Mastering Netflow Data Analysis: A Comprehensive Expert Guide
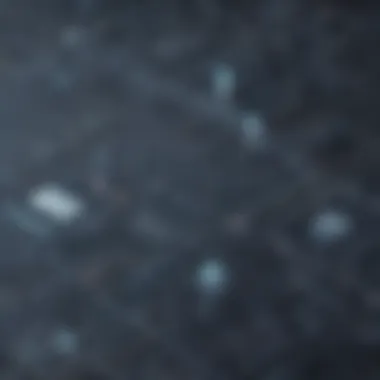
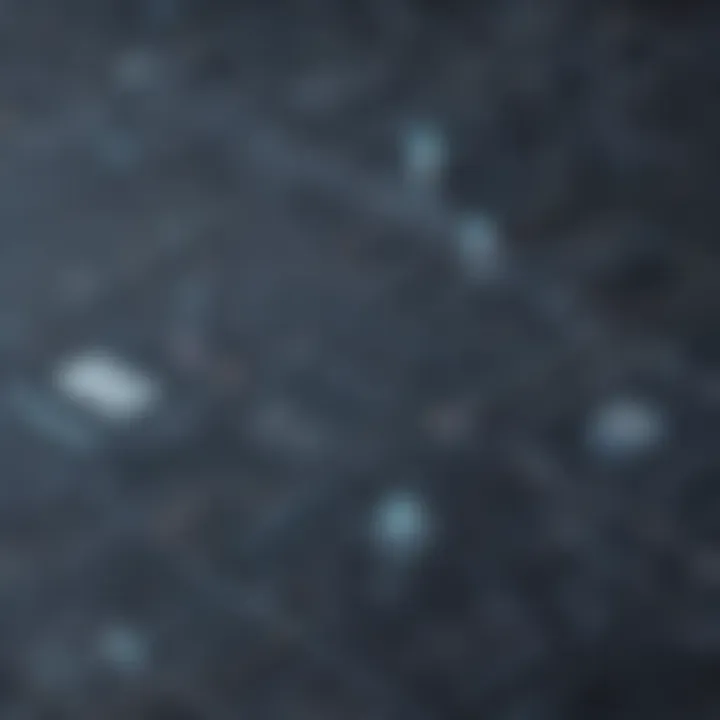
Software Features and Functionalities
Netflow Analyzer
The Netflow Analyzer software provides a range of features and functionalities to facilitate the analysis of network traffic data. It offers real-time monitoring, traffic visualization, and anomaly detection capabilities. Users can generate customized reports and alerts to gain insights into network behavior and potential security threats. The software's dashboard provides a user-friendly interface for easy navigation and data interpretation.
Netflow Collector
The Netflow Collector component gathers flow data from routers and switches and stores it for analysis. It categorizes the information based on different parameters such as source, destination, and protocols. The Netflow Collector enables users to aggregate and filter data, allowing for in-depth analysis of network traffic patterns and trends.
Netflow Traffic Analyzer
The Netflow Traffic Analyzer module processes the collected data and generates valuable insights for network administrators. It identifies bandwidth usage, application performance, and potential bottlenecks in the network infrastructure. By leveraging advanced algorithms, the Traffic Analyzer segment provides accurate traffic analysis and helps optimize network performance.
Pricing and Licensing Options
Subscription Model
The Netflow Data Analysis software offers a subscription-based pricing model, allowing users to pay for the service on a monthly or annual basis. The pricing tiers are structured based on the scale of network traffic to be analyzed and the features required. Users can choose a plan that suits their budget and operational needs.
Licensing Flexibility
Organizations can opt for flexible licensing options to accommodate dynamic network environments. The software allows for scalability, enabling users to upgrade or downgrade their licenses as per evolving requirements. This flexibility ensures cost efficiency and scalability in aligning the software with the organization's growth.
Supported Platforms and Compatibility
System Requirements
The Netflow Data Analysis software is compatible with major operating systems such as Windows, Linux, and macOS. It can be deployed on physical servers or virtual machines to adapt to diverse IT infrastructures. The software is designed to integrate seamlessly with popular network devices and protocols, ensuring compatibility across a wide range of network environments.
Third-Party Integration
To enhance usability and functionality, the software supports integration with third-party tools and applications. Users can synchronize the Netflow Analysis software with SIEM platforms, security tools, and network management systems for centralized monitoring and analysis. This compatibility simplifies data aggregation and correlation, streamlining the network analysis process.
