in planning the budget more efficiently. If you inadvertently run high-end instances without a good grasp on costs, you might find your expenses skyrocketing.
- Optimizing Resources: Understanding the pricing structure allows companies to match their resource usage to their actual needs, avoiding over-provisioning.
- Cost-Benefit Analysis: As businesses weigh which tools and services to use, knowing the pricing can help them ascertain which offerings provide the best value for money.
"In machine learning projects, every dollar counts. An in-depth knowledge of pricing can be the difference between innovation and budget overruns."
In short, this part of the article sets the stage for a thorough examination of AWS SageMaker's pricing, laying down a foundation for informed choices and strategic decision-making.
Overview of Pricing Structure
Understanding the pricing structure of AWS SageMaker is crucial for businesses aiming to optimize their machine learning expenditure. The pricing can be multifaceted, often concealed within layers of components that can bewilder even seasoned professionals. A well-rounded grasp of this structure not only ensures that your organization remains within budget but also aids in strategic planning for future projects.
Parts of the pricing structure range from fundamental costs tied to the instances you select to more nuanced fees associated with data processing, training, and deployment. Each element plays a role in the overall cost and can vary widely based on factors like usage patterns and configuration choices.
Cost Components
When discussing cost components, it's paramount to dissect the core areas that contribute to your AWS SageMaker bill. There are several key components to keep in mind:
- Instance Pricing: This is often the most significant part of your costs. AWS offers various instance types, each with its pricing model. Understanding the differences is essential.
- Data Processing: Charges accrue with the volume and type of data processed. This could include any data transformations or movements.
- Storage Fees: Costs associated with data storage in S3 or EBS can add up. Pay attention to the amount of storage utilized, along with any retrieval fees.
- Networking Costs: Depending on your deployment configuration, transferring data in and out of AWS can incur additional charges.
These components are more than just dry numbers; they affect your budgeting decisions.
Service-Level Variations
Service-level variations can impact how much you'll end up paying. Different service levels exist, which can confuse new users. Here are some noteworthy points:
- Regional Price Differences: AWS operates in different regions, and prices can vary by location. It’s worth looking into whether a different region might offer cost savings for your project.
- Tiered Pricing Models: Some services may offer tiered pricing, where costs decrease as usage increases, meaning bulk or sustained usage may result in savings.
- Free Tier Options: For businesses to experiment with SageMaker, understanding what's included in the free tier offers valuable opportunities to test things without hefty commitments.
It's vital to map out and evaluate these variations, which can often lead to unexpected costs as your usage scales. To sum up, getting a handle on the complexity of AWS SageMaker pricing provides essential insights that can bolster your machine learning initiatives and optimize resource utilization.
Instance Pricing Explained
Understanding the pricing of instances within AWS SageMaker is crucial for anyone venturing into machine learning projects. Instances are the building blocks of any workload in SageMaker and represent the servers on which models are trained, evaluated, or deployed. By grasping the intricacies of how instance pricing works, organizations can better control their spending and optimize their resource allocation.
An informed approach allows businesses of all sizes—from small startups to large enterprises—to make data-driven decisions about how they utilize AWS SageMaker. The impact of choosing the right type of instance extends not just to cost but also the performance and speed of machine learning workflows. A small difference in instance size or type can translate into significant cost variations, especially when running long training sessions or scaling up deployments.
Types of Instances
AWS SageMaker offers a variety of instance types, tailored to fit diverse needs. Here are some notable categories:
- General Purpose Instances: These are versatile and suitable for a wide range of workloads, combining compute, memory, and networking resources. Think of them as the all-rounders of the instance world.
- Compute Optimized Instances: Designed for compute-heavy tasks, these instances deliver high performance for machine learning training and inference. If your models are computational beasts, this is the route to consider.
- Memory Optimized Instances: Ideal for processing large datasets in memory, these instances are a must for data-intensive training jobs. When your models need to juggle large amounts of data, these instances are up to the task.
- Accelerated Computing Instances: This category includes options equipped with GPUs or other hardware accelerators. For tasks such as deep learning and complex simulations, these are indispensable, offering significant performance boosts.
"Choosing the right instance type can be the difference between finishing a job in minutes or waiting hours."
Selecting an instance based on workload requirements leads to efficiency, so it's wise to consult the available documentation and conduct testing to find the best fit.
Spot vs. On-Demand Instances
One of the key decisions in managing instance prices involves understanding the difference between Spot and On-Demand instances.
On-Demand Instances are billed by the hour, allowing you the flexibility to spin up resources as needed without long-term commitments. This is especially advantageous for businesses with variable workloads where predicting usage is challenging. You only pay for what you use—plain and simple.
On the flip side, Spot Instances represent a cost-saving alternative. These are unused AWS capacity available at discounted prices. However, there’s a catch: AWS can reclaim Spot Instances when it needs the capacity back, which can lead to unexpected interruptions during key processes. Thus, while the savings can be substantial, this comes with the risk of having to manage disruptions.
To summarize the comparison:
- On-Demand Instances are reliable and straightforward, ideal for consistent workloads.
- Spot Instances can offer significant savings for flexible tasks but require more robust management strategies to handle potential interruptions.
Evaluating workloads and cost constraints will guide decisions on which instance type works best for each project within AWS SageMaker. It's not just about the bottom line; it's also about ensuring the quality and reliability of machine learning outcomes.
Data Processing Costs
Data processing costs are a critical element when considering the usage of AWS SageMaker. Understanding these costs is paramount for businesses aiming to leverage machine learning capabilities efficiently. When you think about the expenses linked to data processing, it goes beyond just the initial setup. It encompasses data ingestion, storage requirements, and any necessary transfers. Each component can pile up quickly, impacting your overall budget and project profitability.
For those in IT or software development, knowing how to navigate these costs means making informed decisions about project scope and resource allocation. A clear awareness could lead to significant savings in the long run, especially for large businesses with expansive datasets.
Understanding Data Inputs
When using AWS SageMaker, your journey starts with data inputs. The type and volume of data you feed into the system can dramatically influence processing costs. For instance, if you're working with big datasets from sources like IoT devices or social media, the expenses can shoot up due to the increased complexity and volume.
Consider these key points when analyzing data inputs:
- Data Variety: Different formats like CSV, JSON, or images may have unique handling costs. Each type requires different approaches for storage and retrieval.
- Data Volume: Larger data sets increase the need for processing power and storage, leading to higher costs. It’s essential to understand how much data you genuinely require for your project.
- Data Quality: Ensuring that your data is clean and well-organized can save costs related to debugging and processing later on.
Adopting a strategy for managing your data inputs can help in controlling expenses. For instance, preprocessing data to filter out unnecessary information can significantly reduce the load on AWS SageMaker, leading to more manageable costs.
Data Storage and Transfer Fees
Once data is in AWS, how you store and transfer it also has financial implications. AWS typically charges for both data storage and transfer, which adds up over time. The following are key aspects to consider:
- Storage Types: Choosing the right storage option is crucial. AWS offers various solutions like S3 for object storage and EBS for block-level storage. Each comes with its own pricing model, based on factors like durability, retrieval time, and access frequency.
- Data Transfer Patterns: When you move data in and out of AWS, costs arise not just from the transfer, but also from bandwidth usage. Regularly transferring large sets of data, for instance, can rack up considerable fees.
- Cost Optimization Strategies:
- Utilize S3 lifecycle policies to automatically transition data to less expensive storage classes as it ages.
- Implement data compression methods to minimize the volume of data transferred.
- Consider AWS Free Tier options if you are just starting out to get a feel for the costs without breaking the bank.
"Efficient data management can mean the difference between a successful project and a costly misadventure."