Introduction to Netflow Data Analysis
An essential aspect of modern networking, Introduction to Netflow Data Analysis is crucial for understanding network traffic patterns and optimizing performance. In this comprehensive guide, we will delve into the intricacies of Netflow data, exploring its significance in extracting valuable insights from network activities. By highlighting key concepts and methodologies, this section sets the foundation for a deeper exploration of Netflow analysis tools and techniques.
Understanding Netflow Data
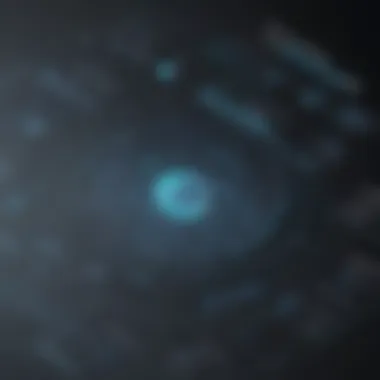
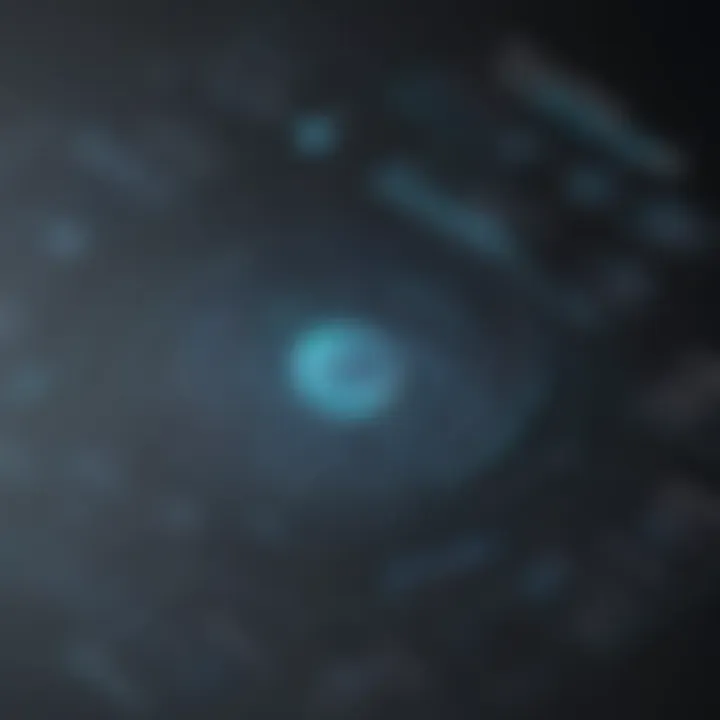
The concept of Netflow
The concept of Netflow signifies the fundamental method for collecting IP network traffic information, enabling detailed analysis of data flow within a network. By capturing network data at strategic points, Netflow facilitates the monitoring and understanding of traffic patterns, aiding in network troubleshooting and performance optimization. Its core characteristic lies in the ability to provide granular visibility into traffic behavior, making it a popular choice for network administrators and analysts alike. The unique feature of Netflow is its capacity to reveal network nuances that might otherwise go unnoticed, offering a holistic view of network operations.
Importance of Netflow data analysis
Effective analysis of Netflow data is paramount for enhancing network security, optimizing resource utilization, and identifying potential bottlenecks in network infrastructure. By extracting actionable insights from Netflow data, organizations can proactively address security threats, streamline network operations, and improve overall performance. The significance of Netflow data analysis lies in its ability to convert raw network data into actionable intelligence, empowering stakeholders to make informed decisions based on quantitative evidence. While the advantages of Netflow data analysis are undeniable, challenges such as data volume management and privacy concerns must be navigated to leverage its full potential.
Tools for Netflow Data Analysis
Popular tools for Netflow analysis
An array of tools exists for Netflow analysis, each offering unique features and functionalities to cater to diverse analytical needs. From open-source solutions to commercial platforms, popular tools like PRTG Network Monitor, SolarWinds NetFlow Traffic Analyzer, and Cisco NetFlow provide comprehensive capabilities for analyzing Netflow data. These tools enhance data visibility, facilitate trend analysis, and empower users to make data-driven decisions. Their popularity stems from the seamless integration of network monitoring features, robust reporting mechanisms, and user-friendly interfaces, making them indispensable for efficient Netflow analysis.