Understanding the nuances of data processing costs can empower decision-makers in both small and large businesses. It equips them with the knowledge needed to refine their machine learning approaches on AWS SageMaker, fostering long-term strategic advantages.
Training Costs
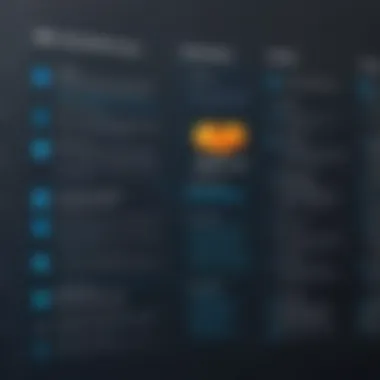
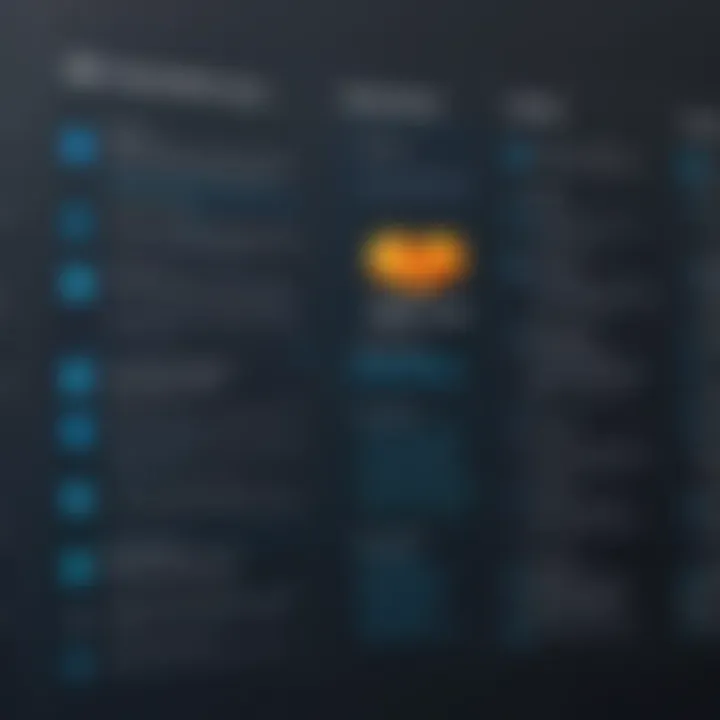
Training costs can often feel like a black box when diving into machine learning projects. Understanding how these costs are structured in AWS SageMaker is crucial for IT professionals and businesses aiming for efficiency and accountability in their budget allocations. It’s not just about the dollars and cents spent; it’s about the strategic decisions that can lead to significant cost savings over time.
The essence of training costs in SageMaker ties back to several key aspects. First, training a model requires computational resources, which can pile up quickly. Additionally, the length of training sessions can weigh heavily on total costs. Factors influencing these costs must be considered at every stage, from the initial setup to the final deployment.
Also, let's keep in mind that the approach to data preparation can affect the overall outlay as well. Getting your data ready for training isn't just a box to check; it can save you a bundle if done right.
Factors Influencing Training Fees
When assessing training fees, it’s vital to consider a myriad of factors. These can influence how much one ends up spending in the long run:
- Instance Type: Different instance types come with different costs. Choosing between CPU and GPU instances can drastically change the fees. For instance, GPU instances often cost more but can process data much faster, leading to potential savings on time.
- Volume of Data: The amount of data being processed also plays a significant role. Larger datasets often mean more expensive training, so businesses must weigh the benefits of expanding their data against potential cost increases.
- Algorithm Complexity: Some algorithms require more resources than others. A complex neural network may need a lot of horsepower, which drives up costs.
- Hyperparameter Tuning: When details matter, and tweaking is needed, tuning parameters can lead to increased training times and costs, but also better model performance.
These factors mold the overall cost landscape, making it essential to understand what's at play.
Duration and Computational Limits
Duration directly dovetails with computational limits in AI training jobs. The longer the training runs, the higher the expense, but there’s more to it than that. AWS SageMaker embeds specific computational limits within its offerings that can result in different cost scenarios.
For example, if one runs a long training job on a powerful instance total, the dollar signs grow quickly. Conversely, running training in bursts on smaller, cheaper instances may lead to lesser immediate costs but could actually elongate the overall project timeline.
Moreover, utilizing features such as distributed training can help but requires careful consideration. It can speed up training by splitting the workload, but if not managed well, it can also inflate costs.
"Understanding the balance between duration and computation not only helps in budgeting but also in optimizing for the best performance of your machine learning models."
In summary, training costs in AWS SageMaker aren’t solely about the number on an invoice; they encapsulate the very strategy of how your project will succeed and grow. Careful analysis of influencing factors and a keen eye on duration help pave the way towards a well-rounded financial strategy in utilizing AWS SageMaker effectively.
Deployment Costs
Understanding deployment costs is crucial when integrating AWS SageMaker into your machine learning workflows. These costs can significantly impact your budget and decision-making, especially for businesses that rely heavily on machine learning to achieve their goals. Proper management of deployment expenses allows organizations to optimize resources and ensure cost efficiency as they scale.
Endpoint Configuration Charges
Endpoint configuration is one of the primary contributors to deployment costs in AWS SageMaker. When deploying a model, you need to set up an endpoint, which acts as the interface where the model can receive requests for inference. The charges related to this configuration can vary based on several factors.
- Instance Type: The choice of instance type directly influences costs. For instance, AWS offers instances with varying computational power and memory capacity. Opting for a more powerful instance can yield faster inferencing but will also incur higher charges.
- Endpoint Count: Each deployed model requires a separate endpoint. If you have multiple models feeding into different endpoints, the costs can add up quickly.
- Data Input Size: The volume of data being processed by the endpoint also plays a role in pricing. Larger datasets may necessitate more powerful configurations, impacting your overall budget.
While setting up endpoints might seem straightforward, it is wise to analyze the trade-offs between performance and cost carefully. Businesses should consider their specific needs when determining how to configure endpoints, as a well-planned approach can save money in the long run.
Scaling Considerations
Scaling your deployment effectively can be a double-edged sword. On one hand, adequate scaling ensures that your applications run smoothly during different loads of requests. On the other, poorly managed scaling can lead to unnecessary expenditures.
- Auto Scaling: AWS SageMaker provides tools for automatic scaling based on predefined metrics. This means you can adjust the number of instances serving your endpoint dynamically. However, if you set the threshold too low, you may face performance lags; too high, and you're just pouring money down the drain. Finding the sweet spot is essential.
- Reserved Instances vs. On-Demand: For long-term use, reserving instances can lower your deployment costs compared to relying purely on on-demand pricing. Analyze your usage patterns carefully – if your workload is steady and predictable, the savings from reserving instances can be significant.
- Monitoring Usage: Implementing observability tools to monitor the scaling actions of your endpoints can help you fine-tune your scaling strategy. Use services like AWS CloudWatch to gain insights into utilization and performance.
Effective scaling takes careful planning and a nuanced understanding of usage patterns. By keeping a close watch on deployment metrics and costs, organizations can manage their AWS SageMaker resources better, ensuring both efficiency and cost-effectiveness.
Investing time in understanding deployment costs can lead to substantial long-term savings and improve overall performance of your machine learning applications.
Additional Features and Services
In the realm of AWS SageMaker, the additional features and services are not just supplementary functions; they can be pivotal in shaping the overall cost landscape and enhancing the functionality of machine learning projects. Understanding these elements allows users to make educated choices, ensuring that their investments align with their operational needs and strategic goals. Each feature brings its own pricing considerations, complexities, and advantages, which can significantly affect long-term budgeting and expenditure.