Features and capabilities of Netflow analysis tools
Netflow analysis tools boast a myriad of features, including real-time traffic monitoring, historical data analysis, and customizable dashboards. These tools offer advanced capabilities such as anomaly detection, traffic segmentation, and traffic pattern recognition, enabling deep insights into network behavior. Users can leverage these features to identify performance issues, detect security breaches, and optimize network configurations. Additionally, the visualization tools integrated into these platforms enhance data interpretation, making complex data sets easily comprehensible for network administrators and security professionals.
Challenges in Netflow Data Analysis
Data volume and scalability issues
One of the primary challenges in Netflow data analysis is managing the sheer volume of data generated by network devices. As network traffic continues to grow exponentially, organizations face difficulties in storing, processing, and analyzing large quantities of Netflow data. Scalability becomes a critical factor in ensuring efficient Netflow analysis, requiring robust infrastructure and optimized data handling techniques. Organizations must devise scalable data management strategies to address this challenge and prevent data overload from impeding effective analysis.
Security and privacy concerns
Another significant challenge in Netflow data analysis pertains to security and privacy considerations. Collecting and analyzing Netflow data raises privacy concerns regarding the monitoring of user activities and the protection of sensitive information. Moreover, ensuring the secure handling of Netflow data to prevent unauthorized access and data breaches is paramount. Organizations must implement stringent data protection measures, encryption protocols, and access controls to mitigate security risks associated with Netflow data analysis.
Techniques for Analyzing Netflow Data
In this section of the article, we delve into the essential topic of Techniques for Analyzing Netflow Data, a pivotal aspect in the realm of network traffic analysis. Understanding the nuances of these techniques is crucial for extracting valuable insights to enhance network security and performance. By incorporating advanced methodologies and tools, organizations can glean actionable information from their Netflow data, driving informed decision-making and proactive threat detection. Emphasizing the significance of a structured approach to Netflow data analysis, this section sheds light on the key elements, benefits, and considerations associated with leveraging various techniques to unravel the complexities of network traffic.
Data Preprocessing
Data cleaning and normalization
Data cleaning and normalization play a fundamental role in refining raw Netflow data for subsequent analysis. The process involves identifying and rectifying discrepancies, errors, and inconsistencies within the dataset, ensuring data integrity and accuracy. By standardizing data formats and eliminating outliers, data cleaning and normalization facilitate a uniform framework for further statistical analysis and visualization. This meticulous procedure enhances the quality and reliability of insights derived from Netflow data, enabling enterprises to make well-informed decisions based on a foundation of clean, consistent data.
Handling missing values
Addressing missing values is a vital aspect of data preprocessing to prevent erroneous conclusions during analysis. By employing strategies such as mean imputation or interpolation, organizations can mitigate the impact of missing data on the overall analysis. Understanding the context in which data is missing and applying appropriate techniques to handle these gaps is crucial for maintaining the integrity and relevance of the analysis results. Despite potential challenges posed by missing values, proactive management ensures the completeness and accuracy of Netflow data interpretation, laying a robust groundwork for subsequent analytical processes.