Built-in Algorithms Pricing
AWS SageMaker comes equipped with a range of built-in algorithms that are optimized for various machine learning tasks. These include options tailored for linear regression, deep learning, and even more specialized algorithms. The pricing structure for these algorithms varies, typically based on the underlying infrastructure they require. When incorporating built-in algorithms, businesses can save time and resources as they do not need to build algorithms from the ground up.
- Cost per use: Utilizing built-in algorithms usually entails a variable charge that aligns with the compute resources consumed during training or inference. It's prudent to analyze the computational needs before diving in.
- Performance efficiency: Not only do these algorithms reduce setup time, but they also optimize performance, which can lead to financial savings. An efficient algorithm uses fewer resources, ultimately lowering the costs incurred.
For instance, when using the XGBoost algorithm provided by AWS, the model can scale up efficiently, leading to a reduction in both processing time and associated costs for training sessions. With such options readily available, users must weigh the cost against the anticipated outcomes to secure the best value for their needs.
Model Monitoring Fees
Monitoring machine learning models post-deployment is another essential feature offered by AWS SageMaker. This process ensures that models are functioning optimally and can adapt to any changes in data patterns, which might affect predictions. However, it's crucial to recognize that model monitoring incurs fees that can become substantial depending on the project's scale.
- Real-time insights: The service provides real-time metrics and logs, enabling users to detect any anomalies or drift in model performance. Getting these insights can be invaluable for maintaining the accuracy and relevancy of predictions.
- Budgeting considerations: Users should consider how often they need to access these monitoring services. Frequent monitoring could lead to increased costs.
- Long-term benefits: Investing in robust monitoring measures can prevent larger losses down the line by allowing for swift corrections. In industry settings, where even minor inaccuracies can lead to significant financial repercussions, this feature becomes even more crucial.
"The ability to monitor and adapt your machine learning models efficiently can be the difference between success and failure in a rapidly evolving market."
In summary, by fully understanding the additional features and services of AWS SageMaker, businesses can skillfully navigate their pricing options. This awareness not only aids in cost management but also empowers users to leverage SageMaker's complete set of tools to enhance their applications and maximize the return on investment.
Cost Management Strategies
Navigating the world of AWS SageMaker comes with its own set of challenges, particularly when it comes to managing costs. Effective cost management strategies are not just about minimizing expenses, but more about maximizing the value you derive from your investments. Understanding the various pricing components helps clarify where you can optimize.
Estimation Tools and Calculators
AWS provides several estimation tools and calculators that can help demystify the complexity of pricing. These tools allow users to input specific project parameters — such as anticipated data processing quantity or instance type — and get a rough idea of what their monthly bill might look like.
For example, when planning, you can utilize the AWS Pricing Calculator. This tool can estimate costs based on your configurations and intended usage. It’s essential to consider factors like potential increases in data volume or sudden spikes in demand.
- Input your desired instance type and other resource components
- Evaluate different service configurations
- Compare costs across multiple regions if applicable
Using estimates from these calculators can give you a more accurate picture, which assists in both budgeting and resource allocation. Moreover, they serve as a sanity check against your spending against your actual usage.
Budgeting for Machine Learning Projects
Budgeting for machine learning initiatives within AWS SageMaker is crucial for steering clear of unexpected costs. It's not all about sticking to a predefined budget but also about ensuring appropriate funds are allocated for necessary services. Consideration for the project's scale, complexity, and duration all play vital roles.
Here’s a simple approach to budgeting:
- Define your scope
Consider what you want to achieve with your project before diving into resource commitments. Define metrics for success, and this will help resist scope creep during implementation. - Itemize costs by component
Break down the costs into resources you’ll use — such as data storage, training hours, and deployment expenses. Knowledge of these figures helps you avoid nasty surprises later. - Include buffer amounts
Give yourself room for the unexpected. Setting aside a percentage of your budget for miscellaneous expenses can act as a safety net, particularly in stages where adjustments are commonplace. - Review periodically
Set milestones to review your spending against the planned budget regularly. This practice allows you to fine-tune ongoing projects and make informed adjustments as necessary.