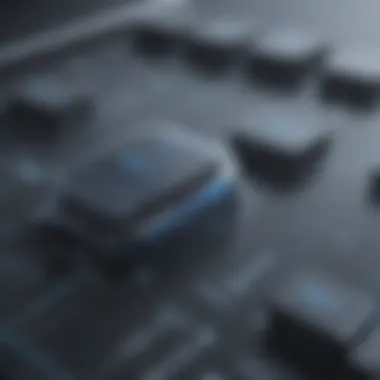
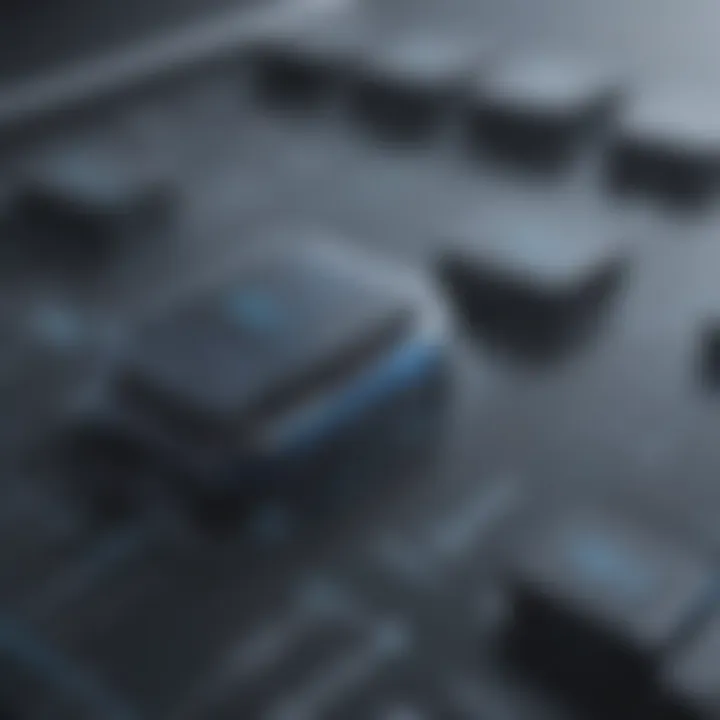
Statistical Analysis
Descriptive statistics
Descriptive statistics offer a comprehensive summary of Netflow data characteristics, including measures of central tendency, dispersion, and distribution. By presenting key metrics such as mean, median, and standard deviation, descriptive statistics provide valuable insights into the underlying patterns and trends within the data. These statistical measures serve as foundational elements for understanding the behavior of network traffic, aiding in the identification of anomalies and performance benchmarks. Leveraging descriptive statistics empowers organizations to quantify and describe the essential attributes of Netflow data, facilitating informed decision-making and strategic planning.
Inferential statistics
Inferential statistics extend beyond descriptive analysis to draw inferences and make predictions based on sample data. By utilizing hypothesis testing and regression analysis, inferential statistics enable organizations to extrapolate insights from limited data sets to make broader judgments about network behavior. These advanced statistical techniques facilitate the interpretation of Netflow data in a probabilistic framework, assisting in uncovering hidden patterns and relationships. Incorporating inferential statistics into Netflow data analysis enhances predictive capabilities and strengthens the foundation for deriving actionable intelligence from complex network datasets.
Data Visualization
Types of visualizations in Netflow analysis
Visual representations of Netflow data offer a powerful means of conveying complex information in a digestible format. From flow diagrams to heat maps, a diverse range of visualization techniques can provide intuitive insights into network activity and patterns. By choosing appropriate visualization methods based on the nature of the data, organizations can enhance data comprehension and decision-making processes. The visual representation of Netflow data not only simplifies the communication of insights but also uncovers hidden correlations and trends, fostering a deeper understanding of network dynamics.
Interpreting visual data
Interpreting visual data requires a keen eye for detail and an analytical mindset to extract meaningful interpretations from graphical representations. By scrutinizing trends, outliers, and anomalies in visualizations, analysts can discern actionable insights about network performance and security. The ability to interpret visual data accurately empowers organizations to identify emerging threats, monitor network efficiency, and optimize resource allocation. Through effective visualization interpretation, stakeholders can gain valuable perspectives on network operations, facilitating informed decision-making to address critical issues and opportunities.
Advanced Approaches to Netflow Data Analysis
In this intricate exploration of Netflow data analysis, the section on Advanced Approaches is particularly pivotal in unraveling the complexities of network traffic examination. By delving into the realms of Machine Learning, Anomaly Detection, and Real-time Data Analysis, this section sheds light on cutting-edge methodologies to enhance insights into network behavior and security measures. Through incorporating advanced approaches, organizations can glean hidden patterns, predict potential threats, and react swiftly to security breaches. Embracing innovative techniques not only amplifies the efficiency of data analysis but also fortifies network defenses against evolving cyber threats.
Machine Learning in Netflow Analysis
Applying machine learning algorithms
- Unraveling the intricate tapestry of Netflow data analysis, the application of machine learning algorithms stands out as a beacon of intelligence and precision. By leveraging algorithms to detect patterns, anomalies, and trends within network traffic, organizations can enhance their predictive capabilities, preempt security breaches, and optimize network performance. The automated nature of machine learning algorithms expedites the analysis process, allowing for real-time threat mitigation and proactive security measures. Although beneficial, biases in algorithm training data and the demand for computational resources pose challenges in deploying machine learning effectively in Netflow analysis.