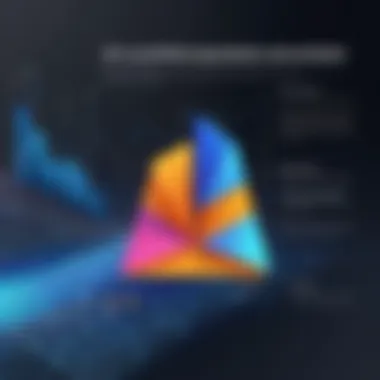
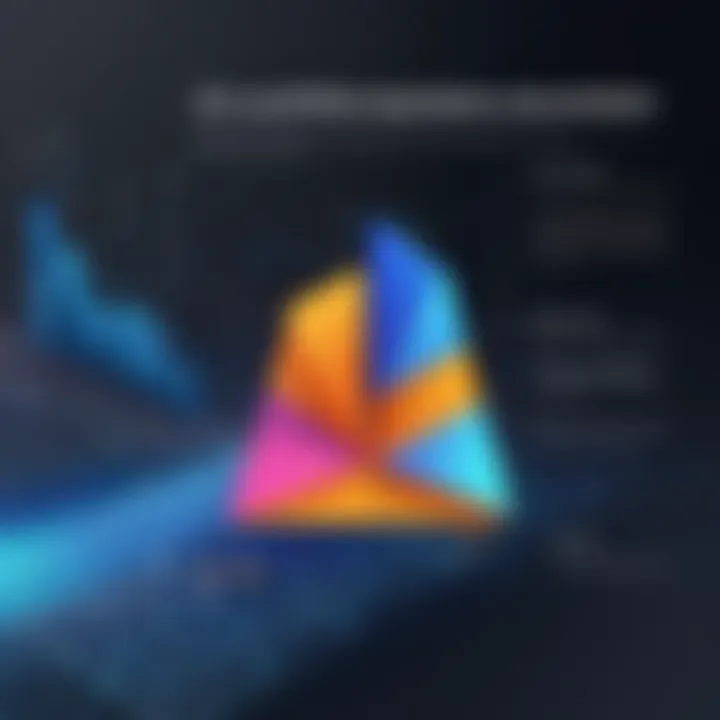
Effective budgeting not only safeguards your financial resources but also leads to strategic decision-making and prioritizing project components.
Keeping an eye on costs and being proactive about budgeting can streamline machine learning efforts within AWS SageMaker. This vigilance leads to informed resource utilization and uncovers opportunities to trim the fat out of unnecessary expenditures.
Comparative Analysis with Competitors
In the rapidly evolving landscape of machine learning platforms, the ability to compare AWS SageMaker's pricing with that of its competitors becomes paramount. Understanding the competitive pricing dynamics allows businesses to judiciously allocate their budgets and resources. A thorough analysis not only illuminates the unique cost structure of AWS SageMaker but also highlights potential areas for savings and efficiency.
The significance of this comparative analysis stems from the necessity for IT professionals and decision-makers to ensure they’re not just getting value for their money, but actually understanding where that value lies relative to alternatives. With many options available—each with its own advantages and drawbacks—this section aims to provide clarity amid the noise.
Pricing Models of Alternative Platforms
While AWS SageMaker employs a specific pricing structure focusing on pay-as-you-go options, alternative platforms like Google AI Platform and Azure Machine Learning offer their own unique models. For instance, Google utilizes a more nuanced approach that sometimes offers preemptible VM instances for reduced pricing, which can be beneficial for cost-sensitive projects. Meanwhile, Azure adopts a hybrid model, combining on-demand and pre-purchased pricing
- Google AI Platform: Offers competitive pricing with flexibility, especially favorable for those needing transient compute power.
- Azure Machine Learning: Integrates machine learning into its broader Azure services, often appealing to enterprises already committed to the Microsoft ecosystem.
- IBM Watson: Follows a subscription-based model which can be advantageous for users looking for predictable expenses.
Working professionals must weigh the trade-offs between these platforms; while initial cost comparison might suggest AWS is pricier, the quality of service and the ecosystem integration are key factors to consider.
Strengths and Weaknesses
Every pricing model comes with its own set of strengths and weaknesses. AWS SageMaker, with its extensive toolset, can be seen as both an advantage and a hindrance depending on use cases.
Strengths:
- Scalability: AWS SageMaker stands out with its capability to seamlessly scale across different machine types, which can optimize costs based on project needs.
- Integration: Staying within the AWS ecosystem can significantly reduce the friction of data transfer and related costs.
Weaknesses:
- Complex Pricing: The diverse pricing components can lead to confusion for newcomers who might miscalculate budgets based on unfamiliar terminologies.
- Higher Base Costs: Compared to some competitors, AWS can have a slightly steeper entry cost, which might deter businesses with tight budgets.
To put things in perspective, while SageMaker has its costs, developers have to think about the overall value it brings to the table. In many cases, the features that support rapid development might outweigh the initial sticker shock for organizations focused on long-term growth.
"What's essential is not merely the cost component, but the holistic value proposition of the platform relative to your project's specific requirements."
This holistic understanding is vital for making informed decisions. As different businesses prioritize different aspects—be it cost, scalability, or support—it's critical to adopt a tailored approach when evaluating these machine learning platforms. Each player brings its own strengths to the game, making it necessary to carefully examine the competitive landscape.
Case Studies
In the realm of cloud computing and machine learning, case studies serve as a vital bridge linking theory to practice. They provide real-world examples that illustrate how organizations harness AWS SageMaker to achieve their goals. This section delves into the specifics of case studies, shedding light on the practical applications of AWS SageMaker and the cost implications that arise from various scenarios.
Real-World Applications of AWS SageMaker
AWS SageMaker isn't just a theoretical platform; it’s effectively applied across multiple industries. Companies deal with data in diverse ways, and the flexibility of SageMaker enables them to tailor solutions that best suit their needs.
- Healthcare: A prime example can be found in how hospitals analyze patient records. By employng SageMaker’s built-in algorithms, healthcare professionals can predict patient outcomes based on historical data, ultimately leading to better care.
- Finance: In finance, firms use machine learning models to detect fraud. SageMaker allows for real-time analysis. It's not just about running numbers; it’s about making quick, informed decisions where every second counts.
- Retail: Retailers leverage SageMaker to personalize shopping experiences. By predicting what products a customer may like based on their past behavior, businesses boost sales while providing tailored content.
These applications showcase the versatility of AWS SageMaker, demonstrating its significant role across sectors while also hinting at the pricing factors involved. The costs associated with instance usage, data transfer, and training can accumulate, yet the benefits often far outweigh these expenses.
Cost Implications in Various Scenarios
Understanding cost implications is a crucial aspect when employing AWS SageMaker. Different scenarios yield different expenses depending on the application and scale. Here are a few considerations:
- Training Scale: For smaller projects, on-demand instances might seem sufficient, but as projects grow, costs quickly add up. Scaling training jobs can inflate expenses unless using spot instances strategically.
- Data Intensive Workloads: Operations requiring a high volume of data can lead to significant storage and transfer fees. Companies must recognize that pushing large datasets can trigger hidden costs not evident at first.
- Long-Term Projects: Projects that extend over several months or years should be planned carefully. As these projects usually leverage numerous services, a comprehensive cost analysis is pivotal to avoid surprise bills.
"Knowing how to manage AWS SageMaker expenses can be the difference between success and bankruptcy for startups."
By evaluating these real-world implementations, businesses can better understand how to approach their pricing concerns. The goal is not just about minimizing costs—it's about leveraging AWS SageMaker for a maximal return on investment. Through these targeted insights, organizations can align their budgeting strategies with the expected benefits, ultimately fostering a more informed and strategic deployment of machine learning technologies.