Predictive analytics in network security
- Predictive analytics revolutionizes network security by forecasting potential threats, identifying vulnerabilities, and orchestrating preemptive security measures. By analyzing historical Netflow data and discerning patterns indicative of security risks, predictive analytics empowers organizations to stay one step ahead of cyber threats. The prescriptive insights derived from predictive analytics assist security teams in fortifying network defenses, allocating resources efficiently, and orchestrating timely responses to emerging threats. However, the reliance on historical data and the necessity of continuous model refinement underscore the complexities of integrating predictive analytics seamlessly into Netflow data analysis.
Anomaly Detection
Identifying network anomalies
- Anomaly detection serves as a cornerstone in the realm of Netflow data analysis, facilitating the identification of irregular patterns, unauthorized access attempts, and potential security breaches. By deploying sophisticated algorithms to detect deviations from normal network behavior, organizations can swiftly pinpoint anomalies, investigate security incidents, and bolster network resilience. The instantaneous nature of anomaly detection enables real-time threat mitigation and preemptive security measures, safeguarding organizational assets from malicious intrusions. Despite its efficacy, the challenge lies in distinguishing genuine anomalies from false positives, necessitating meticulous fine-tuning of detection thresholds and algorithms.
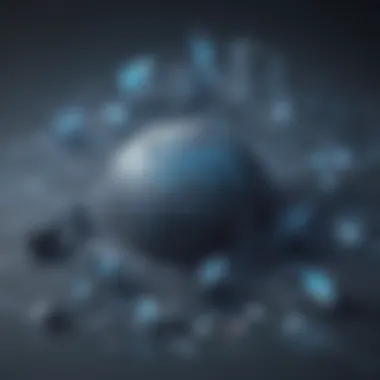
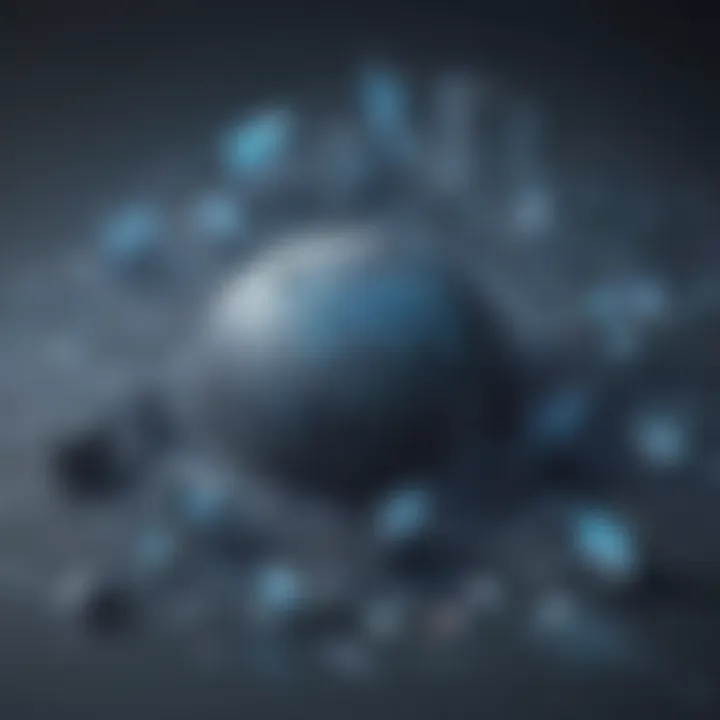
Mitigating security threats
- Mitigating security threats through proactive anomaly detection strategies is imperative in ensuring network integrity and data confidentiality. By swiftly identifying aberrant behaviors, security teams can enact targeted responses, isolate compromised systems, and prevent potential data breaches. Granular insights provided by anomaly detection methodologies empower organizations to fortify their security posture, adapt to evolving threats, and fortify network resilience. However, the volume of false alarms, resource-intensive monitoring requirements, and the need for continuous algorithm refinement pose challenges in implementing effective security threat mitigation strategies.
Real-time Data Analysis
Importance of real-time analytics
- Real-time data analysis emerges as a linchpin in Netflow data analysis, enabling organizations to monitor network traffic dynamically, detect anomalies promptly, and respond swiftly to security incidents. By harnessing the power of real-time analytics, organizations can enhance their situational awareness, fortify network defenses, and orchestrate rapid incident responses. The instantaneous nature of real-time analytics empowers security teams to detect emerging threats, neutralize security vulnerabilities, and ensure uninterrupted network operations. Nevertheless, the demand for high-performance computational infrastructure, real-time data processing capabilities, and real-time decision-making mechanisms present challenges in adopting real-time analytics seamlessly.
Tools for real-time Netflow analysis
- Equipping organizations with the requisite tools for real-time Netflow analysis is paramount in bolstering network security, optimizing resource allocation, and fortifying data insights. From visualization platforms to network monitoring dashboards, an array of tools facilitates real-time Netflow analysis, enabling organizations to identify suspicious activities, correlate security events, and delve deep into network traffic patterns. The integration of real-time analysis tools streamlines security incident response, enhances threat visibility, and augments network performance monitoring. Nevertheless, the complexity of tool integration, interoperability constraints, and the need for skilled personnel proficient in tool utilization underscore the challenges organizations face in leveraging tools effectively for real-time Netflow analysis.
Best Practices for Netflow Data Analysis
In the realm of network analysis, deploying best practices can significantly enhance the efficiency and effectiveness of netflow data evaluation. This section delves into the pivotal role of best practices in ensuring accurate and insightful data interpretation. By adhering to established standards and methodologies, organizations can streamline their analytical processes and derive meaningful insights from network traffic data. Emphasizing the importance of best practice implementation sets a solid foundation for robust data analysis strategies, ultimately leading to informed decision-making and optimized network performance.
Effective Data Interpretation
Developing data-driven insights
When it comes to developing data-driven insights, the focus is on leveraging empirical evidence to draw meaningful conclusions and make informed decisions. This approach involves harnessing the power of data analytics to extract actionable intelligence from netflow datasets. By employing statistical analysis and data visualization techniques, organizations can gain valuable insights into network behavior and performance trends. Developing data-driven insights allows for a data-centric approach to problem-solving and strategy formulation, enabling organizations to make strategic decisions based on concrete evidence.
Interpreting complex patterns
Interpreting complex patterns in netflow data involves analyzing intricate network behaviors and identifying underlying trends or anomalies. By deciphering complex data patterns, analysts can uncover hidden insights that may not be apparent at first glance. This process requires a deep understanding of network dynamics and the ability to discern meaningful patterns from noisy data. Interpreting complex patterns is essential for detecting irregularities in network traffic and preempting potential security threats. While challenging, this aspect of data interpretation plays a fundamental role in ensuring network reliability and data integrity.
Automation in Analysis
Utilizing automation tools
Automation tools play a crucial role in streamlining the data analysis process by automating repetitive tasks and reducing manual intervention. By leveraging automation tools, organizations can expedite data processing and analysis, leading to faster insights and more efficient decision-making. The use of automation tools also minimizes the risk of human error and ensures consistency in data processing. With the increasing volume of netflow data generated daily, automation tools offer a scalable solution for handling large datasets and extracting actionable intelligence effectively.
Streamlining analysis processes
Streamlining analysis processes involves optimizing the workflow of data analysis tasks to improve efficiency and accuracy. By identifying bottlenecks and implementing streamlined processes, organizations can enhance their analytical capabilities and accelerate insights generation. Streamlining analysis processes also involves integrating automation tools and data visualization techniques to facilitate smoother decision-making. This practice ensures that data analysis efforts are focused and yield meaningful results, ultimately maximizing the value derived from netflow data.
Continuous Monitoring
The importance of ongoing monitoring
Continuous monitoring of network traffic is essential for identifying anomalies, detecting security breaches, and maintaining network integrity. By implementing rigorous monitoring protocols, organizations can proactively safeguard their network infrastructure and respond swiftly to emerging threats. The importance of ongoing monitoring lies in its ability to provide real-time visibility into network operations, enabling rapid intervention in case of abnormalities. Continuous monitoring forms the cornerstone of effective network management and plays a critical role in ensuring operational efficiency and data security.
Alert mechanisms for network events
Alert mechanisms for network events are designed to notify administrators of potential security incidents or network irregularities. These systems issue alerts based on predefined criteria, such as unusual traffic patterns or unauthorized access attempts. By configuring alert mechanisms, organizations can stay vigilant against malicious activities and promptly address suspected security breaches. The proactive nature of alert mechanisms allows for timely responses to critical network events, minimizing potential downtime and data loss risks. Implementing robust alert mechanisms enhances network security posture and reinforces incident response capabilities, contributing to overall network resilience and stability.