More examples can be found on related forums, such as Reddit, where users often share their experiences and tips on cost management. Additionally, resources like Wikipedia provide foundational knowledge that can help contextualize these findings.
Future Predictions
Understanding the future of AWS SageMaker pricing is essential for IT professionals and businesses alike. Pricing models, as they currently stand, are constantly evolving. This is driven by numerous factors, such as technological advancements, shifts in market dynamics, and competitive pressures. Being well-versed in these changes can provide a crucial advantage in cost management and project planning.
Forecasting future pricing models allows organizations to align their budgets more effectively and make informed decisions regarding resource allocation. With the ever-growing demand for machine learning solutions, it is vital to grasp how these trends will shape the landscape.
Adapting Pricing Models
As the world of machine learning expands, AWS may find it necessary to adapt its pricing structures. The emergence of new technologies, like automation and predictive analytics, could prompt AWS to re-evaluate how they price services. For instance, the integration of machine learning operations (MLOps) might lead to bundled pricing models which could reduce costs but require careful scrutiny.
Businesses must remain vigilant to these shifts. Keeping tabs on AWS announcements and roadmaps will be important, as they may hint at upcoming pricing changes. This can help organizations remain agile, avoiding any unpleasant surprises that could arise from sudden increases in costs or alterations in service packages.
Impact of Market Trends
Market trends hold significant sway over AWS SageMaker pricing. For instance, the growing competition among cloud service providers means that pricing strategies might continuously adjust to attract and retain customers. Companies such as Microsoft Azure and Google Cloud Platform could introduce new features or lower their prices, thereby forcing AWS to respond in kind.
Another trend impacting pricing revolves around the demand for AI and machine learning capabilities. As more businesses incorporate these technologies into their operations, the cost of services may adjust upward due to heightened demand. This can create a scenario where early adopters benefit from lower prices while latecomers face higher costs as the services become more sought after.
Staying ahead of market trends is crucial for businesses that rely on AWS SageMaker for their machine learning needs. Knowledge and foresight can translate into significant cost savings.
End
Understanding the pricing structure of AWS SageMaker is vital for anyone looking to harness the power of machine learning without breaking the bank. In the ever-evolving landscape of cloud computing, being able to navigate the costs associated with different services can provide a significant competitive edge. The pricing model is multifaceted, with numerous variables that come into play, from instance types to data processing fees.
Summary of Key Points
- Comprehensive Costs: AWS SageMaker's pricing covers a range of components, including instance pricing, data storage, and processing charges. Each of these aspects contributes to the total expenditure, making it important to assess your specific usage needs.
- Variations in Services: Different services within AWS SageMaker come with their own pricing structures. Understanding how these variations impact your budget helps in optimizing the use of resources. For instance, distinguishing between on-demand and spot instances can lead to significant savings.
- Projected Future Trends: As the market in cloud services shifts, so too does the pricing model of platforms like AWS SageMaker. Being aware of potential market trends and adjustments in pricing can aid in better budgeting for future projects.
Final Considerations
In summary, grasping AWS SageMaker pricing not only aids in making informed financial decisions but also empowers businesses and professionals to drive innovation through machine learning.
Consider the following when assessing your use of AWS SageMaker:
- Tailored Solutions: Each project may require a different approach to budgeting. Customizing your instance types and understanding your data processing needs is critical.
- Continuous Review: Regularly reviewing costs, alongside evaluating usage metrics, can unearth opportunities for optimization.
- Leveraging Tools: Utilize AWS's tools for cost estimation to create a realistic financial roadmap before embarking on extensive projects.
The understanding of these pricing dynamics not only helps in fiscal planning but also aligns your machine learning initiatives with strategic business objectives. Educating yourself on these elements is a fundamental step toward leveraging AWS SageMaker effectively.